Loss-Based Performance Assessment and Seismic Network Optimization for Earthquake Early Warning
BULLETIN OF THE SEISMOLOGICAL SOCIETY OF AMERICA(2022)
摘要
The goal of earthquake early warning (EEW) is to issue an alert before the damaging seismic waves of an earthquake hit a given exposure. We develop a framework to evaluate the EEW performance in a loss-based context. We use warning time as a key performance indicator and determine statistics of warning time by loss severity to assess the rate and consistency with which an EEW system can deliver timely alerts. In the second part of this work, we develop a Genetic Algorithm approach to optimize an existing sensor network by proposing sites for new stations to enhance the EEW performance in damaging earthquakes. We demonstrate this framework for Switzerland using 2000 realizations of a 50-yr-long stochastic earthquake catalog, which samples the earthquake rate forecast of the Swiss Hazard Model in space and time. For each of the almost 24k earthquake scenario ruptures (5.0 <= M <= 7.4), we predict shaking intensities and losses (here, fatalities and injuries) at the largest Swiss cities. We find that the current Swiss Seismic Network could provide positive warning times to the affected sites for about 80% of very damaging earthquakes (>= 100 fatalities) and for around 85% of earthquakes with >= 10 fatalities. Warning times of > 5 s could be achieved for about 40%-55% of very damaging earthquakes and > 10 s for about 35%. For around 50% of events with >= 1 fatality (>= 10 injuries), EEW could provide > 15 s of warning. The greatest benefit of EEW is expected in Zurich, where the population density and, consequently, absolute long-term expected losses are highest and the warning times for damaging earthquakes are often long (> 15 s). Densifying the Swiss Seismic Network with additional stations can increase warning times in selected scenarios by up to 5 s. However, because it is already very dense (7 +/- 5 km interstation distance), decreasing data latencies (currently similar to 2 s) may be more important.
更多查看译文
AI 理解论文
溯源树
样例
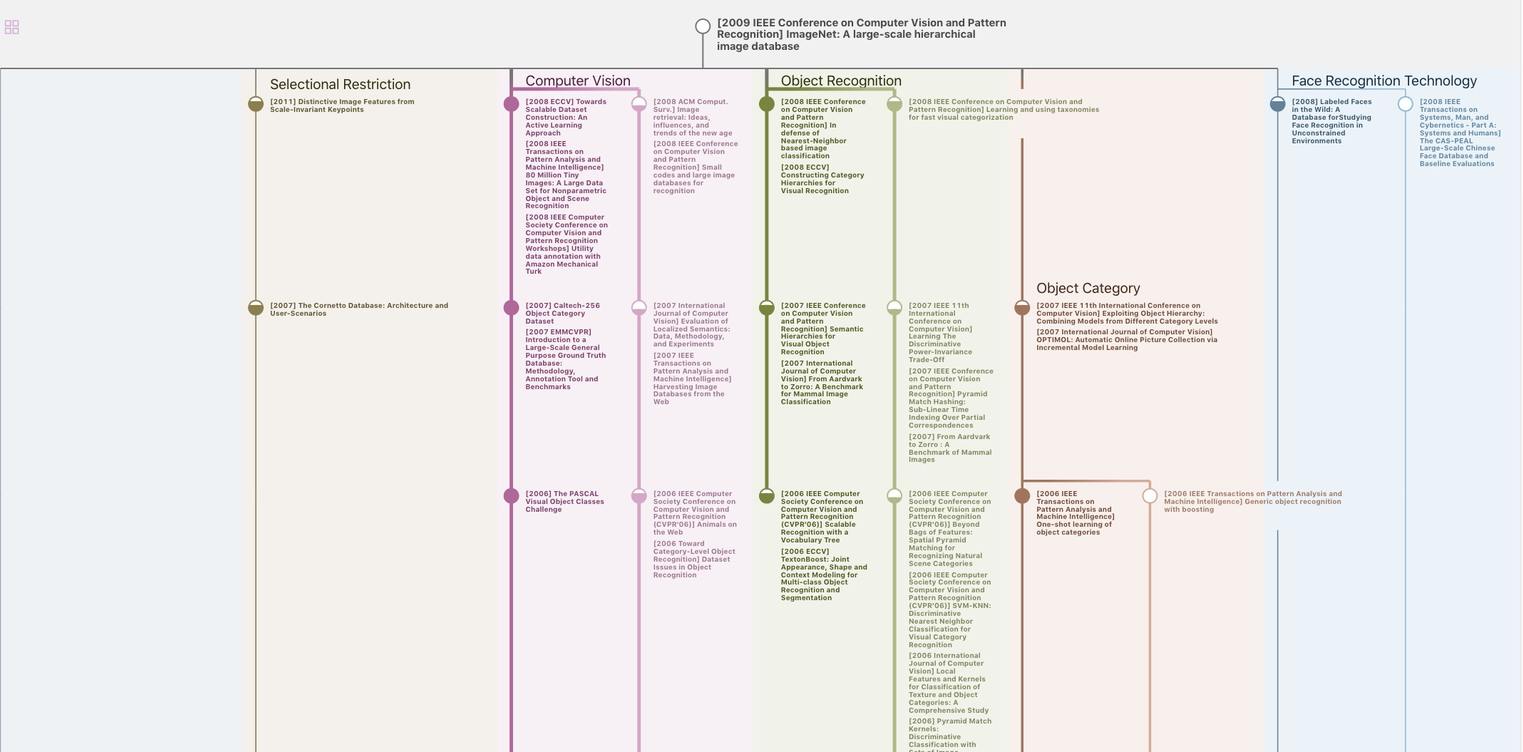
生成溯源树,研究论文发展脉络
Chat Paper
正在生成论文摘要