Weakly Supervised Anomaly Detection Based on Two-Step Cyclic Iterative PU Learning Strategy
NEURAL PROCESSING LETTERS(2022)
摘要
Weakly supervised anomaly detection for surveillance video is a highly challenging task due to inaccurate label information and sparse and diverse distribution of abnormal samples. In this paper, we define the videos containing only normal frames as the positive sample P set, and the abnormal videos lacking frame-level labels as the unlabeled sample U set, thus defining weakly supervised anomaly detection as a Positive and Unlabeled Learning (PUL) problem for the first time. Aiming at the characteristics of video anomaly detection task, this paper improved the traditional PUL strategy and proposed a two-step cyclic iterative PUL strategy. By connecting the sifting stage with the classification stage through the feedback loop, the detection performance of the network was improved through alternate iteration training. At the same time, we propose an anomaly detection framework oriented to local features, and use the feature vectors after feature decomposition to train the network. Through the validation experiments on three different datasets, the results show that the proposed detection model is superior to most competitive models, which proves the effectiveness of this method.
更多查看译文
关键词
Weakly supervised video anomaly detection,Positive and Unlabeled Learning,Two-step cyclic iterative algorithm,Feature decomposition
AI 理解论文
溯源树
样例
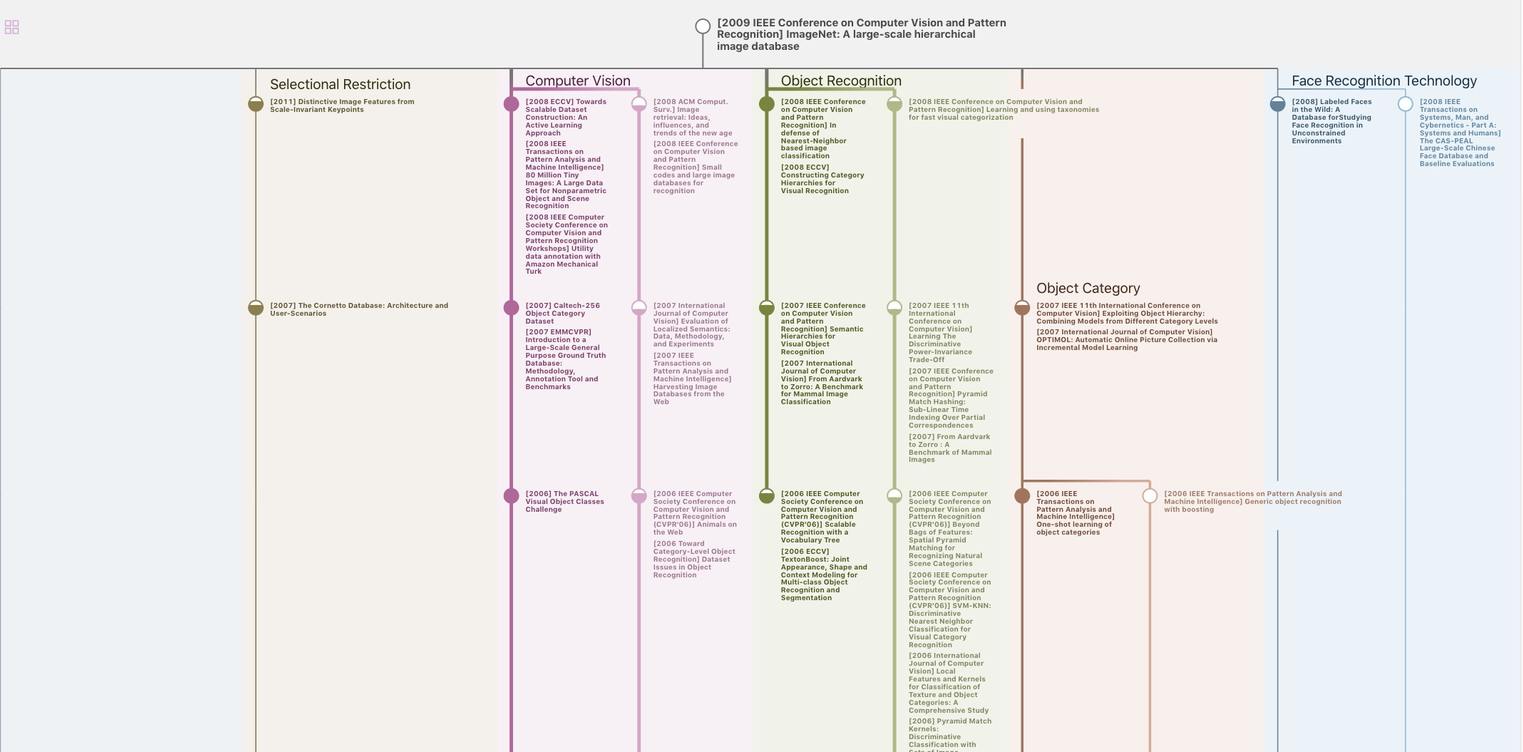
生成溯源树,研究论文发展脉络
Chat Paper
正在生成论文摘要