A multi-fault advanced diagnosis method based on sparse data observers for lithium-ion batteries
JOURNAL OF ENERGY STORAGE(2022)
Abstract
Lithium-ion batteries are a typical energy storage device involving complex electrochemical reactions and transfer mechanisms. In order to ensure the safety and durability of the battery, it is crucial to accurately diagnose and predict the battery faults in the early stage. Therefore, a real-time multi-fault advanced diagnosis method based on Sparse Data Observers (SDO) is proposed in this paper. This method can diagnose and predict battery faults without obvious abnormalities, including short circuit and open circuit faults. Firstly, calculating the outlierness score of the voltage sequence, and then the faulty battery and the fault time are identified by fault flags. Finally, the correction coefficient is introduced to detect the fault types. Moreover, this method does not require an accurate battery model, but only needs the voltage data measured from the battery. Also, it is not affected by cell inconsistency and measurement noise. The experimental results verify the feasibility and effectiveness of the proposed method, which has strong robustness and high sensitivity.
MoreTranslated text
Key words
Lithium-ion batteries, Fault diagnosis, Sparse data observers, Outlier detection, Electric vehicles
AI Read Science
Must-Reading Tree
Example
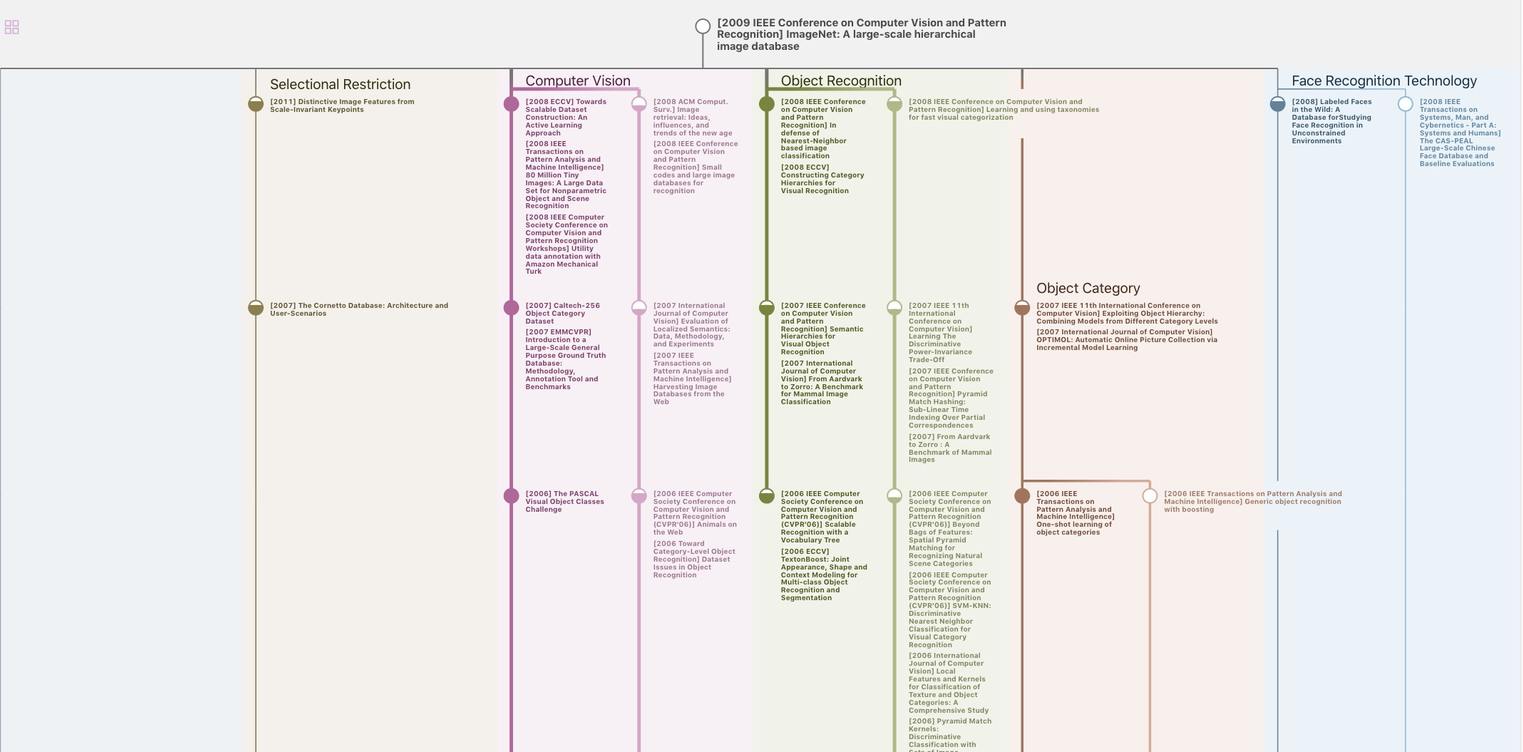
Generate MRT to find the research sequence of this paper
Chat Paper
Summary is being generated by the instructions you defined