Modeling Energy Gap of Doped Tin (II) Sulfide Metal Semiconductor Nanocatalyst Using Genetic Algorithm-Based Support Vector Regression
JOURNAL OF NANOMATERIALS(2022)
摘要
Tin (II) sulfide (SnS) is a metal chalcogenide semiconducting material with fascinating and admirable physical features for practical applications in solid-state batteries, photodetectors, gas sensors, optoelectronic devices, emission transistors, and photocatalysis among others. The energy gap of SnS semiconductor nanomaterial that facilitates its usefulness in many applications can be adjusted through dopant incorporation which results in crystal lattice distortion at various crystallite sizes of the semiconductor. This work employs lattice parameter descriptors to develop a hybrid genetic algorithm (GA) and support vector regression algorithm (SVR) intelligent model for determining the energy gap of doped SnS semiconductors. The predictive strength of the developed GA-SVR model is compared with the stepwise regression algorithm- (STRA-) based model using different performance evaluation parameters. The developed GA-SVR model performs better than STRA model based on root mean square error, mean absolute error, and correlation coefficient with performance improvement of 70.68%, 67.63%, and 20.98%, respectively, using the testing set of data. Influence of different dopants and experimental conditions on energy gap of SnS semiconductor were investigated using the developed model, while the obtained values for the energy gaps agree with the measured values. The developed models demonstrate high degree of potentials in terms of accuracy, precision, and ease of implementation that fosters their real-life applicability in estimating the energy gap of doped SnS semiconductor with experimental stress circumvention.
更多查看译文
AI 理解论文
溯源树
样例
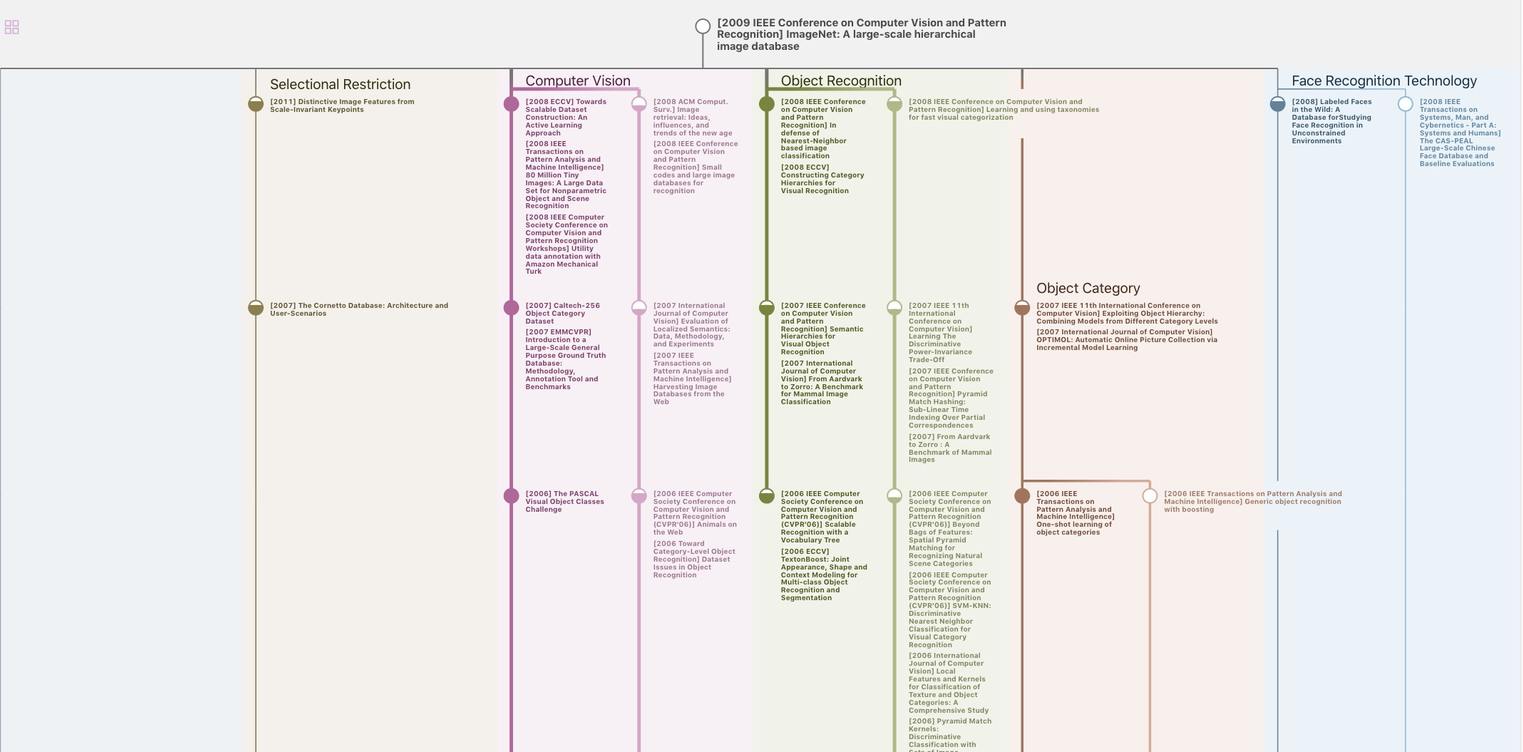
生成溯源树,研究论文发展脉络
Chat Paper
正在生成论文摘要