Integrating Single-Shot Fast Gradient Sign Method (FGSM) with Classical Image Processing Techniques for Generating Adversarial Attacks on Deep Learning Classifiers
FOURTEENTH INTERNATIONAL CONFERENCE ON MACHINE VISION (ICMV 2021)(2022)
摘要
Deep learning architectures have emerged as powerful function approximators in a broad spectrum of complex representation learning tasks, such as, computer vision, natural language processing and collaborative filtering. These architectures bear a high potential to learn the intrinsic structure of data and extract valuable insights. Despite the surge in the development of state-of-the-art intelligent systems using the deep neural networks (DNNs), these systems have found to be vulnerable to adversarial examples produced by adding a small-magnitude of perturbations. Such adversarial examples are adept at misleading the DNN classifiers. In the past, different attack strategies have been proposed to produce adversarial examples in the digital, physical, and transform domain, but the likelihood to generate perceptually realistic adversarial examples require more research efforts. In this paper, we present a novel approach to produce adversarial examples by combining the single-shot fast gradient sign method (FGSM) and spatial, as well as, transform domain image processing techniques. The resulted perturbations neutralize the impact of low-intensity based regions, thus, instilling the noise only in the selective high-intensity regions of the input image. While combining the customized perturbation with one-step FGSM perturbation in an un-targeted black-box attack scenario, the proposed approach successfully fools state-of-the-art DNN classifiers with 99% adversarial examples being misclassified on the ImageNet validation dataset.
更多查看译文
关键词
FGSM, Image Processing, Steganography, Perturbations, Adversarial Examples, Black-Box Attacks
AI 理解论文
溯源树
样例
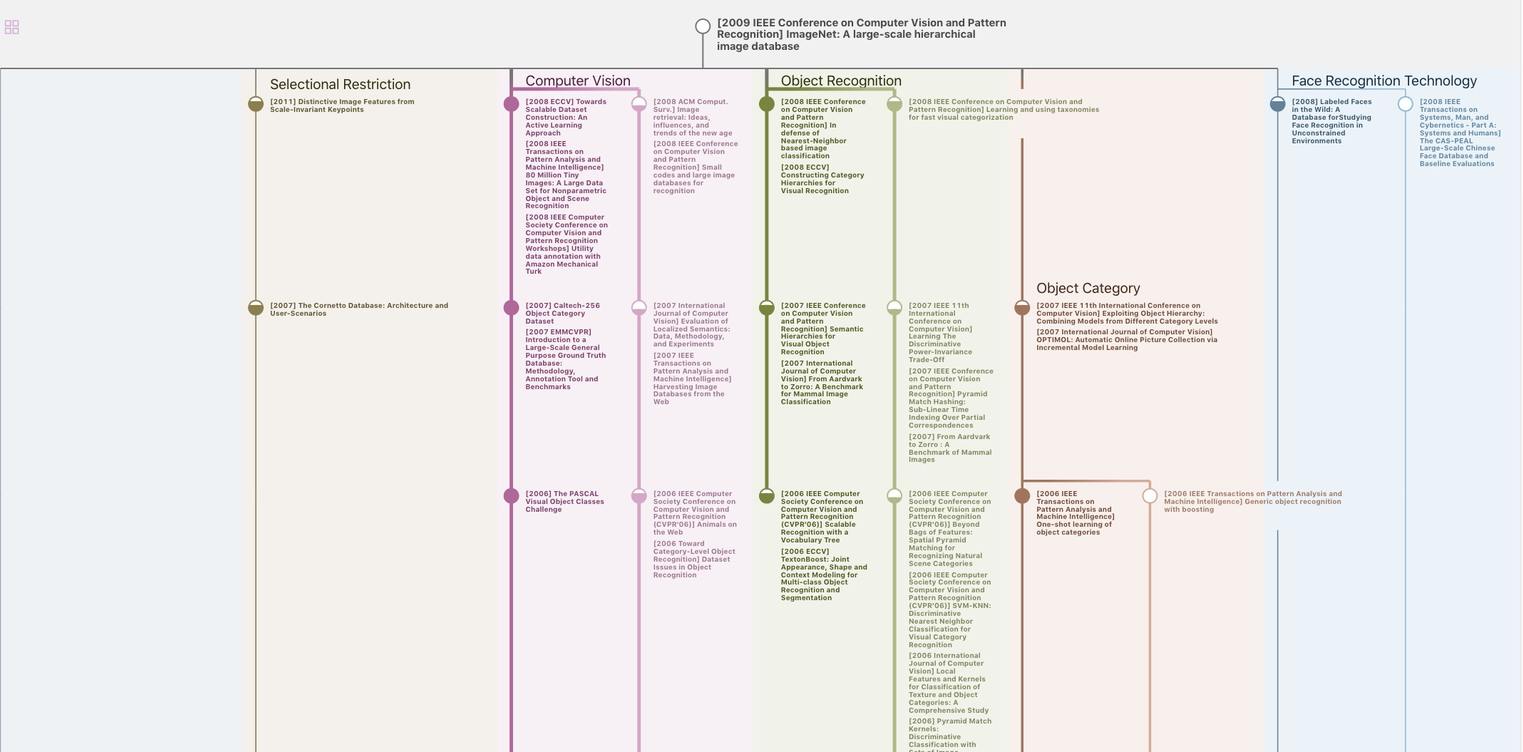
生成溯源树,研究论文发展脉络
Chat Paper
正在生成论文摘要