Detecting Marine Organisms Via Joint Attention-Relation Learning for Marine Video Surveillance
IEEE Journal of Oceanic Engineering(2022)
摘要
The better way to understand marine life and ecosystems is to surveil and analyze the activities of marine organisms. Recently, research on marine video surveillance is becoming increasingly popular. With the rapid development of deep learning (DL), convolutional neural networks (CNNs) have made remarkable progresses in image/video understanding tasks. In this article, we explore a visual attention and relation mechanism for marine organism detection, and propose a new way to apply an improved attention-relation (AR) module on an efficient marine organism detector (EMOD), which can well enhance the discrimination of organisms in complex underwater environments. We design our EMOD via integrating current state-of-the-art (SOTA) detection methods in order to detect organisms and surveil marine environments in a real time and fast fashion for high-resolution marine video surveillance. We implement our EMOD and AR on the annotated video data sets provided by the public data challenges in conjunction with the workshops (CVPR 2018 and 2019), which are supported by National Oceanic and Atmospheric Administration (NOAA) and their research works (NMFS-PIFSC-83). Experimental results and visualizations demonstrate that our application of AR module is effective and efficient, and our EMOD equipped with AR modules can outperform SOTA performance on the experimental data sets. For application requirements, we also provide the application suggestions of EMOD framework. Our code is publicly available at
https://github.com/zhenglab/EMOD
.
更多查看译文
关键词
Convolutional neural network (CNN),marine organism detection,marine video surveillance,relation model,visual attention
AI 理解论文
溯源树
样例
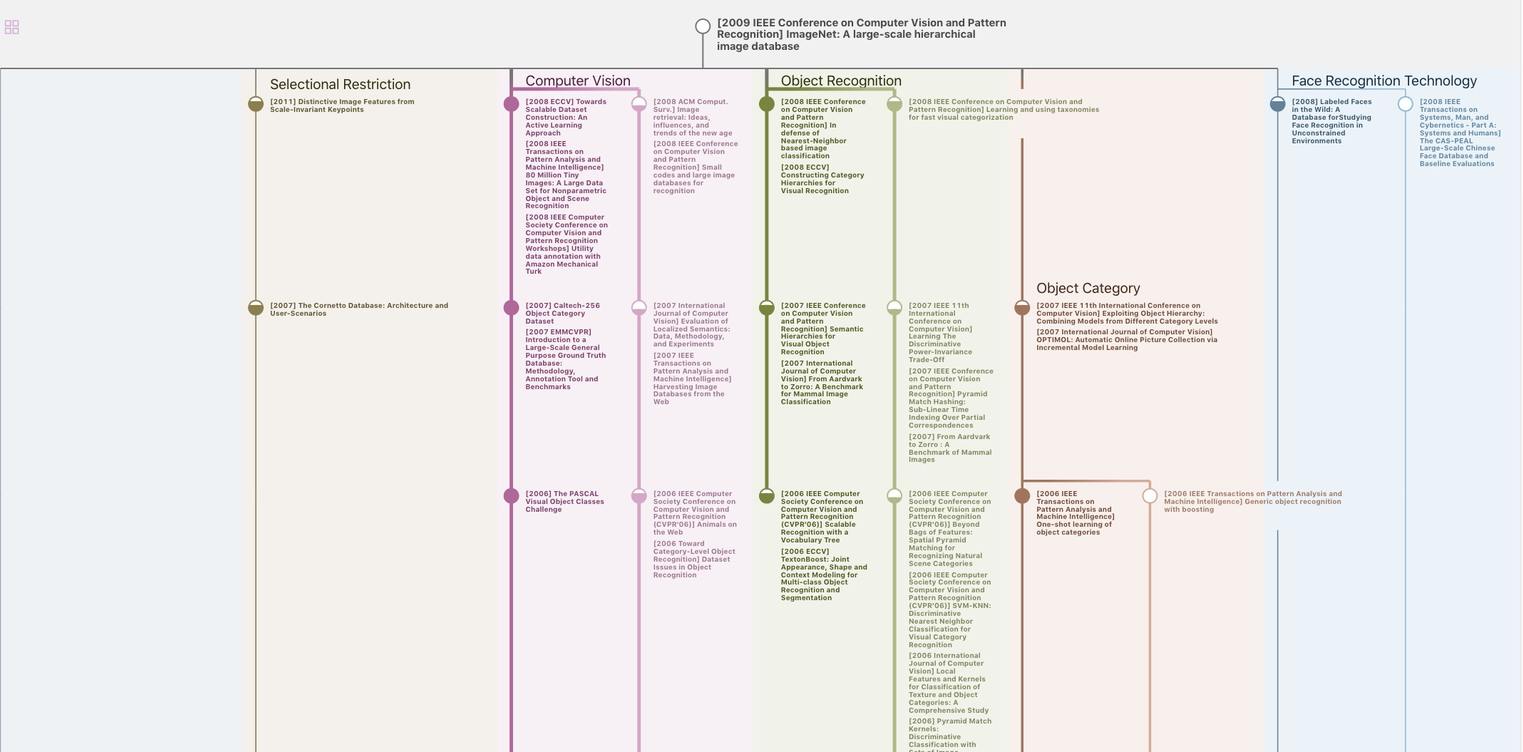
生成溯源树,研究论文发展脉络
Chat Paper
正在生成论文摘要