Exploring the Utility of Anonymized EHR Datasets in Machine Learning Experiments in the Context of the MODELHealth Project
APPLIED SCIENCES-BASEL(2022)
Abstract
The object of this paper was the application of machine learning to a clinical dataset that was anonymized using the Mondrian algorithm. (1) Background: The preservation of patient privacy is a necessity rising from the increasing digitization of health data; however, the effect of data anonymization on the performance of machine learning models remains to be explored. (2) Methods: The original EHR derived dataset was subjected to anonymization by applying the Mondrian algorithm for various k values and quasi identifier (QI) set attributes. The logistic regression, decision trees, k-nearest neighbors, Gaussian naive Bayes and support vector machine models were applied to the different dataset versions. (3) Results: The classifiers demonstrated different degrees of resilience to the anonymization, with the decision tree and the KNN models showing remarkably stable performance, as opposed to the Gaussian naive Bayes model. The choice of the QI set attributes and the generalized information loss value played a more important role than the size of the QI set or the k value. (4) Conclusions: Data anonymization can reduce the performance of certain machine learning models, although the appropriate selection of classifier and parameter values can mitigate this effect.
MoreTranslated text
Key words
machine learning, anonymization, Mondrian
AI Read Science
Must-Reading Tree
Example
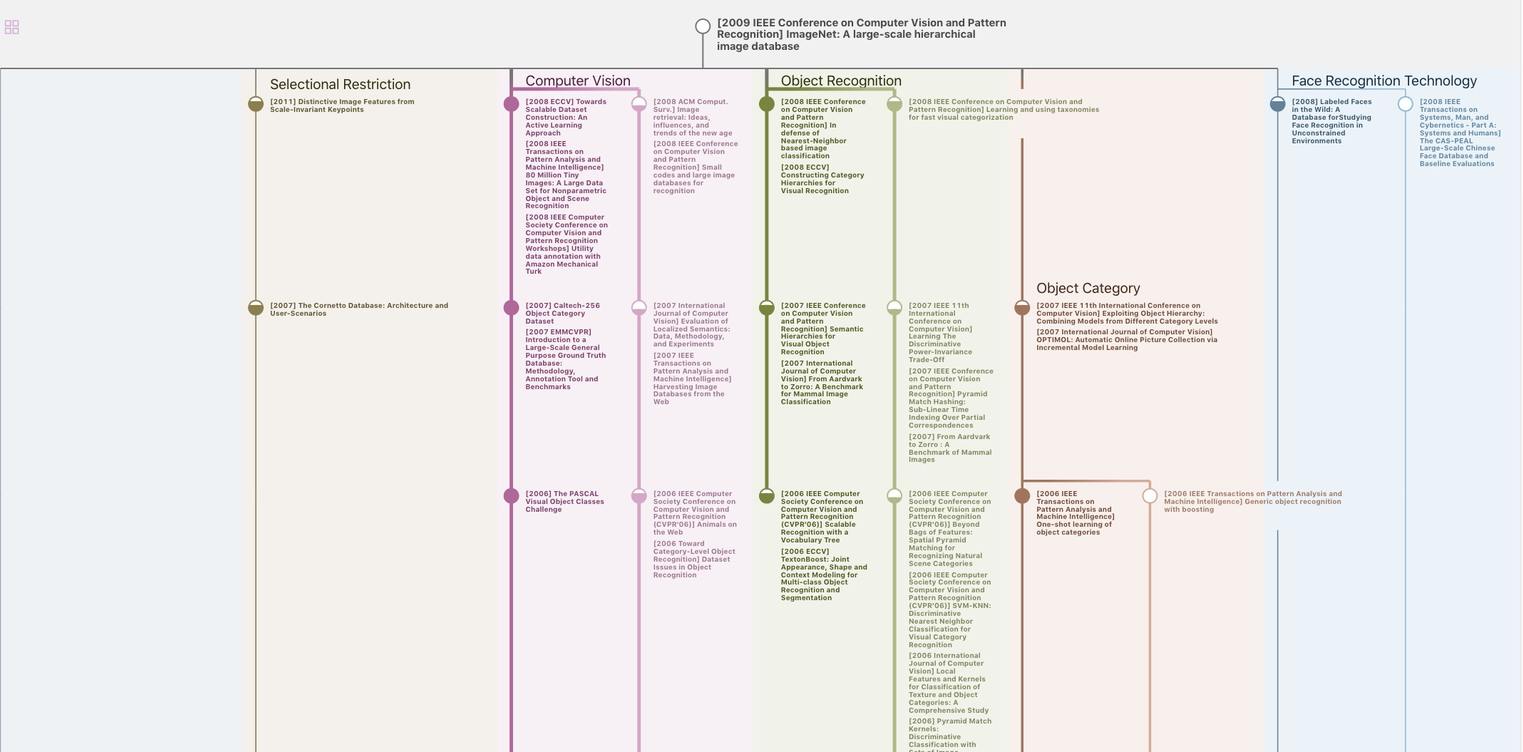
Generate MRT to find the research sequence of this paper
Chat Paper
Summary is being generated by the instructions you defined