A novel hybrid water quality forecast model based on real-time data decomposition and error correction
Process Safety and Environmental Protection(2022)
Abstract
Accurate forecast for water quality is of great importance because it can support water resource management with the future information. In this research, we propose a novel hybrid model by using data decomposition, error correction, and machine learning. In our method, first, the initial forecast is obtained by a prediction model that uses improved complete ensemble empirical mode decomposition with adaptive noise and bidirectional long short-term memory (BLSTM) neural network. Next, a novel error correction framework, which is built by variational mode decomposition and BLSTM neural network, is used to improve forecast accuracy by correcting the initial forecast error. Water quality data of Poyang Lake, China is used to evaluate our model. Results indicate that our model shows highly accurate forecast performance for all of the 9 water quality datasets (the average of mean absolute percentage error (MAPE) of 7 day-ahead forecast is 2.12%; 30 day-ahead forecast is 4.06%). In addition, our model outperforms the competitor models, particularly, compared to the prediction model without error correction framework, the average of MAPE is reduced by 33.33% for 7 day-ahead forecast; 30.48% for 30 day-ahead forecast. This research demonstrates that the proposed error correction framework is an effective tool to improve forecast accuracy for water quality.
MoreTranslated text
Key words
BLSTM,CEEMDAN,DO,Dstat,EEMD,ELM,EMD,ICEEMDAN,IOFA,LSTM,MAE,MAPE,NH3-H,RMSE,SDE,VMD,CB,CB-VB,IB,IB-B,IB-VB,VB
AI Read Science
Must-Reading Tree
Example
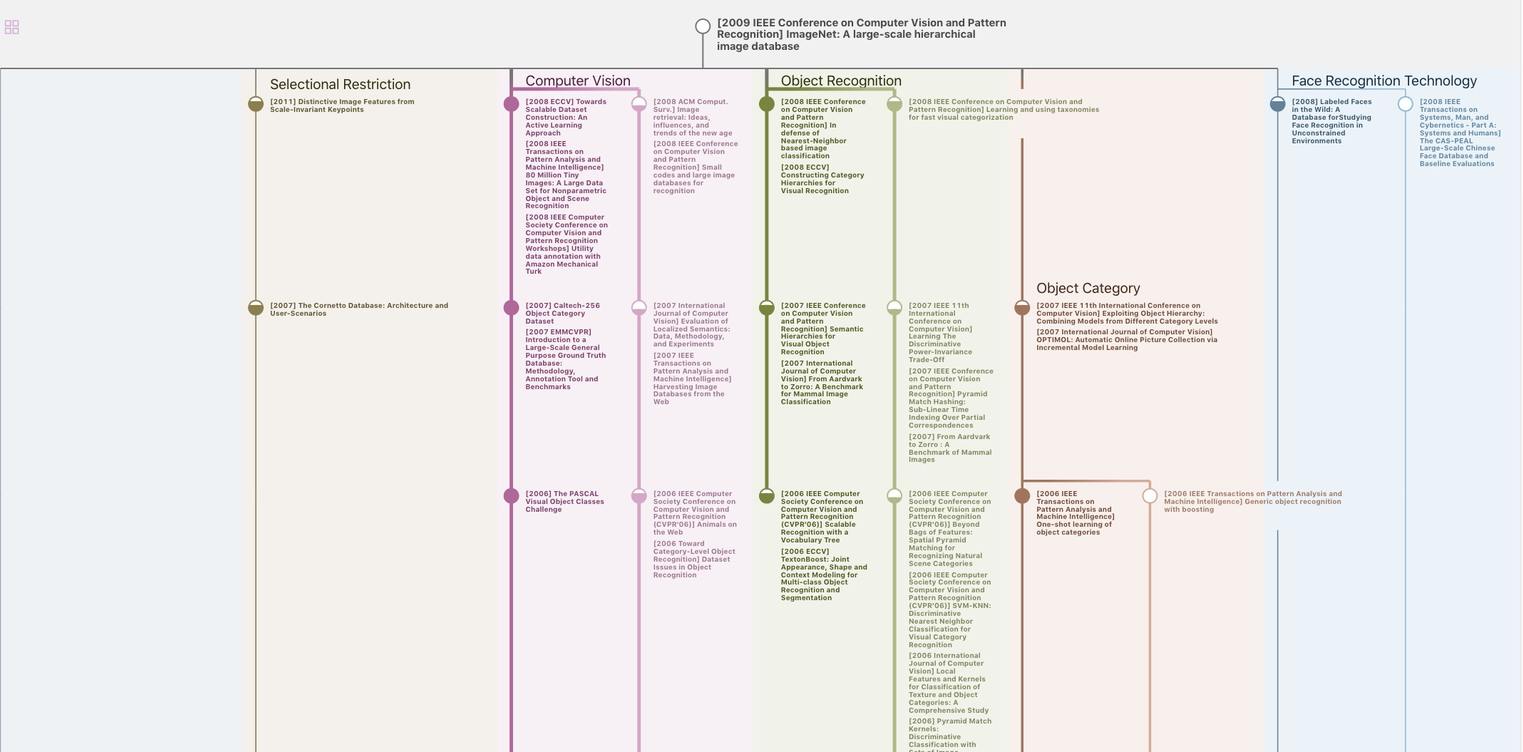
Generate MRT to find the research sequence of this paper
Chat Paper
Summary is being generated by the instructions you defined