Spectral-Spatial Correlation Exploration for Hyperspectral Image Classification via Self-Mutual Attention Network
IEEE GEOSCIENCE AND REMOTE SENSING LETTERS(2022)
摘要
Recently, deep learning methods have been widely used to extract spectral-spatial features for hyperspectral image (HSI) classification and dramatically boost the performance. However, most of them usually take the original HSI cube as the input, where spectral-spatial information is mixed together. Consequently, they cannot explicitly model the inherent correlation (e.g., complementary relation) between spectral and spatial domains, limiting the classification performance. To alleviate this issue, a spectral-spatial self-mutual attention network (S(3)MANet) is proposed in this letter. It, respectively, extracts spectral and spatial features via the corresponding feature module. Subsequently, a self-mutual attention module is designed to enhance these features. More concretely, it performs feature interaction to emphasize the correlation of spectral and spatial domains via mutual attention while self-attention is applied to each domain for learning long-range dependencies. Finally, we infer two classification results from the enhanced spectral and spatial features, and a weighted summation is further applied to obtain a joint spectral-spatial results. Experimental results on two public HSI datasets validate that the proposed S(3)MANet could achieve more satisfactory performance in comparison with several state-of-the-art methods.
更多查看译文
关键词
Feature extraction, Convolution, Hyperspectral imaging, Correlation, Spectral analysis, Convolutional neural networks, Three-dimensional displays, Convolutional neural network (CNN), hyperspectral image (HSI) classification, mutual attention, self-attention
AI 理解论文
溯源树
样例
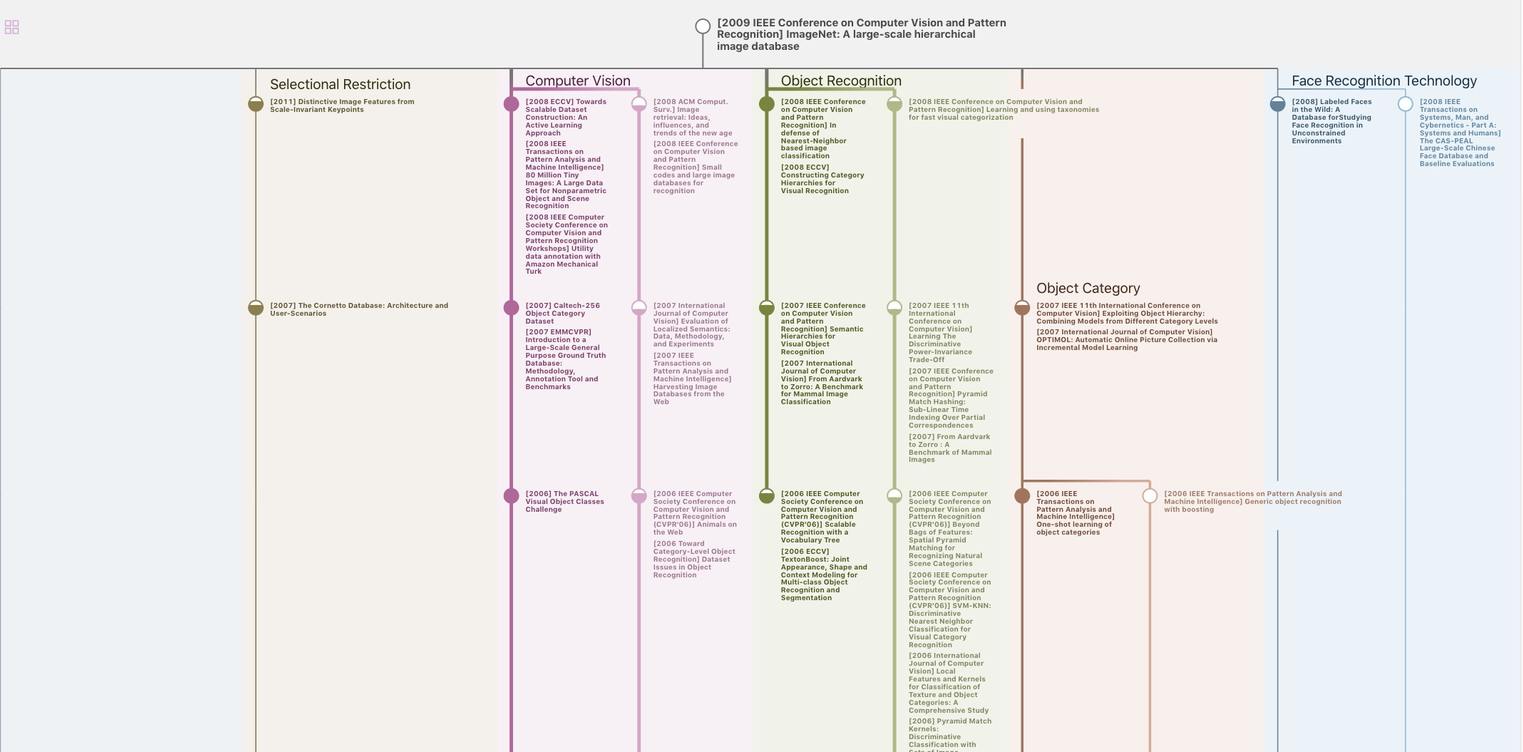
生成溯源树,研究论文发展脉络
Chat Paper
正在生成论文摘要