Few-Shot Multi-Class Ship Detection in Remote Sensing Images Using Attention Feature Map and Multi-Relation Detector
REMOTE SENSING(2022)
摘要
Monitoring and identification of ships in remote sensing images is of great significance for port management, marine traffic, marine security, etc. However, due to small size and complex background, ship detection in remote sensing images is still a challenging task. Currently, deep-learning-based detection models need a lot of data and manual annotation, while training data containing ships in remote sensing images may be in limited quantities. To solve this problem, in this paper, we propose a few-shot multi-class ship detection algorithm with attention feature map and multi-relation detector (AFMR) for remote sensing images. We use the basic framework of You Only Look Once (YOLO), and use the attention feature map module to enhance the features of the target. In addition, the multi-relation head module is also used to optimize the detection head of YOLO. Extensive experiments on publicly available HRSC2016 dataset and self-constructed REMEX-FSSD dataset validate that our method achieves a good detection performance.
更多查看译文
关键词
few-shot learning, multi-class ship detection, deep learning, remote sensing images
AI 理解论文
溯源树
样例
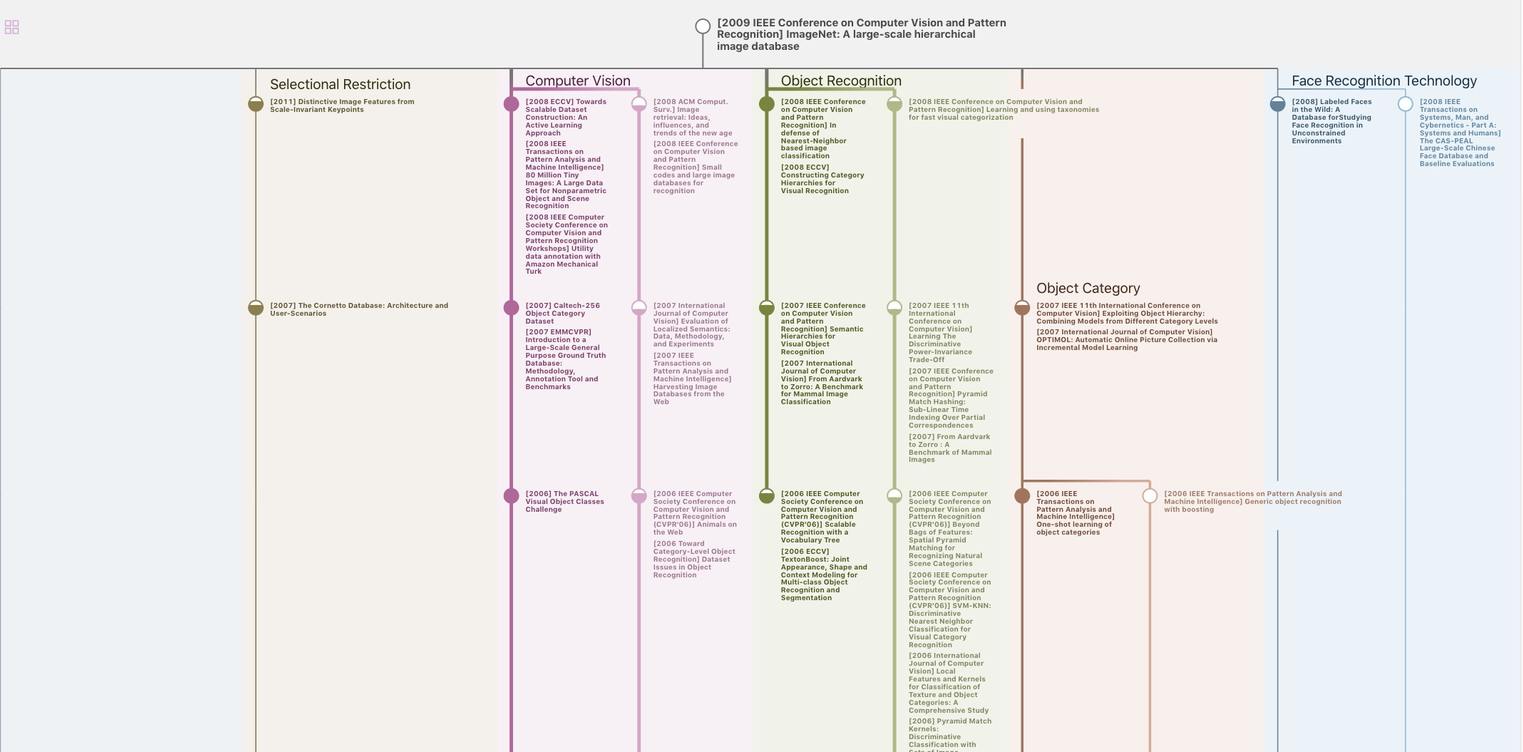
生成溯源树,研究论文发展脉络
Chat Paper
正在生成论文摘要