Grid Load Forecasting Based on Dual Attention BiGRU and DILATE Loss Function
IEEE ACCESS(2022)
Abstract
To learn the deep relationship implicit in load data and improve the accuracy of load prediction, this paper presents a new Seq2seq framework based on dual attention and a bidirectional gated recurrent unit (BiGRU). The authors also introduce the Distortion Loss including Shape and Time (DILATE) loss function. Firstly, a dual attention mechanism is added to the Seq2seq architecture. The first layer of the attention mechanism enables the encoder to output multiple intermediate states, making the decoder more targeted when calculating the predicted values at different times. The second layer is an automatic attention mechanism, which reduces the possibility of errors accumulated during a long-time span prediction by calculating the internal correlation of the decoder output sequence data. Secondly, the DILATE loss function is introduced to improve the prediction lag problem caused by the mean square error (MSE) loss function. Finally, the proposed model is tested using power load data from a region in northern China. The simulation results show that the method proposed in this paper has a better prediction effect than LSTM, GRU and LS-SVR.
MoreTranslated text
Key words
Logic gates, Load modeling, Decoding, Predictive models, Time series analysis, Mathematical models, Load forecasting, Load forecasting, BiGRU, dual attention, seq2seq, DILATE
AI Read Science
Must-Reading Tree
Example
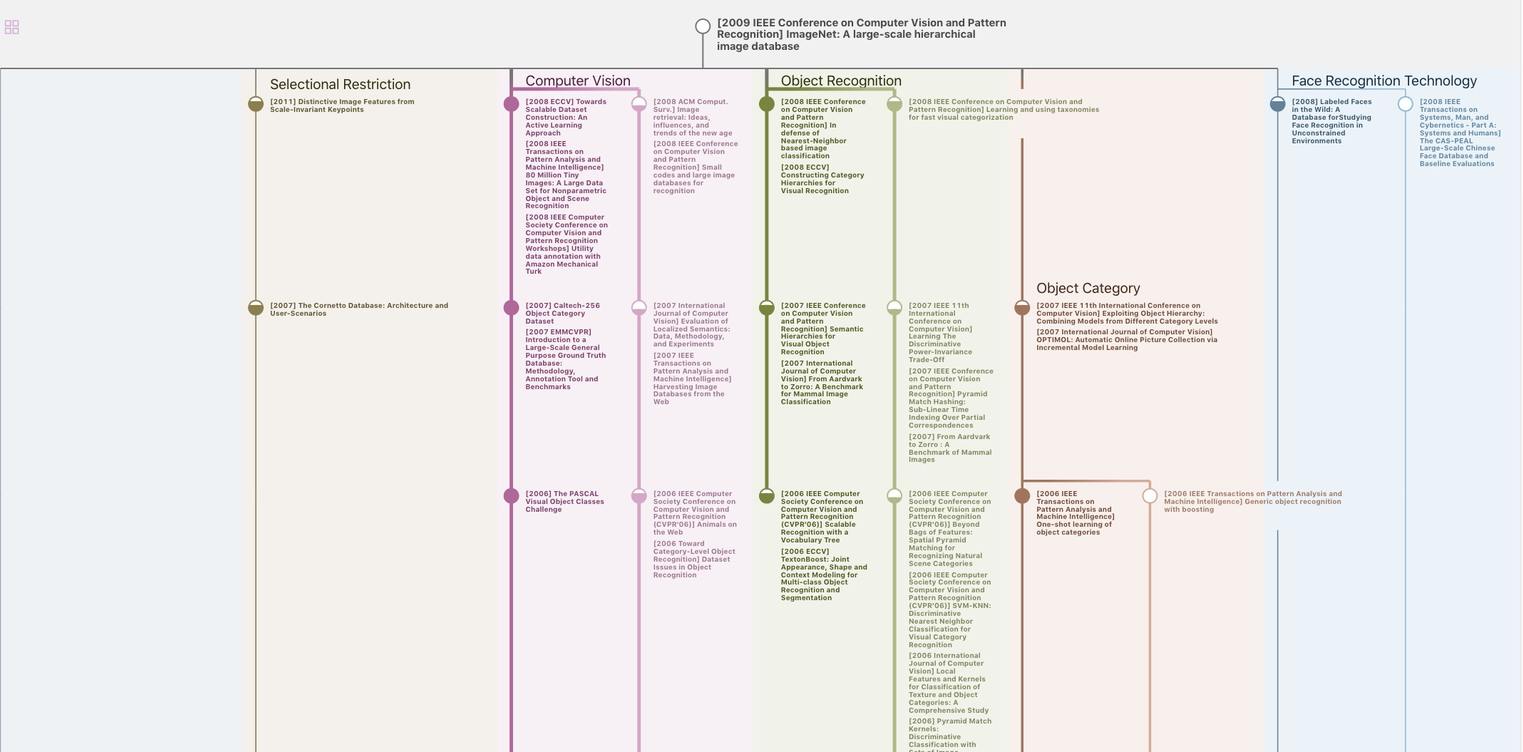
Generate MRT to find the research sequence of this paper
Chat Paper
Summary is being generated by the instructions you defined