Ground Pedestrian and Vehicle Detections Using Imaging Environment Perception Mechanisms and Deep Learning Networks
ELECTRONICS(2022)
Abstract
In order to build a robust network for the unmanned aerial vehicle (UAV)-based ground pedestrian and vehicle detection with a small number of training datasets but strong luminance environment adaptability, a system that considers both environment perception computation and a lightweight deep learning network is proposed. Because the visible light camera is sensitive to complex environmental lights, the following computational steps are designed: First, entropy-based imaging luminance descriptors are calculated; after image data are transformed from RGB to Lab color space, the mean-subtracted and contrast-normalized (MSCN) values are computed for each component in Lab color space, and then information entropies were estimated using MSCN values. Second, environment perception was performed. A support vector machine (SVM) was trained to classify the imaging luminance into excellent, ordinary, and severe luminance degrees. The inputs of SVM are information entropies; the output is the imaging luminance degree. Finally, six improved Yolov3-tiny networks were designed for robust ground pedestrian and vehicle detections. Extensive experiment results indicate that our mean average precisions (MAPs) of pedestrian and vehicle detections can be better than similar to 80% and similar to 94%, respectively, which overmatch the corresponding results of ordinary Yolov3-tiny and some other deep learning networks.
MoreTranslated text
Key words
ground pedestrian detection, ground vehicle detection, environment luminance perception, deep learning, smart city
AI Read Science
Must-Reading Tree
Example
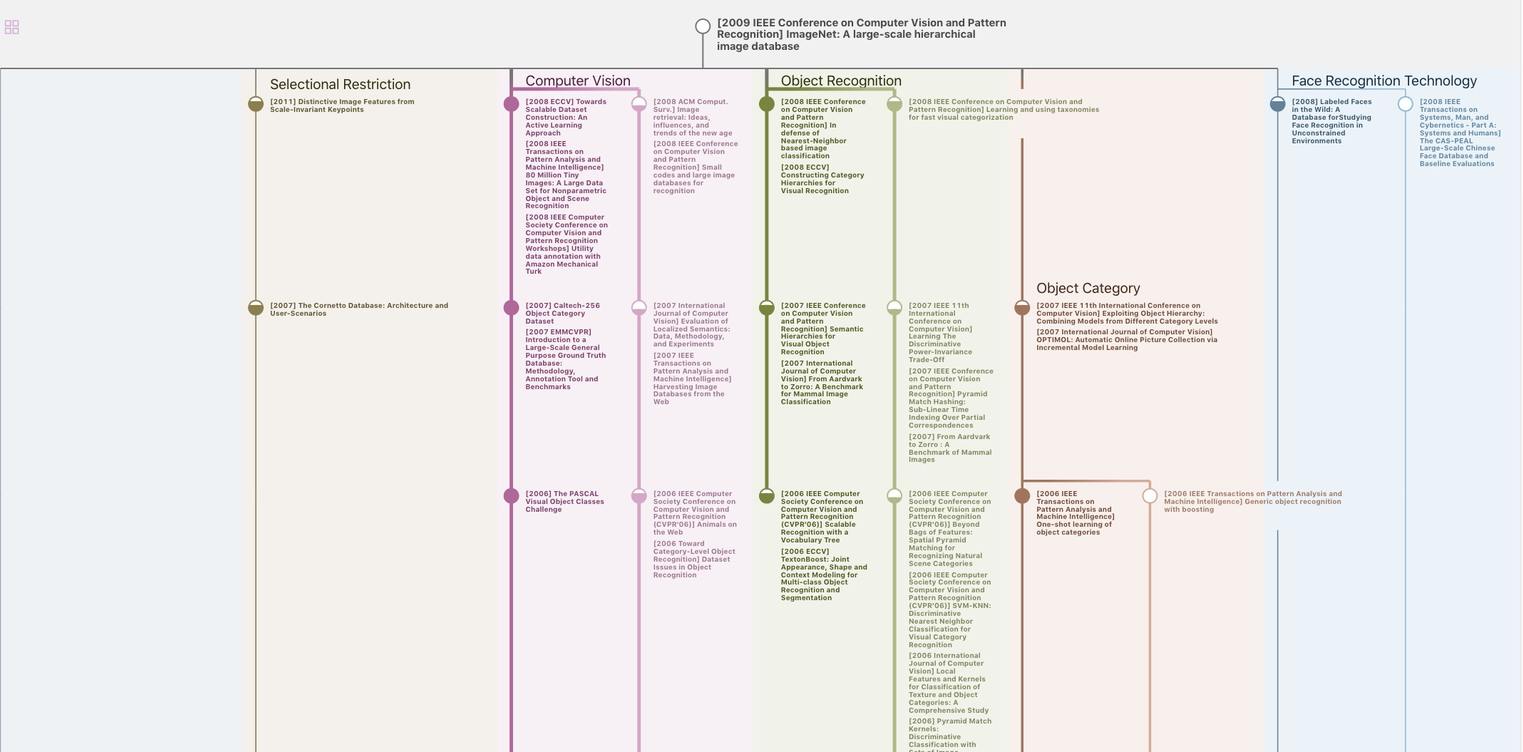
Generate MRT to find the research sequence of this paper
Chat Paper
Summary is being generated by the instructions you defined