Balancing Fairness and Energy Efficiency in SWIPT-Based D2D Networks: Deep Reinforcement Learning Based Approach
IEEE ACCESS(2022)
摘要
In this study, we propose a method to balance between user fairness and energy efficiency of users in the context of simultaneous wireless information and power transfer (SWIPT)-based device-to-device (D2D) networks. For this purpose, we build an optimization model which determines the subchannel allocation, transmit power level, and power splitting ratio of D2D users, with the purpose of maximizing the objective function which presents the trade-off level between the harvested energy and the average logarithmic data rate of users. To solve this problem, we employ deep reinforcement learning (DRL) which combines deep neural network (DNN) with reinforcement learning (RL). Despite the use of DRL, the dimension of the action space in our work is still very high because it should include subchannel allocation indicator, power splitting ratio, and transmit power of all D2D users. We therefore apply an interior point method to the output of the DNN in DRL to avoid the excess convergent time of DRL. Through the simulations, we compare the performance of our proposed algorithm to that of the conventional iterative algorithms; exhaustive search (ES) and gradient search (GS). Results show that the objective function value remains stable regardless of the change in the maximum transmission power. In addition, it is verified that varying the power splitting ratio has little effect on the system performance, which justifies using a constant power splitting ratio in SWIPT-based D2D networks. Furthermore, it is verified that the proposed DRL achieves near-global-optimal solution compared with conventional algorithms, with lower computational complexity.
更多查看译文
关键词
Device-to-device communication, Optimization, Resource management, Energy efficiency, Reinforcement learning, Deep learning, Interference, Energy efficiency, packet scheduling, D2D network, joint optimization, deep reinforcement learning
AI 理解论文
溯源树
样例
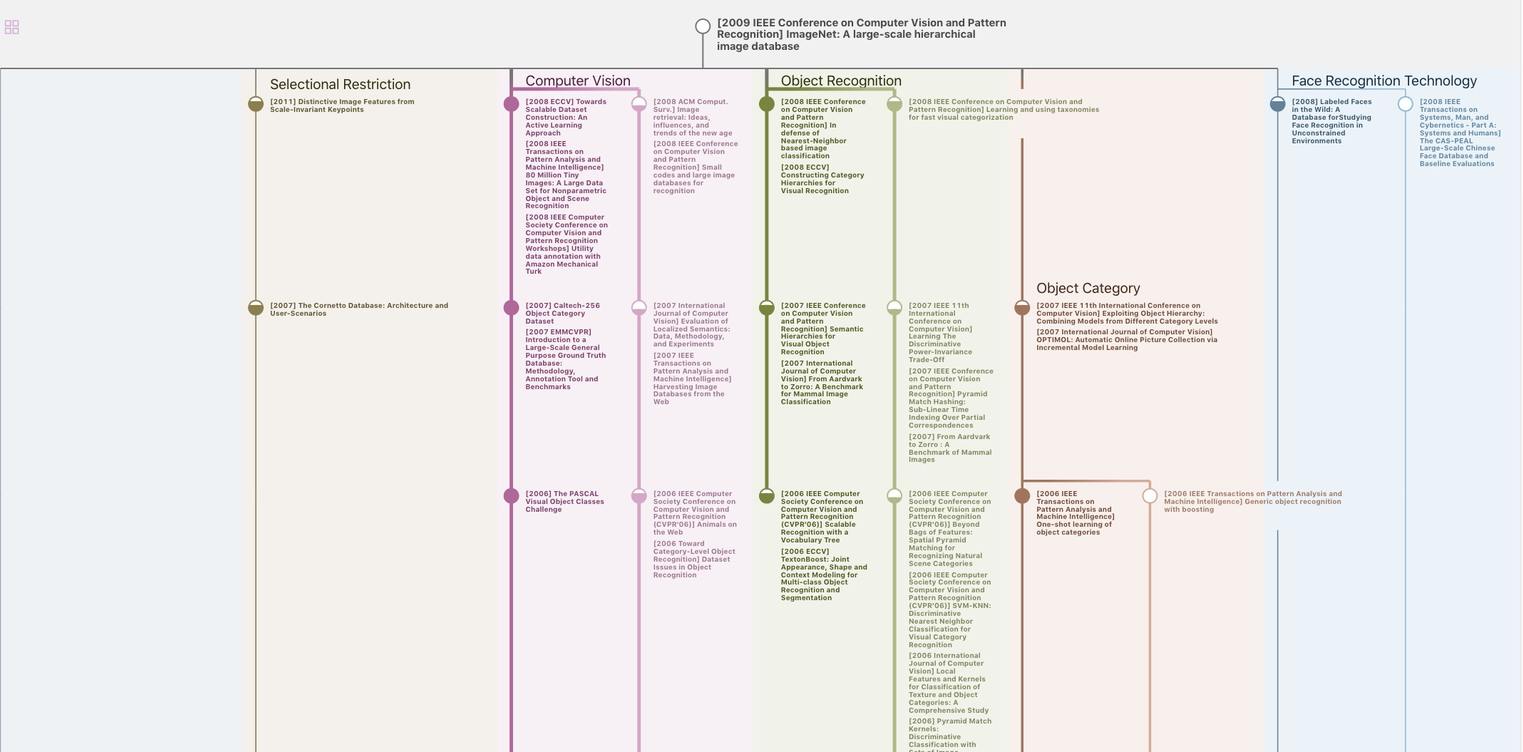
生成溯源树,研究论文发展脉络
Chat Paper
正在生成论文摘要