Vote-based integration of review spam detection algorithms
Applied Intelligence(2022)
摘要
Due to the growth of online review data, detecting fake or fraudulent reviews is becoming an urgent issue. One barrier to effective detection of fake reviews/reviewers is the great difficulty of collecting ground-truth data—fake reviews are hard to judge, even by human experts. As researchers propose a large number of methods to detect review spam from a variety of perspectives, e.g., text-based or behavior-based, there is a need to combine these methods to improve the overall detection performance. In this paper, we raise the important question of how to integrate multiple ranking lists generated by different types of review spam detection algorithms into an overall ranking list. To address this problem, we propose a novel unsupervised integration model, namely SpamVote , that combines multiple ranking lists together by voting. In view of the diversity of review spam strategies, we model the fitness of a particular algorithm to detect a specific item as latent, and learn the latent variables from the ranking data. Extensive experiments on real-world datasets with various kinds of algorithms show that the integrated ranking list created by SpamVote outperforms the voting lists with a large probability.
更多查看译文
关键词
Review spam detection, Ranking list, Integration, Vote
AI 理解论文
溯源树
样例
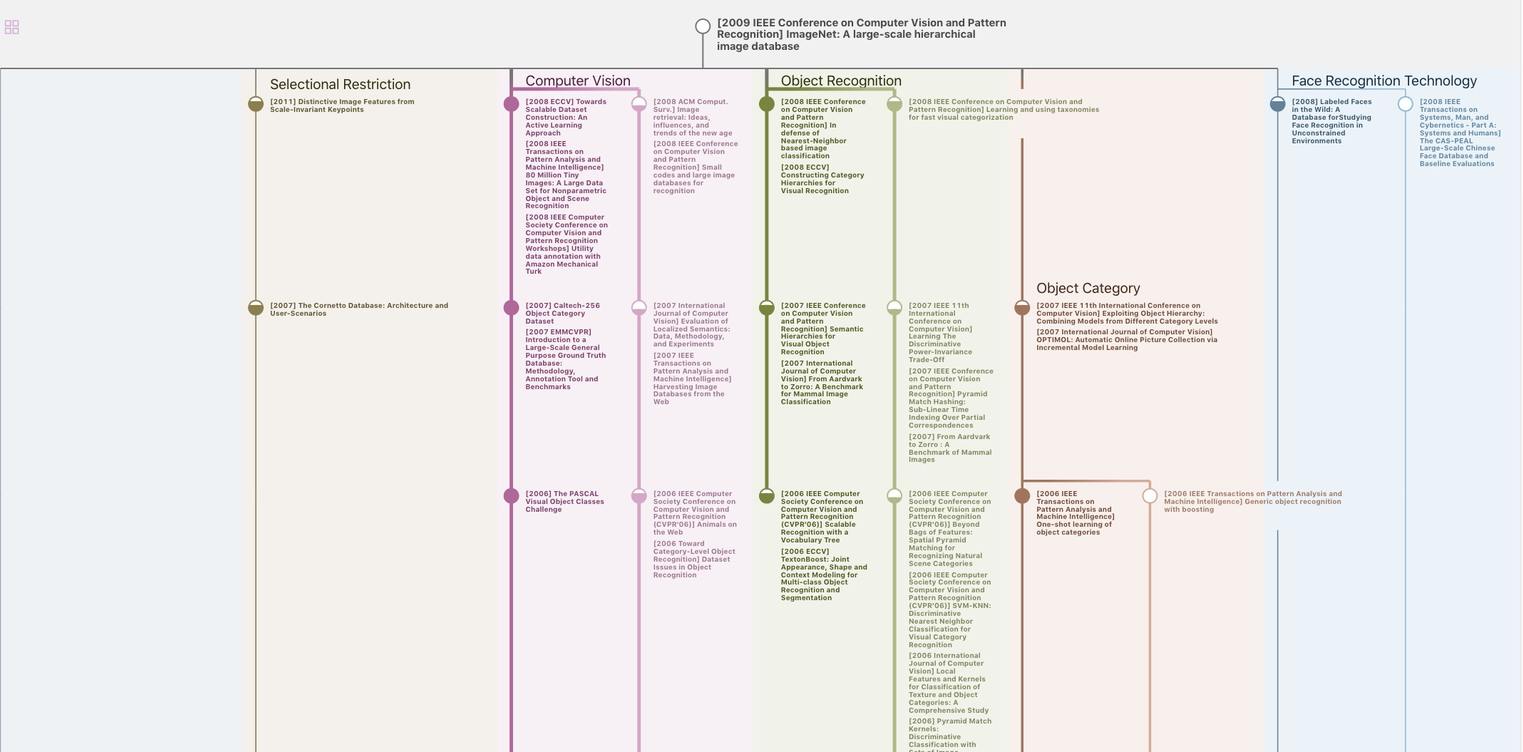
生成溯源树,研究论文发展脉络
Chat Paper
正在生成论文摘要