Groundwater Quality Modeling Using Geostatistical Methods and Artificial Neural Networks: a Case Study of the Western Middle Cheliff Alluvial Plain in Algeria
DESALINATION AND WATER TREATMENT(2022)
Key words
Middle Cheliff alluvial plain,Groundwater quality,Geostatistics,Artificial neural network,Prediction of water quality index,Kriging model
AI Read Science
Must-Reading Tree
Example
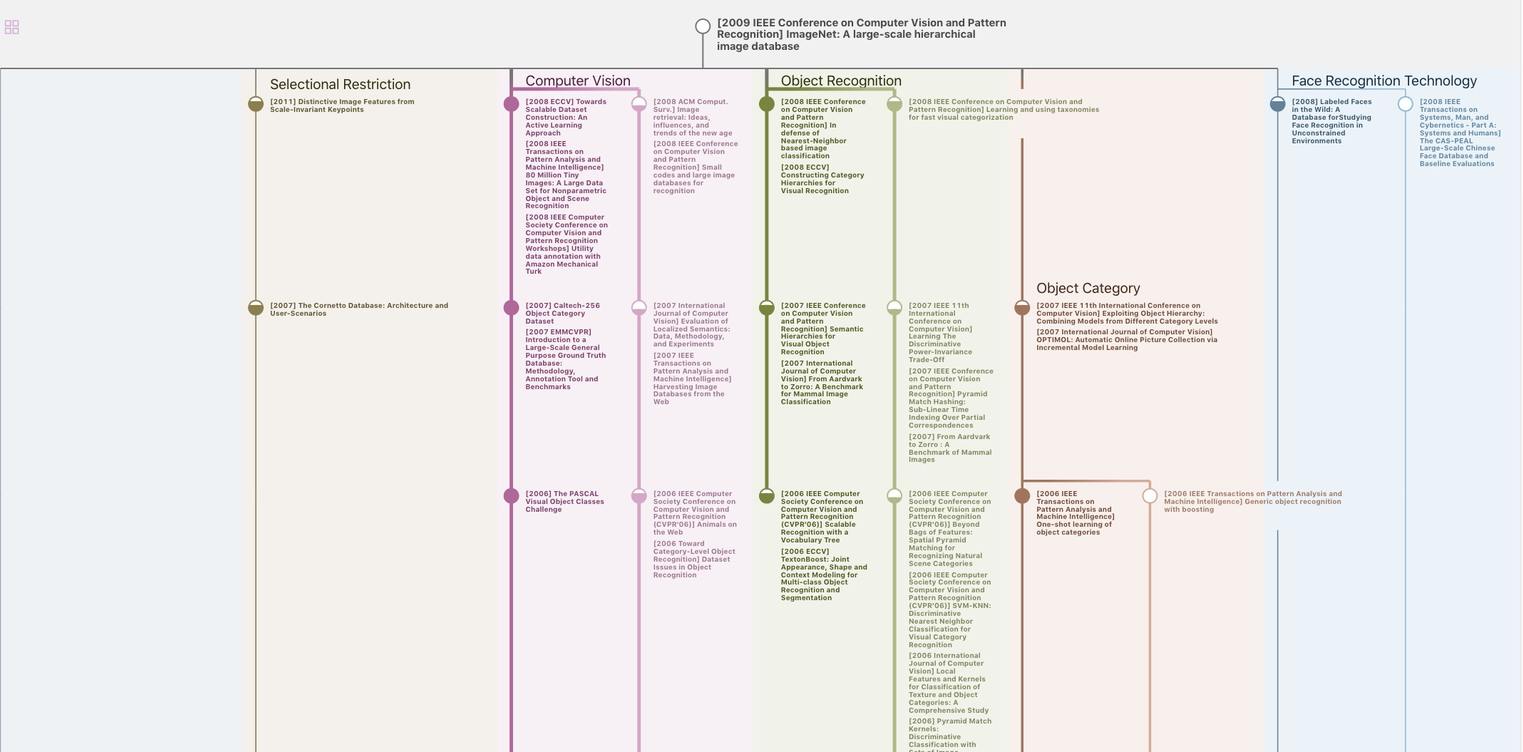
Generate MRT to find the research sequence of this paper
Chat Paper
Summary is being generated by the instructions you defined