Efficient Load Frequency Control of Renewable Integrated Power System: A Twin Delayed DDPG-Based Deep Reinforcement Learning Approach
IEEE ACCESS(2022)
摘要
Power systems have been evolving dynamically due to the integration of renewable energy sources, making it more challenging for power grids to control the frequency and tie-line power variations. In this context, this paper proposes an efficient automatic load frequency control of hybrid power system based on deep reinforcement learning. By incorporating intermittent renewable energy sources, variable loads and electric vehicles, the complexity of the interconnected power system is escalated for a more realistic approach. The proposed method tunes the proportional-integral-derivative (PID) controller parameters using an improved twin delayed deep deterministic policy gradient (TD3) based reinforcement learning agent, where a non-negative fully connected layer is added with absolute function to avoid negative gain values. Multi deep reinforcement learning agents are trained to obtain the optimal controller gains for the given two-area interconnected system, and each agent uses the local area control error information to minimize the deviations in frequency and tie-line power. The integral absolute error of area control error is used as a reward function to derive the controller gains. The proposed approach is tested under random load-generation disturbances along with nonlinear generation behaviors. The simulation results demonstrate the superiority of the proposed approach compared to other techniques presented in the literature and show that it can effectively cope with nonlinearities caused by load-generation variations.
更多查看译文
关键词
Frequency control,Reinforcement learning,Power systems,Renewable energy sources,Batteries,Load modeling,Transfer functions,Load frequency control,deep reinforcement learning,twin delayed deep deterministic policy gradient (TD3),hybrid power system
AI 理解论文
溯源树
样例
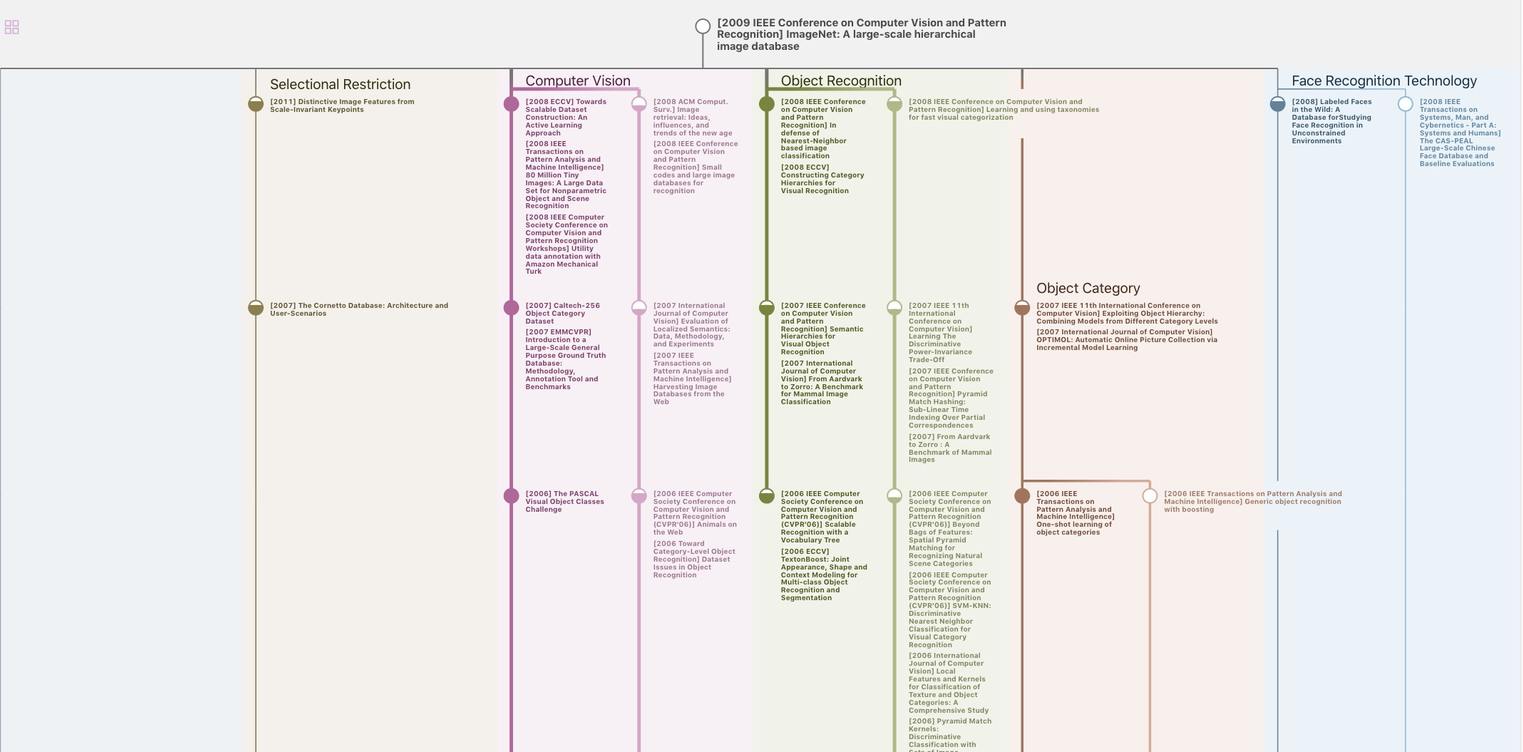
生成溯源树,研究论文发展脉络
Chat Paper
正在生成论文摘要