Using random forests to predict passengers' thermal comfort in underground train carriages
INDOOR AND BUILT ENVIRONMENT(2023)
摘要
This research developed an intelligent ensemble machine learning prediction model for the thermal comfort of passengers inside the compartment of the subway. Data sources used for data-driven modelling were obtained from on-site measurements and passengers' questionnaires in the compartments of the Nanjing subway. The four models were established using methodologies of Logistic Regression (LR), Random Forest (RF), Support Vector Machine (SVM) and Decision Tree (DT) in machine learning, respectively. The performance of the RF method was compared with DT, LR and SVM in terms of conventional statistical metrics, namely, Mean Squared Error (MSE), Root Mean Square Error (RMSE) and Correlation Coefficients squares (R-2). Thermal Sensation Vote with the seven-level indicator (TSV-7) and Thermal Sensation Vote with the three-level indicator (TSV-3) were employed to obtain passengers' thermal comfort and evaluate the models' predictions. In this study, the R-2 value of the RF model is 0.6527 and 0.6607 for TSV-7 and TSV-3, which shows higher accuracy than DT, LR and SVM models in predicting the two kinds of Thermal Sensation Vote (TSV). The results show that the predictive performance of the proposed RF model is outstanding, and it can predict the TSV value of passengers inside the compartment of the subway more efficiently.
更多查看译文
关键词
Thermal sensation vote,data-driven modelling,machine learning,random forest
AI 理解论文
溯源树
样例
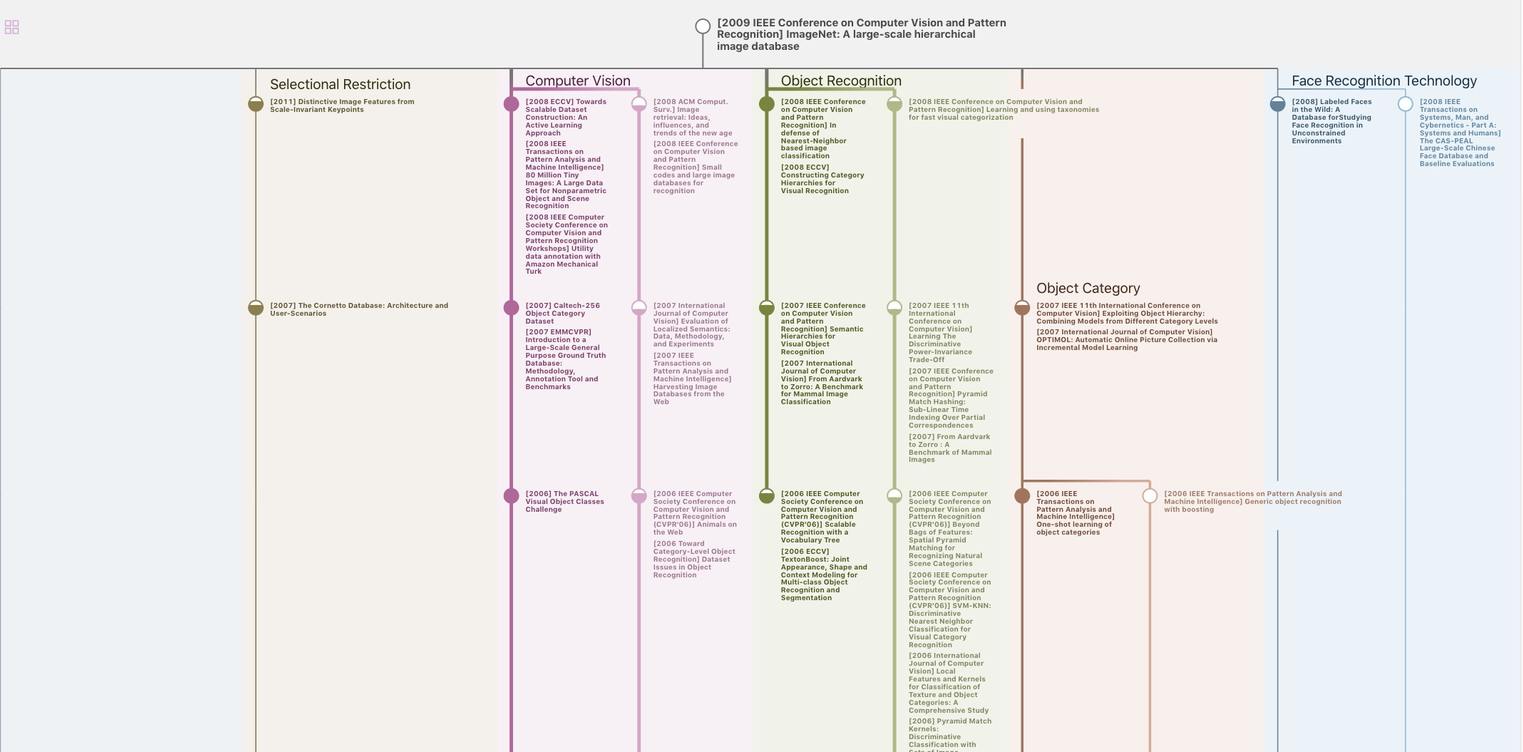
生成溯源树,研究论文发展脉络
Chat Paper
正在生成论文摘要