Generalization in transfer learning: robust control of robot locomotion
ROBOTICA(2022)
摘要
In this paper, we propose a set of robust training methods for deep reinforcement learning to transfer learning acquired in one control task to a set of previously unseen control tasks. We improve generalization in commonly used transfer learning benchmarks by a novel sample elimination technique, early stopping, and maximum entropy adversarial reinforcement learning. To generate robust policies, we use sample elimination during training via a method we call strict clipping. We apply early stopping, a method previously used in supervised learning, to deep reinforcement learning. Subsequently, we introduce maximum entropy adversarial reinforcement learning to increase the domain randomization during training for a better target task performance. Finally, we evaluate the robustness of these methods compared to previous work on simulated robots in target environments where the gravity, the morphology of the robot, and the tangential friction coefficient of the environment are altered.
更多查看译文
关键词
deep reinforcement learning, transfer learning, control of robotic systems, bipeds, humanoid robots, legged robots, service robots, space robotics, mobile robots, adversarial learning, robot locomotion
AI 理解论文
溯源树
样例
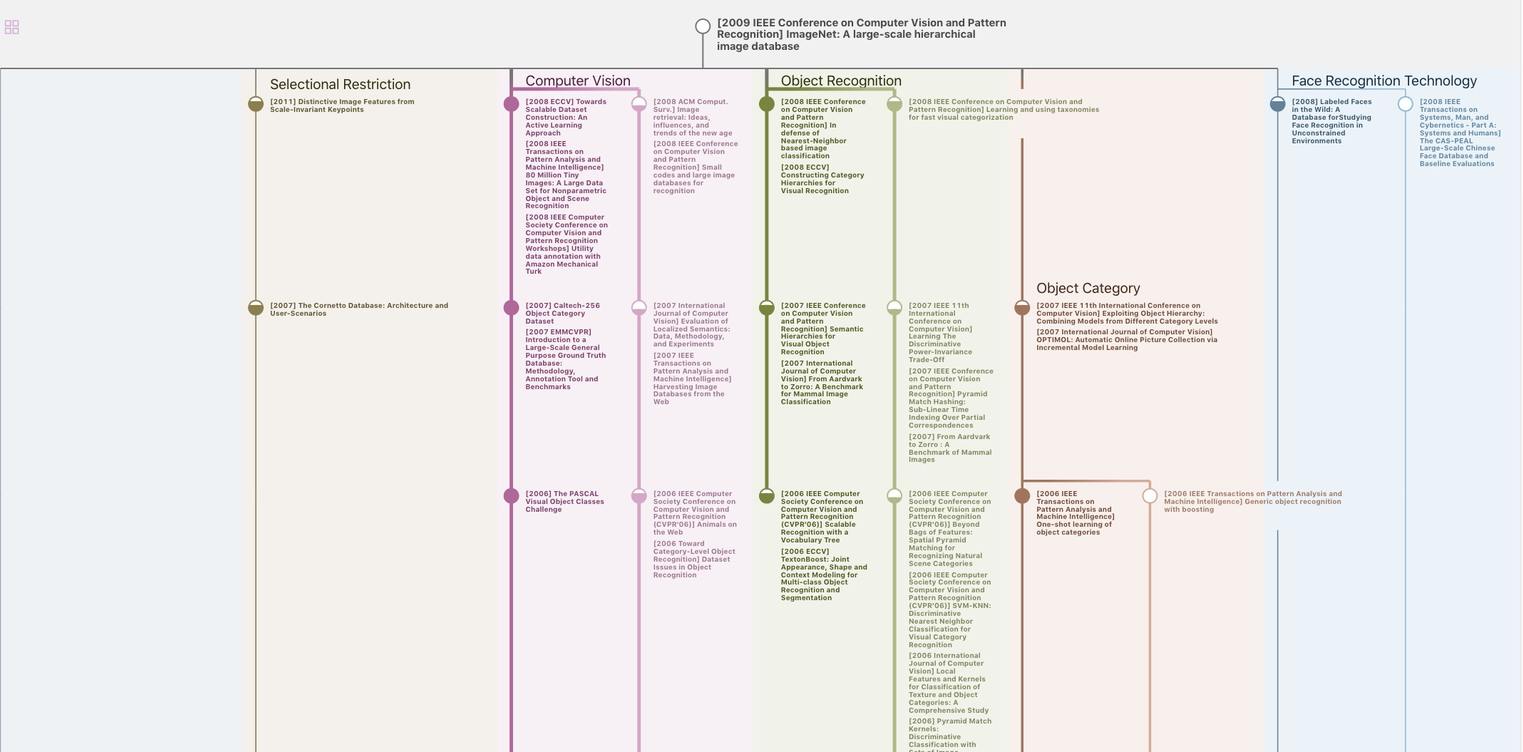
生成溯源树,研究论文发展脉络
Chat Paper
正在生成论文摘要