Multivariate stacked bidirectional long short term memory for lithium-ion battery health management
Reliability Engineering & System Safety(2022)
摘要
Prognostics and health management (PHM) will ensure the safe and reliable operation of the battery systems. The remaining useful life (RUL) prediction as one of the major PHM strategies gives early warning of faults, which can be applied to recognize the necessary battery maintenance and replacement in advance. This study investigates a novel deep learning method for predicting lithium-ion battery RUL, which can learn the long-term dependency of degradation trend of batteries. This is the first time which a stacked bidirectional long short-term memory (SBLSTM) based on extreme gradient boosting (XGBoost) is applied to predict the battery capacity degradation trajectories. Using the XGBoost technique, important time-domain features are selected as multivariate inputs to feed the deep learning model for predicting. To improve the trained model, Bayesian optimization (BO) is also performed to tune the hyper-parameters. The findings show that the SBLSTM model achieves a low root mean square percentage error of 1.94%, which is lower than the state-of-the-art methods due to two-way learning. The suggested model will provide excellent support for the maintenance strategy development and health management of the battery systems.
更多查看译文
关键词
Lithium-ion battery,Remaining useful life,Multivariate time series,Stacked bidirectional long short term memory
AI 理解论文
溯源树
样例
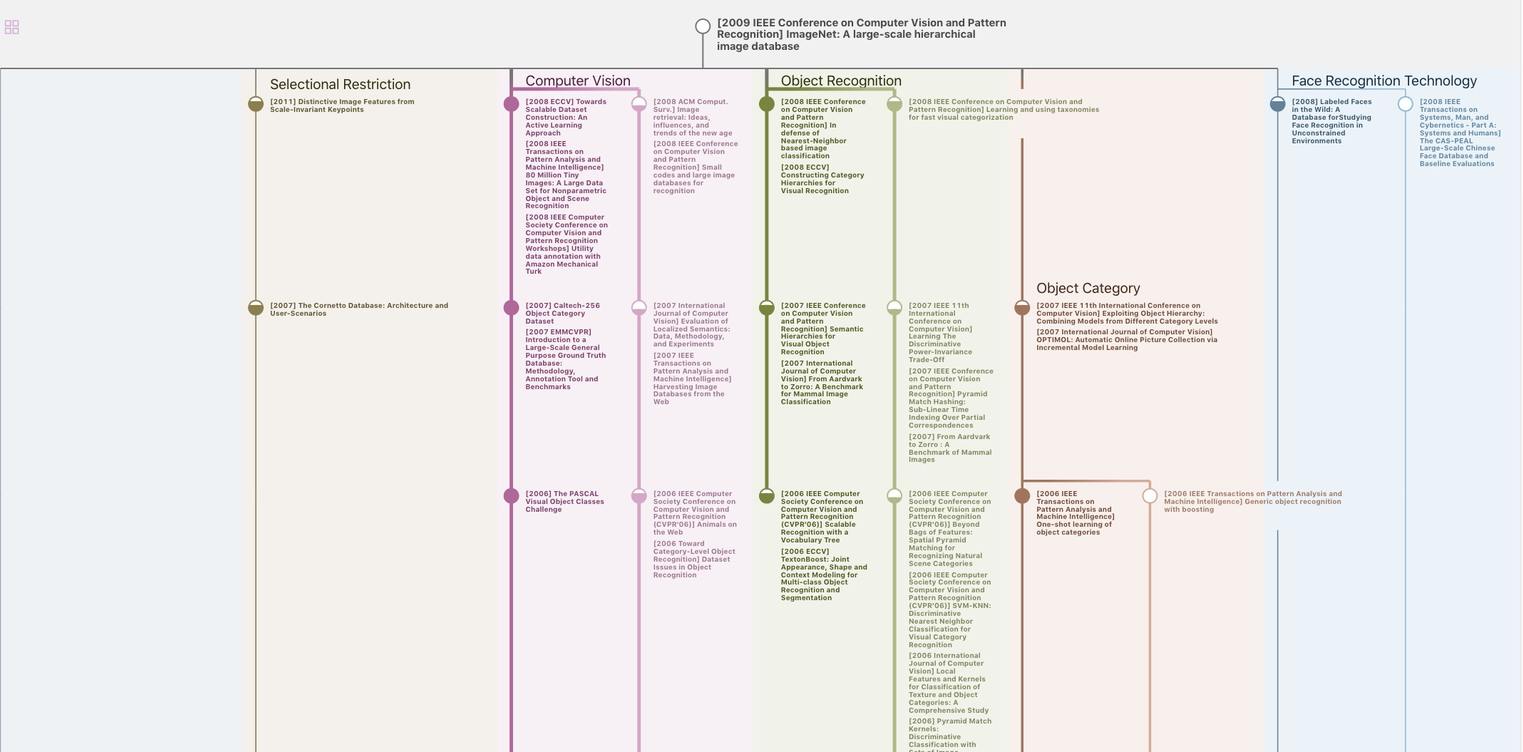
生成溯源树,研究论文发展脉络
Chat Paper
正在生成论文摘要