Semi-supervised multi-view clustering with dual hypergraph regularized partially shared non-negative matrix factorization
Science China Technological Sciences(2022)
Abstract
Real-world data can often be represented in multiple forms and views, and analyzing data from different perspectives allows for more comprehensive learning of the data, resulting in better data clustering results. Non-negative matrix factorization (NMF) is used to solve the clustering problem to extract uniform discriminative low-dimensional features from multi-view data. Many clustering methods based on graph regularization have been proposed and proven to be effective, but ordinary graphs only consider pairwise relationships between samples. In order to learn the higher-order relationships that exist in the sample manifold and feature manifold of multi-view data, we propose a new semi-supervised multi-view clustering method called dual hypergraph regularized partially shared non-negative matrix factorization (DHPS-NMF). The complex manifold structure of samples and features is learned by constructing samples and feature hypergraphs. To improve the discrimination power of the obtained low-dimensional features, semi-supervised regression terms are incorporated into the model to effectively use the label information when capturing the complex manifold structure of the data. Ultimately, we conduct experiments on six real data sets and the results show that our algorithm achieves encouraging results in comparison with some methods.
MoreTranslated text
Key words
multi-view clustering,semi-supervised learning,nonnegative matrix factorization (NMF),dual hypergraph
AI Read Science
Must-Reading Tree
Example
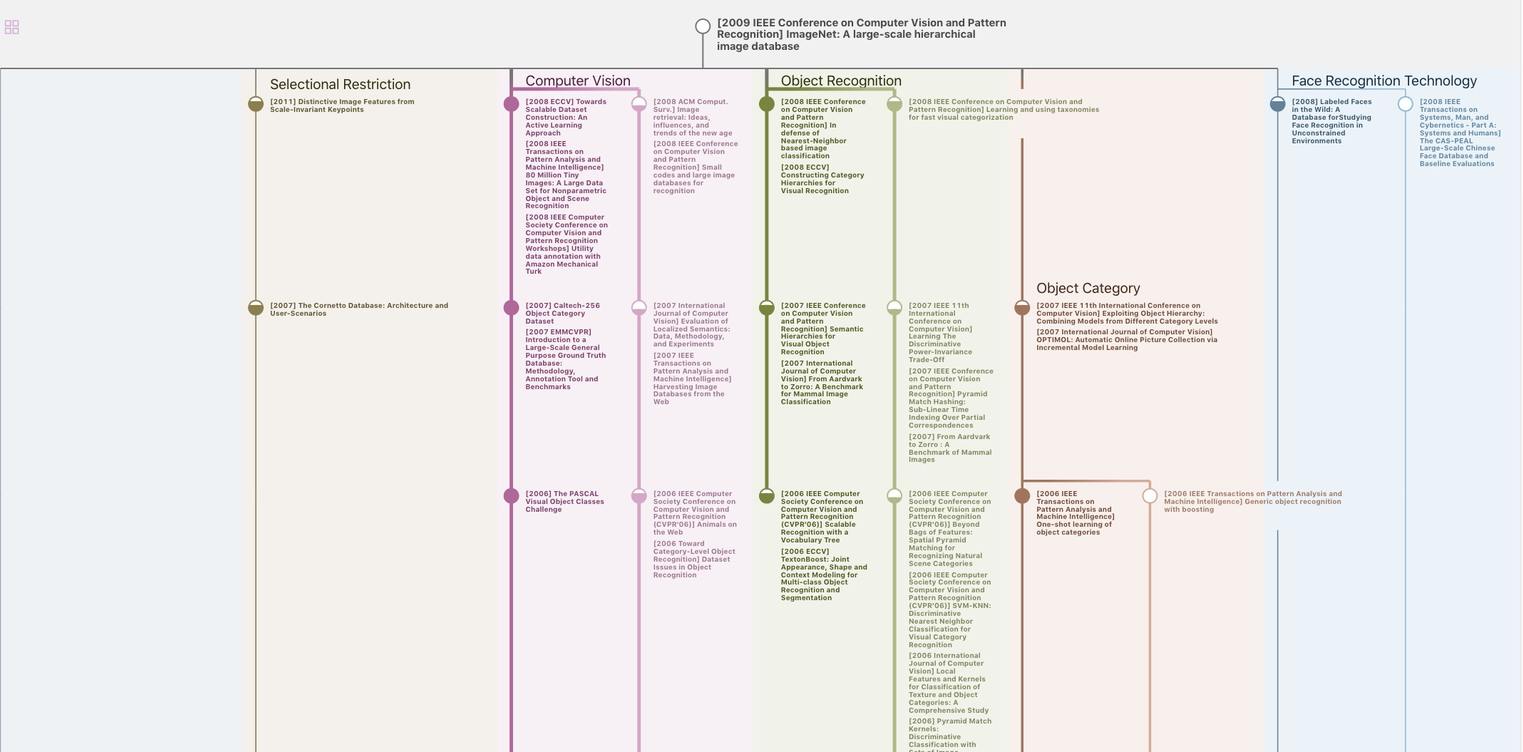
Generate MRT to find the research sequence of this paper
Chat Paper
Summary is being generated by the instructions you defined