Reconstruction of 3D Images from Human Activity by a Compound Reconstruction Model
Cognitive Computation(2022)
摘要
Reconstructing visual stimuli from brain activity measured by functional magnetic resonance imaging (fMRI) is challenging for fMRI-based decoding. Some previous studies reconstructed 2D visual stimuli by using a voxel-wise encoding model and decoding model. Because nonlinear feature mappings were used in most previous studies, complicated nonlinear decoding methods, such as Bayesian model or deep learning methods, were needed to reconstruct 2D images and could increase the computation complexity and time cost. Although our previous study proposed contrast-disparity local decoding model to reconstruct 3D images from brain activity, the time cost of local decoding models increased with the size of images. In this study, we proposed a novel fast compound reconstruction model that combined the linear encoding–decoding model and the disparity decoding model to reconstruct 3D visual images from the fMRI responses. The results demonstrated that the linear encoding–decoding model successfully reconstructed the 2D contrasts of 3D images from the early visual regions while it failed to reconstruct 3D images directly. The proposed compound reconstruction model successfully reconstructed 3D images by combining the reconstructed 2D contrasts from the early visual region (V1) and the decoded disparities from the dorsal visual region (V3A and V7). In contrast to the contrast-disparity local decoding model, the compound reconstruction model showed significantly better reconstruction performance and much faster training speed. The successful reconstruction of the compound reconstruction model possibly suggested that the contrasts and disparity were firstly processed in two different visual pathways (early and dorsal) separately and the two pathways finally worked together to represent 3D images.
更多查看译文
关键词
fMRI, Linear feature mapping, Linear encoding model, Linear decoding model, 3D image reconstruction
AI 理解论文
溯源树
样例
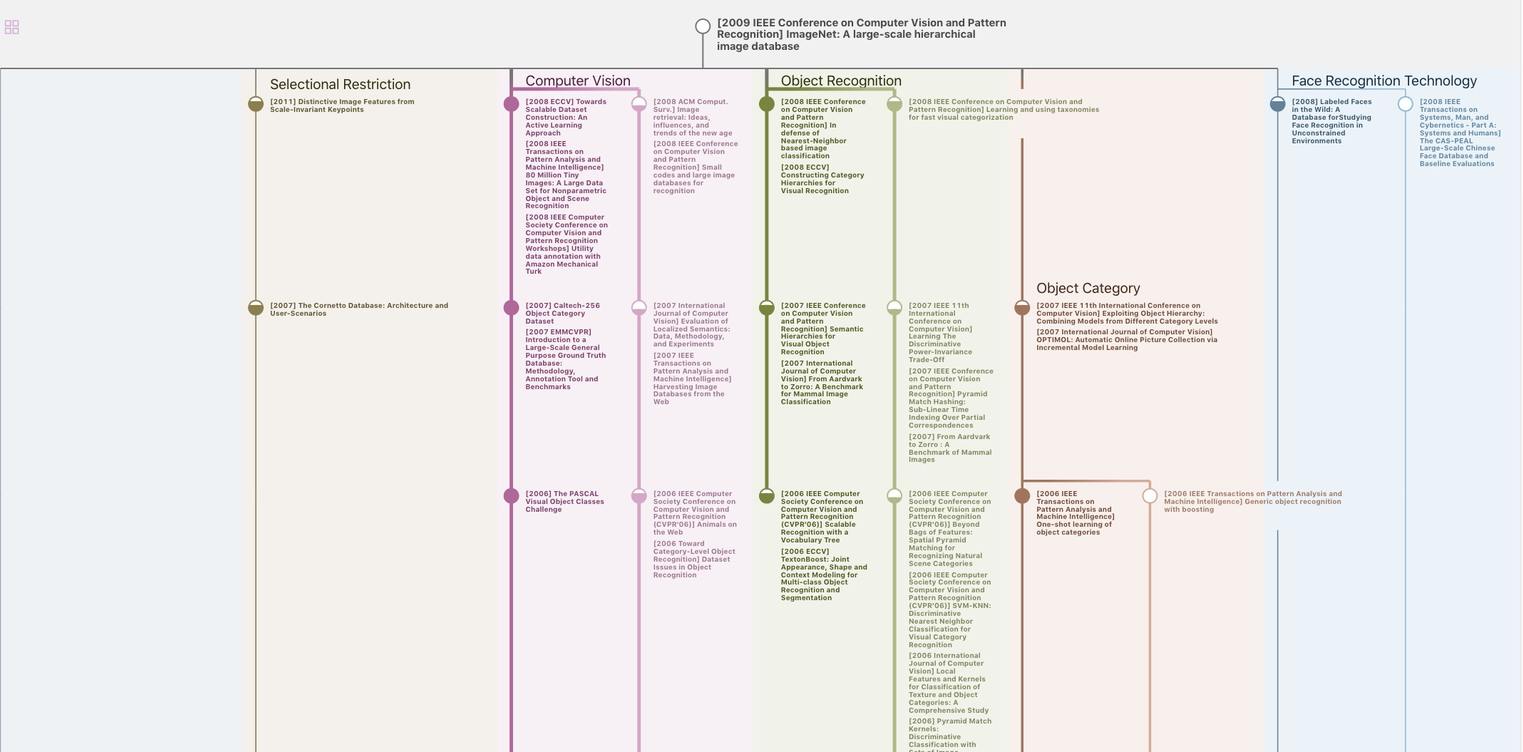
生成溯源树,研究论文发展脉络
Chat Paper
正在生成论文摘要