Heterogeneous Information Network Representation Learning Incorporating Community Structure
IEEE ACCESS(2022)
摘要
Network embedding usually learns the node representations using their local context information. However, as an important mesoscopic description of network structures, community structures hidden in the networks have been largely ignored. Incorporating community structures into network embedding could provide effective and a wealth of information to overcome the problem of sparse data in local structures, and make the learned node representation more identifiable. Inspired by this, we propose a representation learning model of community-augmented heterogeneous information network embedding (CAHE), which integrates community structure to preserve community structure information of various types of nodes. In detail, we assume that the different types of nodes exist in the different community structures for a heterogeneous information network, and define community embedding as a set of Mixture Gaussian models. Then, we unify the community embedding of all types of nodes to improve the capacity of network representation. A large of experimental results of several kinds of homogeneous and heterogeneous information network representation learning on two public datasets suggest that the proposed CAHE is superior to other several state-of-art methods.
更多查看译文
关键词
Representation learning, Complex networks, Task analysis, Semantics, Clustering algorithms, Transforms, Research and development, Network embedding, community-augmented, node embedding, community embedding, heterogeneous information network
AI 理解论文
溯源树
样例
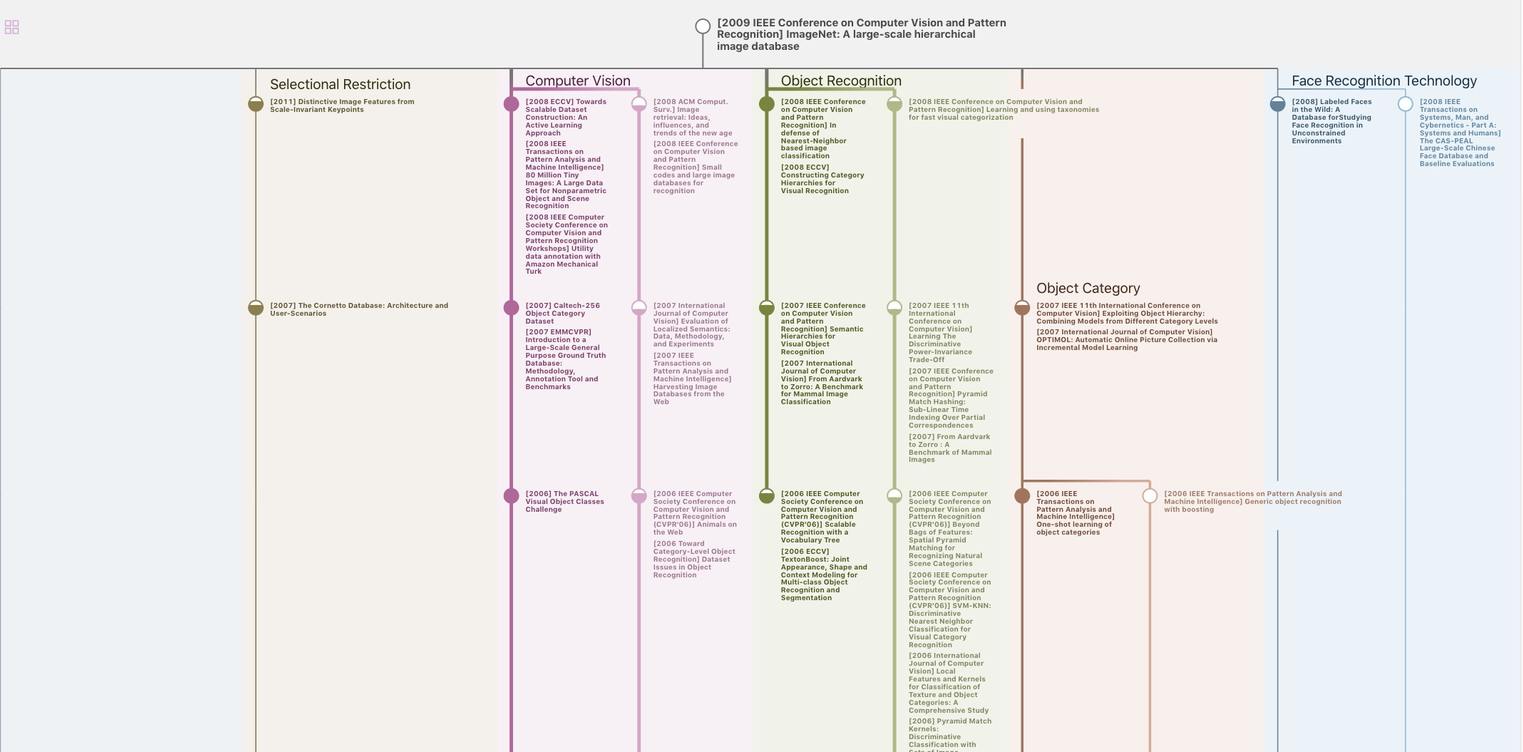
生成溯源树,研究论文发展脉络
Chat Paper
正在生成论文摘要