Costate-Supplement ADP for Model-Free Optimal Control of Discrete-Time Nonlinear Systems
IEEE TRANSACTIONS ON NEURAL NETWORKS AND LEARNING SYSTEMS(2024)
摘要
In this article, an adaptive dynamic programming (ADP) scheme utilizing a costate function is proposed for optimal control of unknown discrete-time nonlinear systems. The state-action data are obtained by interacting with the environment under the iterative scheme without any model information. In contrast with the traditional ADP scheme, the collected data in the proposed algorithm are generated with different policies, which improves data utilization in the learning process. In order to approximate the cost function more accurately and to achieve a better policy improvement direction in the case of insufficient data, a separate costate network is introduced to approximate the costate function under the actor-critic framework, and the costate is utilized as supplement information to estimate the cost function more precisely. Furthermore, convergence properties of the proposed algorithm are analyzed to demonstrate that the costate function plays a positive role in the convergence process of the cost function based on the alternate iteration mode of the costate function and cost function under a mild assumption. The uniformly ultimately bounded (UUB) property of all the variables is proven by using the Lyapunov approach. Finally, two numerical examples are presented to demonstrate the effectiveness and computation efficiency of the proposed method.
更多查看译文
关键词
Cost function,Optimal control,Mathematical models,Convergence,Estimation,Costs,Approximation algorithms,Adaptive dynamic programming (ADP),costate function,model-free control,optimal control
AI 理解论文
溯源树
样例
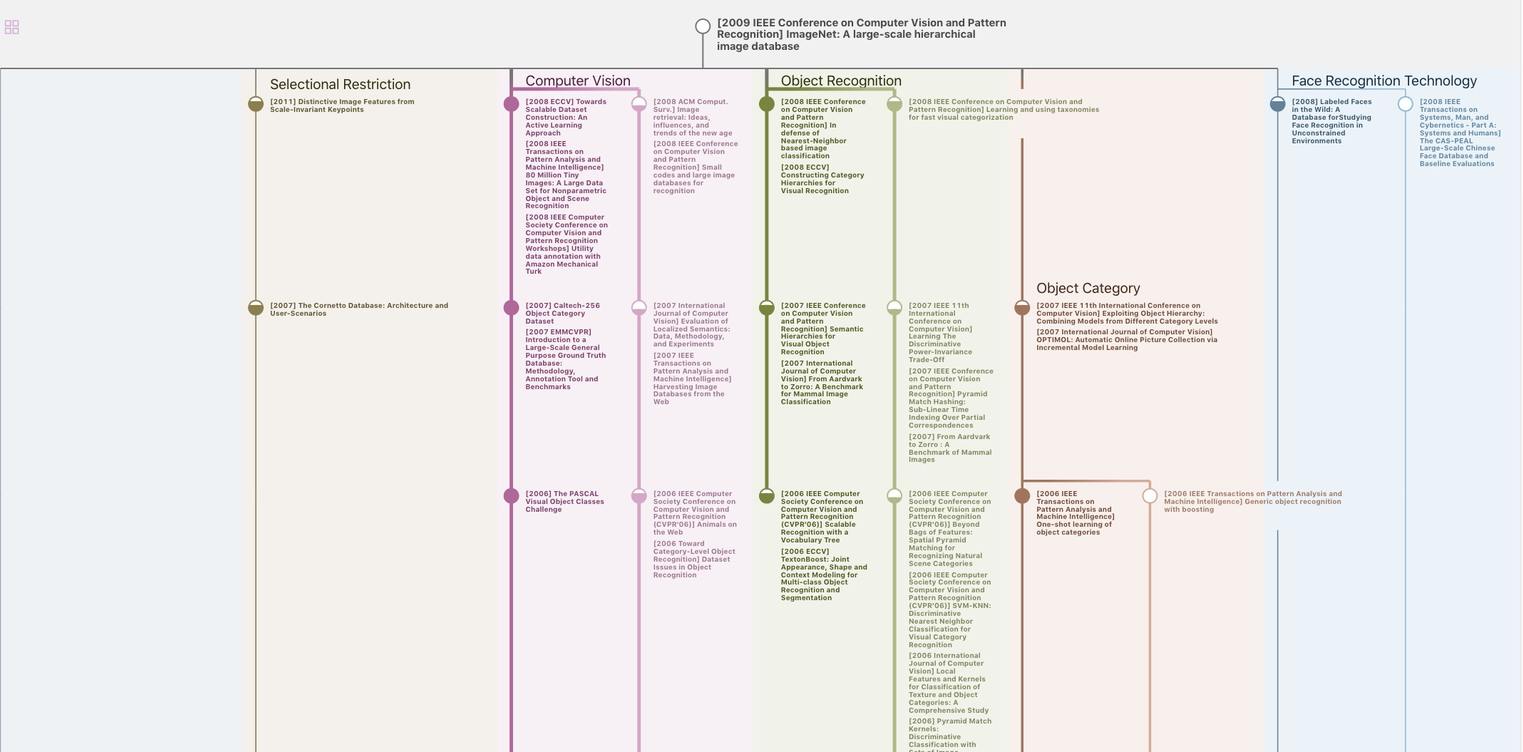
生成溯源树,研究论文发展脉络
Chat Paper
正在生成论文摘要