Multiobjective Reinforcement Learning Based Energy Consumption in C-RAN Enabled Massive MIMO
RADIOENGINEERING(2022)
摘要
Multiobjective optimization has become a suitable method to resolve conflicting objectives and enhance the performance evaluation of wireless networks. In this study, we consider a multiobjective reinforcement learning (MORL) approach for the resource allocation and energy consumption in C-RANs. We propose the MORL method with two conflicting objectives. Herein, we define the state and action spaces, and reward for the MORL agent. Furthermore, we develop a Q-learning algorithm that controls the ON-OFF action of remote radio heads (RRHs) depending on the position and nearby users with goal of selecting the best single policy that optimizes the trade-off between EE and QoS. We analyze the performance of our Q -learning algorithm by comparing it with simple ON-OFF scheme and heuristic algorithm. The simulation results demonstrated that normalized ECs of simple ON-OFF, heuristic and Q-learning algorithm were 0.99, 0.85, and 0.8, respectively. Our proposed MORL-based Q-learning algorithm achieves superior EE performance compared with simple ON-OFF scheme and heuristic algorithms.
更多查看译文
关键词
Convergence, energy consumption, reinforcement , learning, reward, optimization
AI 理解论文
溯源树
样例
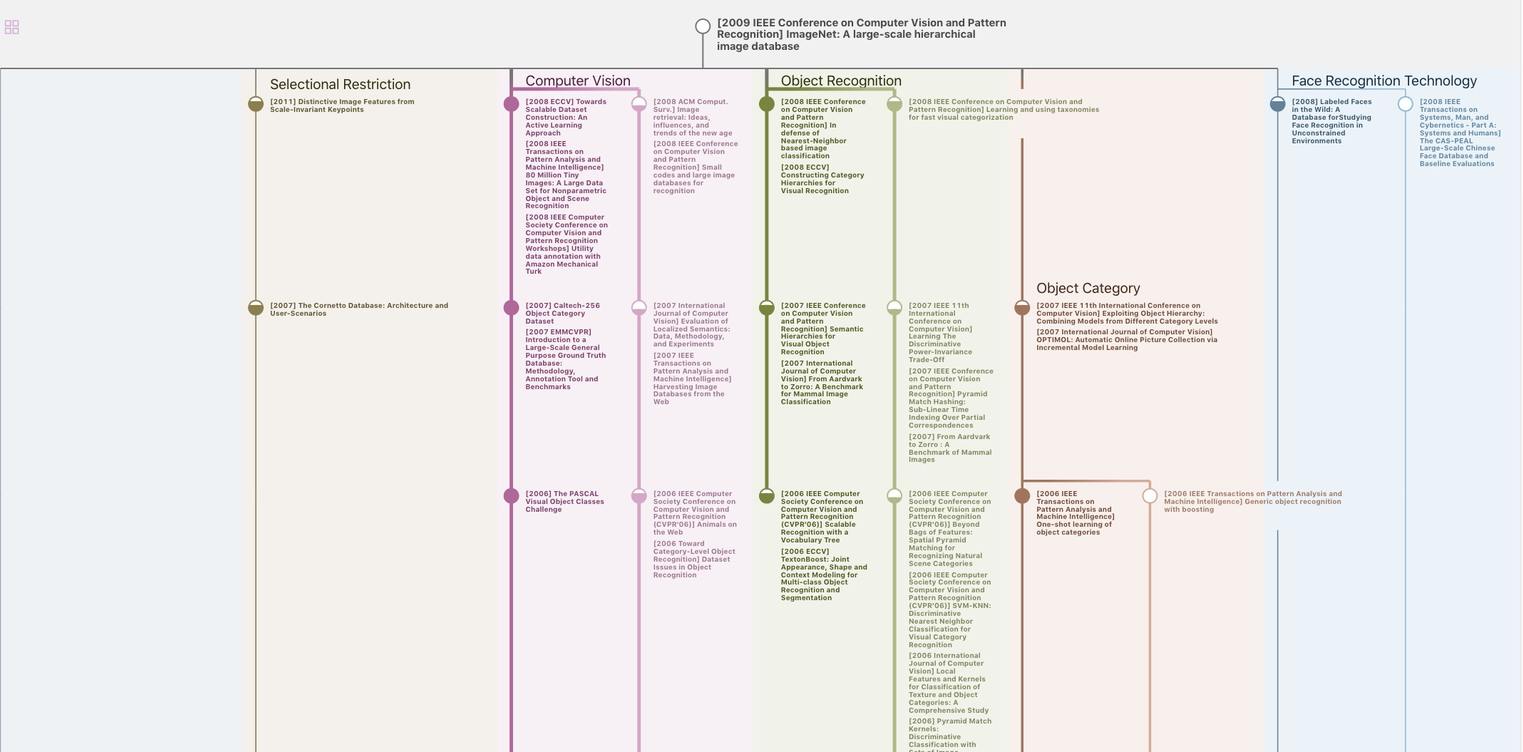
生成溯源树,研究论文发展脉络
Chat Paper
正在生成论文摘要