MANet: a multi-level aggregation network for semantic segmentation of high-resolution remote sensing images
INTERNATIONAL JOURNAL OF REMOTE SENSING(2022)
Abstract
With the continuous improvement of the segmentation effect for natural datasets, some studies have gradually been applied to high-resolution remote sensing images (HRRSIs). Due to a large amount of ground object information contained, even objects of the same type present the diversity and complexity of features in different periods or locations. The existing algorithms applied to semantic segmentation of remote sensing images are limited by the short-range context, and the high-resolution details, especially the edges, couldnot be fully recovered. Aiming at the problem, a multi-level aggregation network (MANet) is proposed. Firstly, the proposed global dependency module extracts deep global features by learning the interrelationships of all positions in the context, and filters redundant channel information as well. Secondly, MANet we proposed extends Multi-level Feature Aggregation Network by adding a simple and effective two-path feature refining module before each up-sample module to optimize the segmentation results. The two-path feature refining module uses two independent branches to obtain the features with different depths, which enriches the hierarchical structure of the network. Besides, it is combined with the subsequent up-sample module to effectively enhance MANet's ability to recover detailed information of HRRSI. Experimental results show that the methods proposed in the paper achieve competitive performance.
MoreTranslated text
Key words
High-Resolution,remote sensing images,Mosaic data enhancement,semantic segmentation,deep learning
AI Read Science
Must-Reading Tree
Example
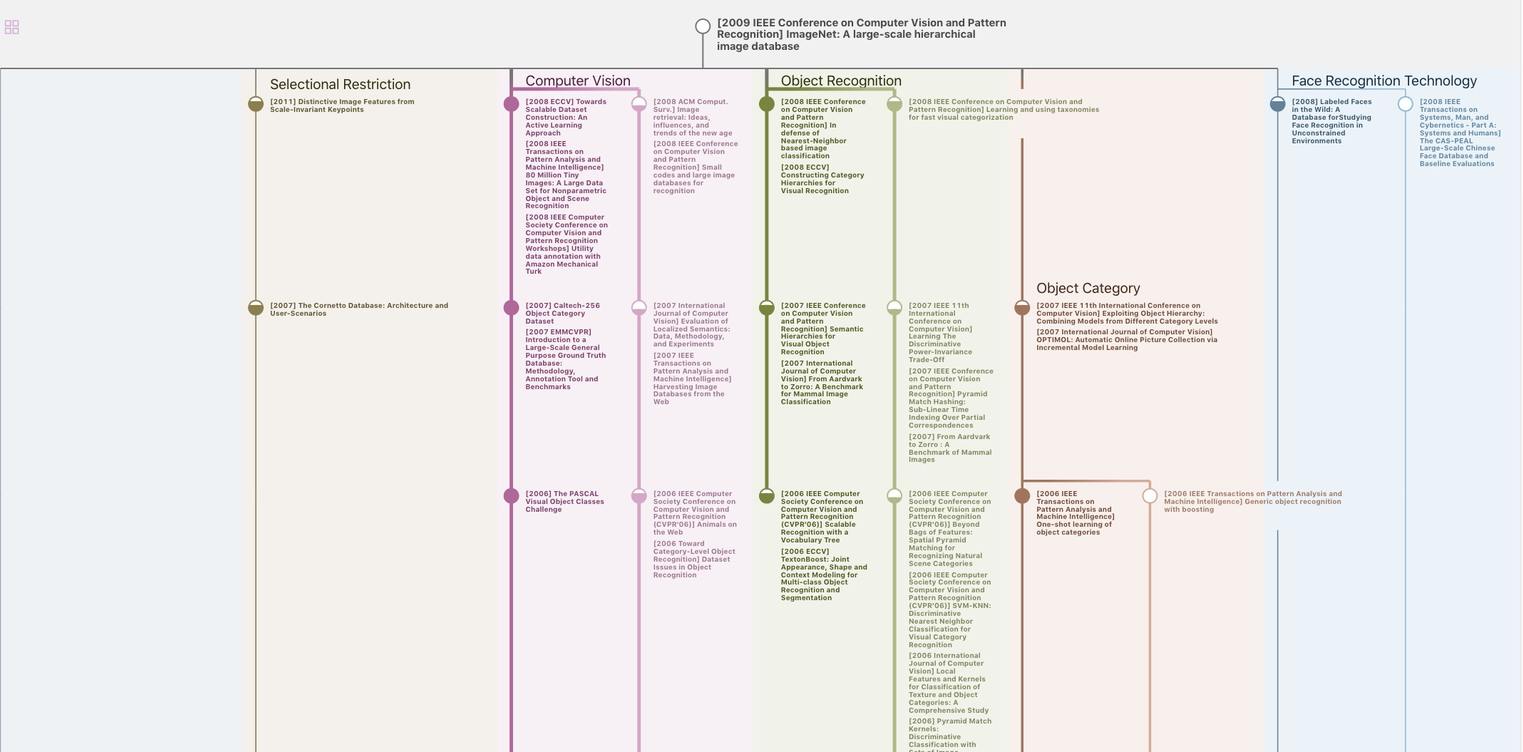
Generate MRT to find the research sequence of this paper
Chat Paper
Summary is being generated by the instructions you defined