Advanced multi-input system identification for next generation aircraft loads monitoring using linear regression, neural networks and deep learning
Mechanical Systems and Signal Processing(2022)
摘要
Over the past decade, the ideologies surrounding Structural Health Monitoring (SHM) have shifted drastically within the aerospace engineering disciplines, predominantly onus to rapid advancements in machine intelligence. While traditional SHM practices are based on scheduled and pre-emptive maintenance, the NextGen SHM system, known commonly as Prognostics and Health Management (PHM), has a focus on pro-active condition-based maintenance, forecasting and prognostics — a milestone on the trajectory towards Digital Twin technology. In aircraft, particularly defense fighter air platforms, fatigue-critical high-amplitude cyclic behavior is unavoidable, where rapid fatigue life consumption due to an airframe buffet is one of the most problematic phenomena that engineers have encountered throughout the 4th and 5th generation fighter programs. This paper serves as a point-of-reference consolidating a range of machine learning models, under a single benchmark aircraft Multi-Input Single-Output (MISO) loads monitoring problem. Linear regression models, traditional (shallow) artificial neural networks, and deep learning strategies are all explored, where strain sensors are used as inputs to predict representative bending and torsional dynamic (buffet) and quasi-static (maneuver) load spectra on an aircraft wing during transonic buffeting maneuvers. For the benchmark system considered herein, the MISO coherence ranges from high to very weak depending on the load case, hereby providing a unique opportunity to rigorously explore the time-series modeling requirements and make valuable recommendations across a wide range of data-qualities that are likely to be encountered in traditional or modern aircraft data-acquisition systems or, for that matter, in any mechanical systems plagued by fatigue.
更多查看译文
关键词
Structural Health Monitoring,MISO loads monitoring,Dynamic loads,Quasi-static loads,Artificial neural networks,Deep learning,Linear regression
AI 理解论文
溯源树
样例
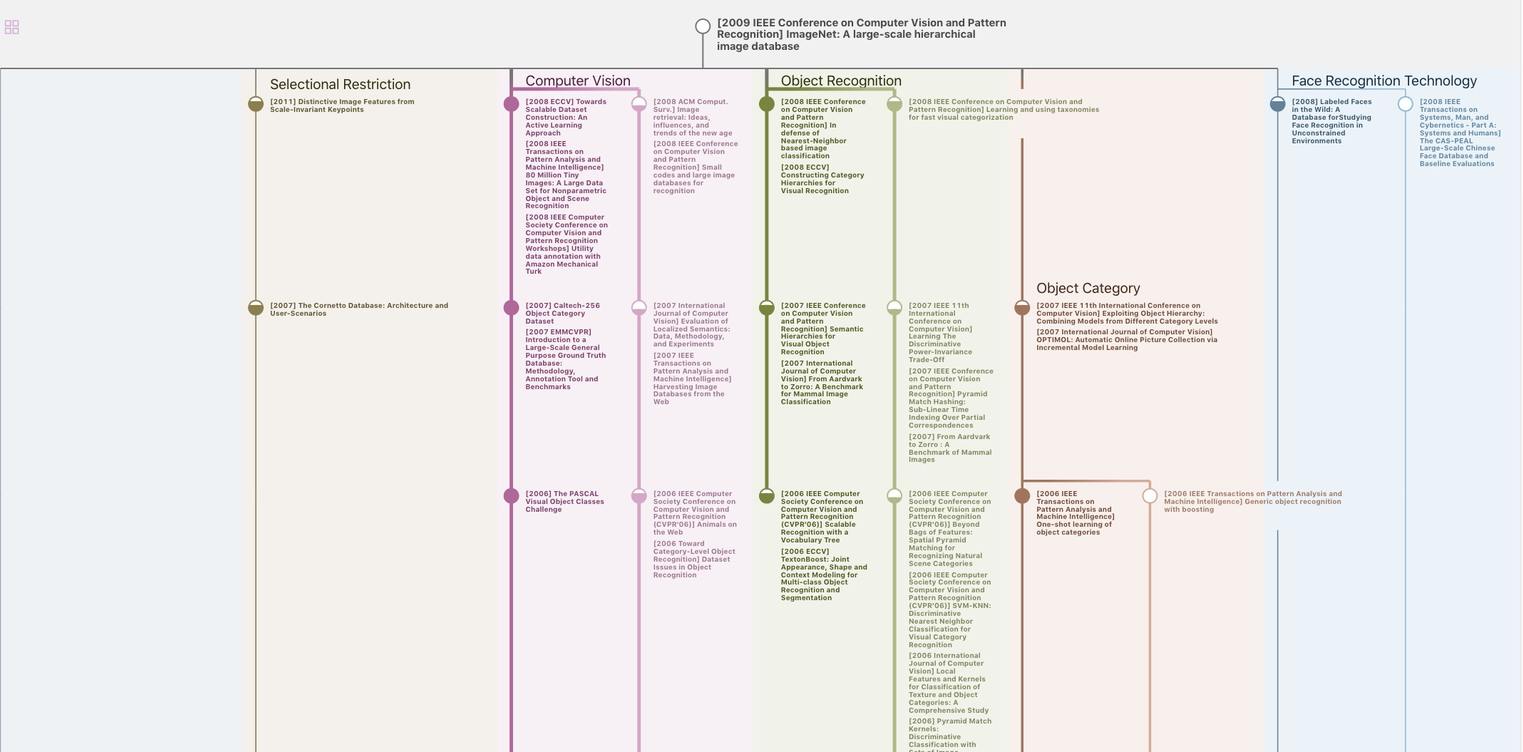
生成溯源树,研究论文发展脉络
Chat Paper
正在生成论文摘要