Detection of Image Steganography Using Deep Learning and Ensemble Classifiers
ELECTRONICS(2022)
摘要
In this article, the problem of detecting JPEG images, which have been steganographically manipulated, is discussed. The performance of employing various shallow and deep learning algorithms in image steganography detection is analyzed. The data, images from the BOSS database, were used with information hidden using three popular steganographic algorithms: JPEG universal wavelet relative distortion (J-Uniward), nsF5, and uniform embedding revisited distortion (UERD) at two density levels. Various feature spaces were verified, with the discrete cosine transform residuals (DCTR) and the Gabor filter residuals (GFR) yielding best results. Almost perfect detection was achieved for the nsF5 algorithm at 0.4 bpnzac density (99.9% accuracy), while the detection of J-Uniward at 0.1 bpnzac density turned out to be hardly possible (max. 56.3% accuracy). The ensemble classifiers turned out to be an encouraging alternative to deep learning-based detection methods.
更多查看译文
关键词
steganography, machine learning, image processing, BOSS database, ensemble classifier, deep learning, steganalysis, stegomalware
AI 理解论文
溯源树
样例
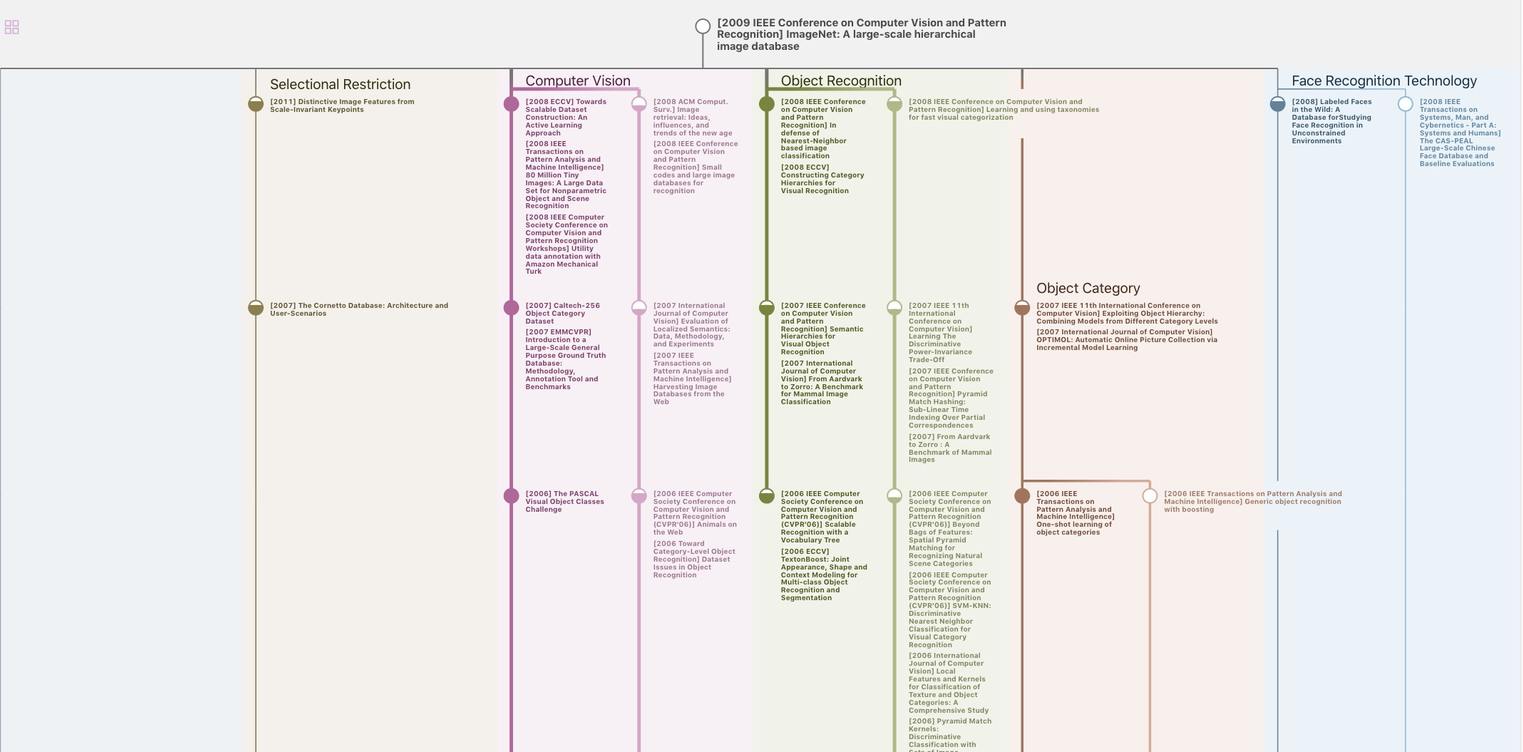
生成溯源树,研究论文发展脉络
Chat Paper
正在生成论文摘要