Depth Estimation from a Single CD-SEM Image Using Domain Adaptation with Multimodal Data
FOURTEENTH INTERNATIONAL CONFERENCE ON MACHINE VISION (ICMV 2021)(2022)
摘要
There is a growing need for accurate depth measurements of on-chip structures, fueled by the ongoing size reduction of integrated circuits. However, current metrology methods do not offer a satisfactory solution. As Critical Dimension Scanning Electron Microscopes (CD-SEMs) are already being used for fast and local 2D imaging, it would be beneficial to leverage the 3D information hidden in these images. In this paper, we present a method that can predict depth maps from top-down CD-SEM images. We demonstrate that the proposed neural network architecture, together with a tailored training procedure, leads to accurate depth predictions on synthetic and real experimental data. Our training procedure includes a domain adaptation step, which utilizes data from a different modality (scatterometry), in the absence of ground-truth data in the experimental CD-SEM domain. The mean relative error of the proposed method is smaller than 6.2% on a contact-hole dataset of synthetic CD-SEM images with realistic noise levels. Furthermore, we show that the method performs well in terms of important semiconductor metrics. To the extent of our knowledge, we are the first to achieve accurate depth estimation results on experimental data, by combining data from the aforementioned modalities. We achieve a mean relative error smaller than 1%.
更多查看译文
关键词
CD-SEM images, scatterometry, optical critical dimension, 3D, monocular depth estimation, deep learning, domain adaptation, weakly supervised learning, CNN
AI 理解论文
溯源树
样例
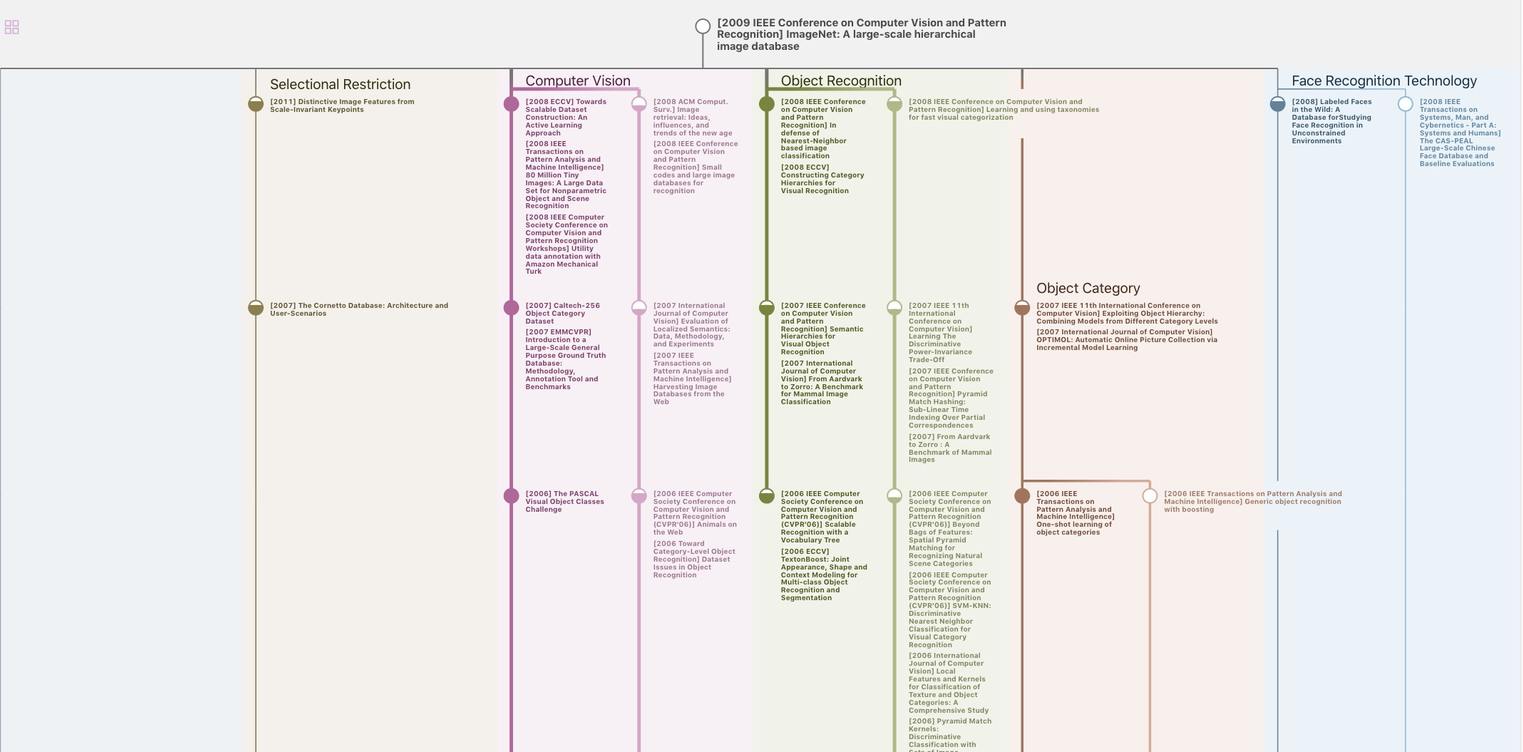
生成溯源树,研究论文发展脉络
Chat Paper
正在生成论文摘要