Forecasting Damaged Containers with Machine Learning Methods
DIGITIZING PRODUCTION SYSTEMS, ISPR2021(2022)
摘要
Forecasting the number of damaged containers is crucial for a maritime company to effectively plan future port operations. The purpose of this study is to forecast damaged container entries and exits. In this paper, we worked with a global logistics company in Turkey. Comparisons between ports were made using the company's internal port operations data and externally available data. The external data that we used are Turkey's GDP, exchange rates (USD/EUR), import and export data, TEU of Mersin port and the total TEU of Turkey's ports (2015-2020). Our aim is to forecast the number of damaged containers at a specific port (Mersin, Turkey) using different machine learning methods and find the best method. We used Linear Regression, Boosted Decision Tree Regression, Decision Forest Regression and Artificial Neural Network Regression algorithms. The performances of these methods were evaluated according to various metrics, such as R-2, MAE, RMSE, RAE and RSE. According to our results, machine learning methods can forecast container demand effectively and the best performing method is Boosted Decision Tree regression.
更多查看译文
关键词
Logistics, Forecasting, Container demand, Machine learning, Regression
AI 理解论文
溯源树
样例
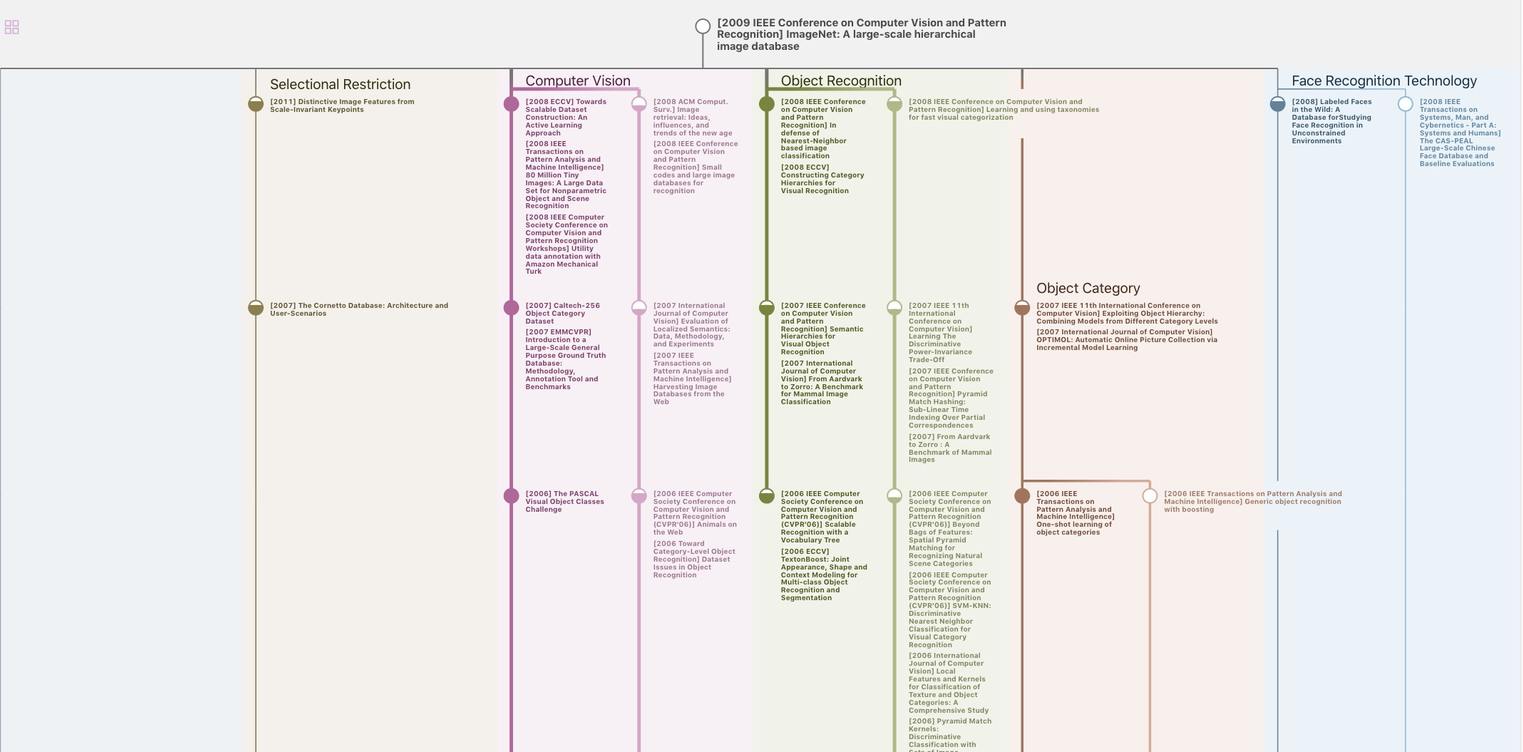
生成溯源树,研究论文发展脉络
Chat Paper
正在生成论文摘要