SIHRNet: a fully convolutional network for single image highlight removal with a real-world dataset
JOURNAL OF ELECTRONIC IMAGING(2022)
Abstract
Specular highlight in images is detrimental to accuracy in object recognition tasks. The prior model-based methods for single image highlight removal (SHIR) are limited in images with large highlight regions or achromatic regions, and recent learning-based methods do not perform well due to lack of proper datasets for training either. A network for SHIR is proposed, which is trained with losses that utilize image intrinsic features and can reconstruct a smooth and natural specular-free image from a single input highlight image. Dichromatic reflection model is used to compute the pseudo specular-free image for providing complementary information for the network. A real-world dataset with highlight images and the corresponding ground-truth specular-free images is collected for network training and quantitative evaluation. The proposed network is validated by comprehensive quantitative experiments and outperforms state-of-the-art highlight removal approaches in structural similarity and peak signal-to-noise ratio. Experimental results also show that the network could improve the recognition performance in applications of computer vision. Our source code is available at https://github.com/coach-wang/SIHRNet. (C) 2022 SPIE and IS&T
MoreTranslated text
Key words
image processing, highlight removal, image enhancement, computer vision, neural network
AI Read Science
Must-Reading Tree
Example
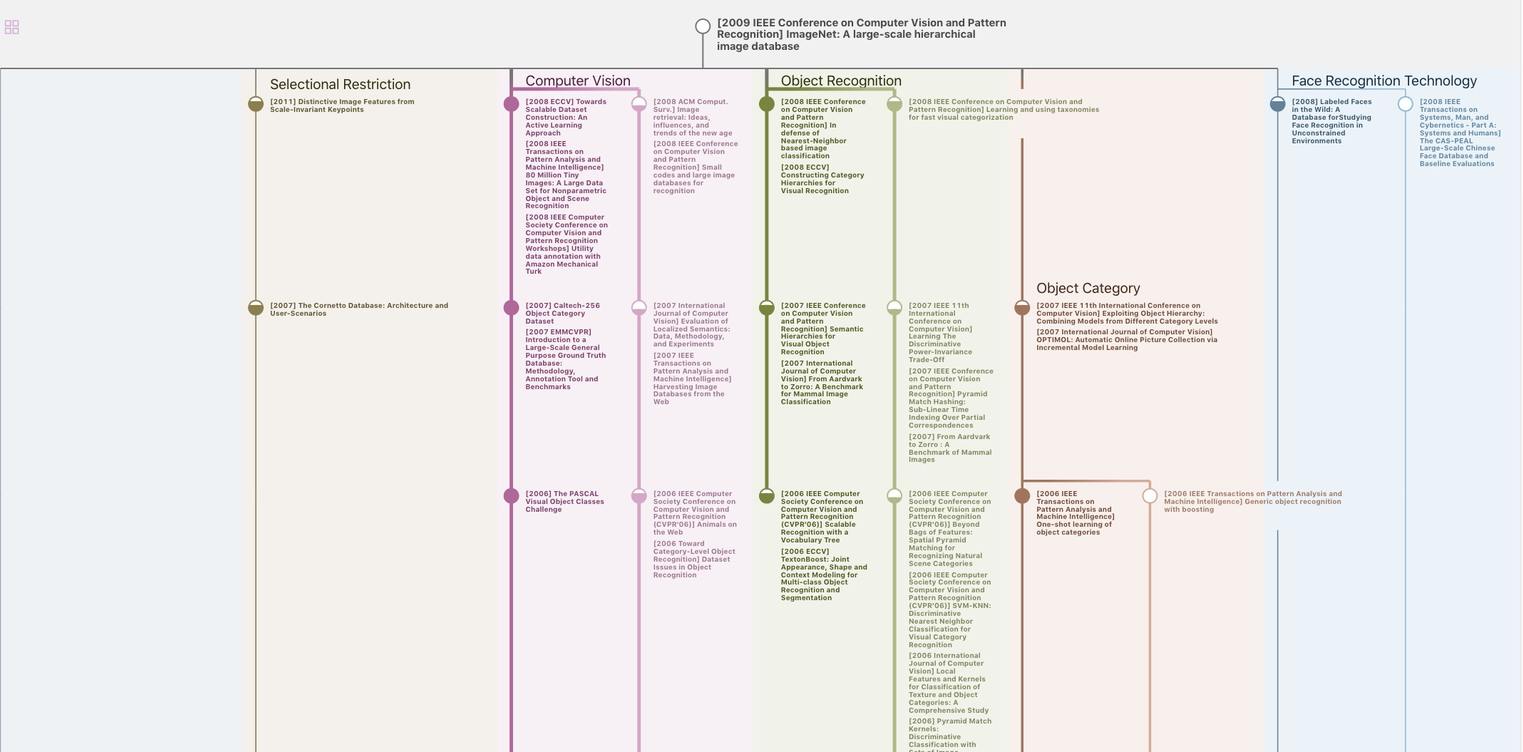
Generate MRT to find the research sequence of this paper
Chat Paper
Summary is being generated by the instructions you defined