Machine learning analysis of features extracted from time–frequency domain of ultrasonic testing results for wood material assessment
Construction and Building Materials(2022)
摘要
In this paper, a systematic machine learning strategy is proposed to classify wood properties based on a contact ultrasonic testing results. As such, several aspects of the wood material including the type of wood (hardwood or softwood), the direction of an ultrasonic test with respect to the growth rings of the wood, and, whether the wood is damaged or intact are investigated. As a pre-processor, the Variational Mode Decomposition technique is applied to the nonlinearly modulated ultrasonic signals, and the centre frequencies of the decomposition results are taken as features for Machine learning Algorithms (MLAs). Then with each of the MLAs, hyperparameter settings were optimised and technical aspects of the feature engineering are discussed. Best results were achieved using Ensemble classifiers, SVM, and KNN using three features/decomposition. To explore the physics behind the nonlinear problem, the relative false discovery rate obtained from the confusion matrix associated with applying the MLAs is proposed as a metric. We show that different features are capable of exploring different aspects of the problem better. The techniques of this paper can be applied to quality assessment of wood materials. The paper also demonstrates the capability of MLAs in exploring some physics of such problems. Moreover, billets with natural imperfections harvested from the site Collie in WA, Australia are classified to demonstrate the applicability of the proposed approach in real world problems. The result of 94% 5-fold cross-validation accuracy indicates the effectiveness of the proposed approach.
更多查看译文
关键词
Ultrasonics,Variational Mode Decomposition,Machine learning,Nondestructive damage testing,Wood
AI 理解论文
溯源树
样例
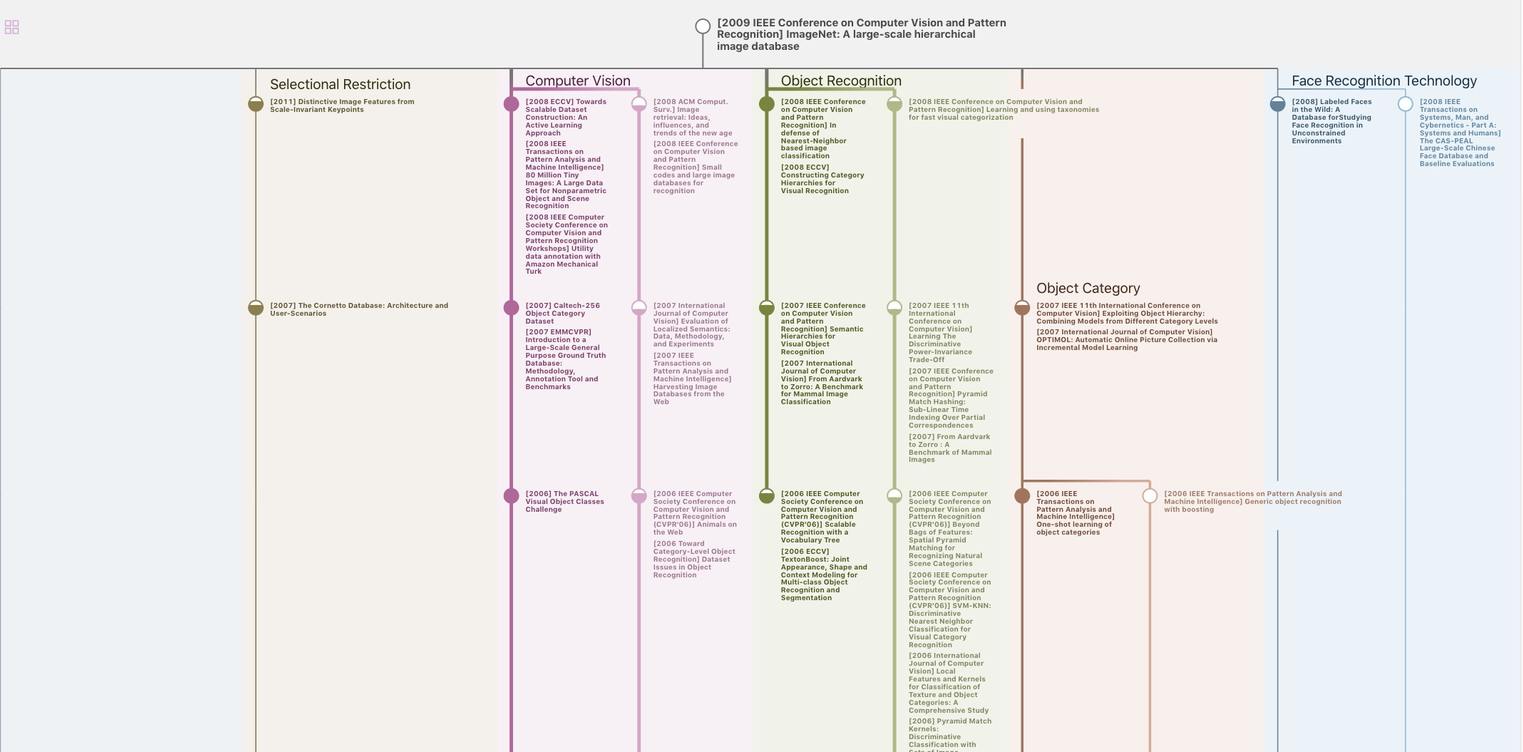
生成溯源树,研究论文发展脉络
Chat Paper
正在生成论文摘要