Application of CNN and Long Short-Term Memory Network in Water Quality Predicting
INTELLIGENT AUTOMATION AND SOFT COMPUTING(2022)
摘要
Water resources are an indispensable precious resource for human sur-vival and development. Water quality prediction plays a vital role in protectingand enhancing water resources. Changes in water quality are influenced by manyfactors, both long-term and short-term. Therefore, according to water qualitychanges'periodic and nonlinear characteristics, this paper considered dissolvedoxygen as the research object and constructed a neural network model combiningconvolutional neural network (CNN) and long short-term memory network(LSTM) to predict dissolved oxygen index in water quality. Firstly, we prepro-cessed the water quality data set obtained from the water quality monitoring plat-form. Secondly, we used a CNN network to extract local features from thepreprocessed water quality data and transferred time series with better expressivepower than the original water quality information to the LSTM layer for predic-tion. We choose optimal parameters by setting the number of neurons in theLSTM network and the size and number of convolution kernels in the CNN net-work. Finally, LSTM and the proposed model were used to evaluate the waterquality data. Experiments showed that the proposed model is more accurate thanthe conventional LSTM in the prediction effect of peakfitting. Compared with theconventional LSTM model, its root mean square error, Pearson correlation coeffi-cient, mean absolute error and mean square error were respectively optimized by5.99%, 2.80%, 2.24%, and 11.63%.
更多查看译文
关键词
LSTM,CNN,dissolved oxygen,water quality predicting
AI 理解论文
溯源树
样例
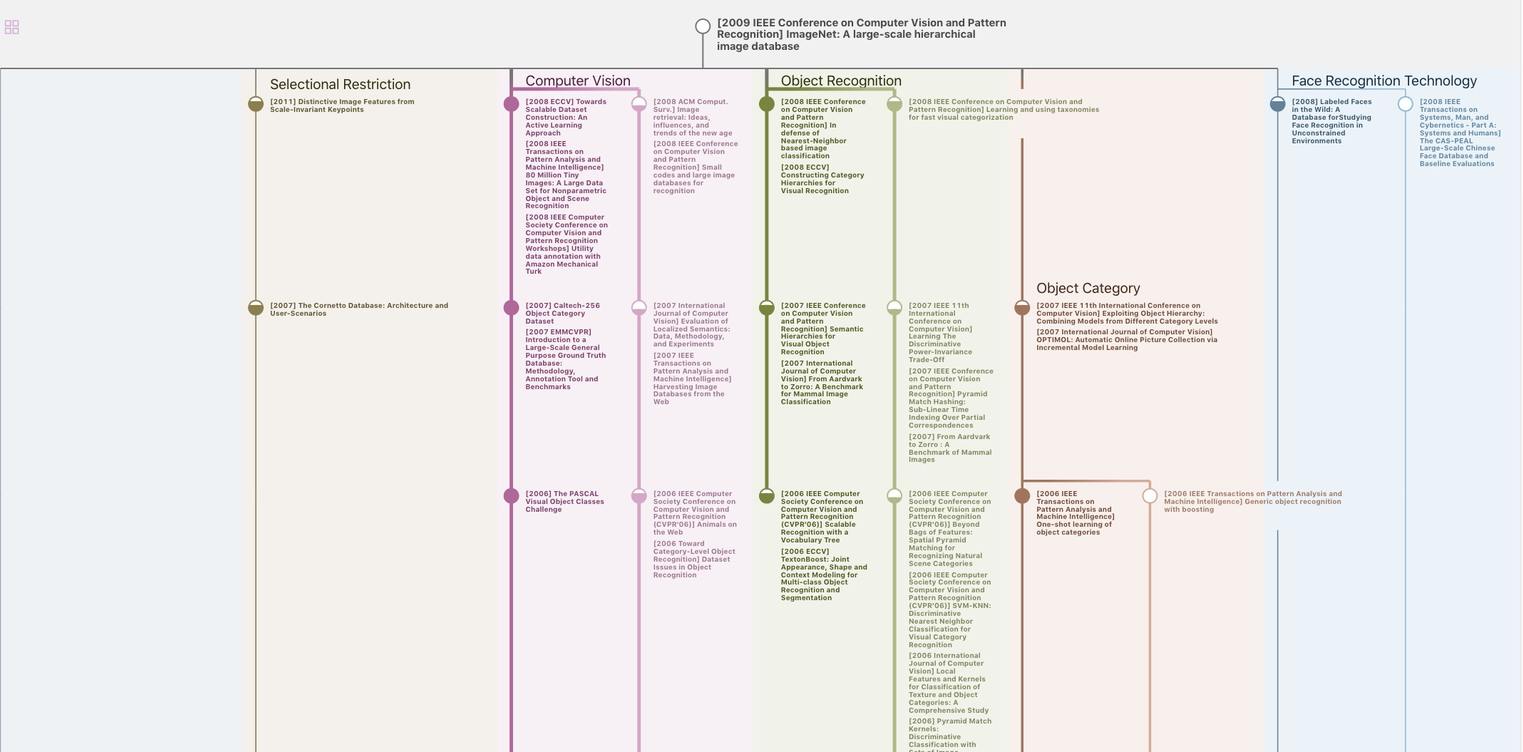
生成溯源树,研究论文发展脉络
Chat Paper
正在生成论文摘要