Combining low-cost noisy measurements with expensive accurate measurements to guide precision applications
PRECISION AGRICULTURE(2022)
摘要
Precision agriculture requires many local measurements. Sometimes two measurements are available: a low-cost noisy measurement and an accurate expensive one. For example, soil testing in a laboratory is expensive and accurate. On-the-go pH meters are available, but they are not as accurate. The question addressed here is what is the best way to combine these measures to guide precision applications? The first step is to estimate the joint spatial distribution of the two measures. The joint distribution is estimated using Bayesian Kriging since it can consider the information when the measures are spatially autocorrelated. The second step is to determine the economic optimum of how many of each measure to use. This study obtained the ratio of expensive and accurate measurements by maximizing the expected net present value using Bayesian Decision Theory and a grid search procedure. To demonstrate the method, a harmonization process that uses no spatial information was compared with Bayesian Kriging using Monte Carlo data. A wheat production example was used to parameterize the Monte Carlo simulation. Soil pH lab sampling and on-the-go soil pH sensors were simulated as the two different measurements for soil mapping in wheat fields. Bayesian Kriging led to more accurate soil mapping and a higher expected net present value.
更多查看译文
关键词
Bayesian Kriging, Precision agriculture, Soil mapping, Soil pH
AI 理解论文
溯源树
样例
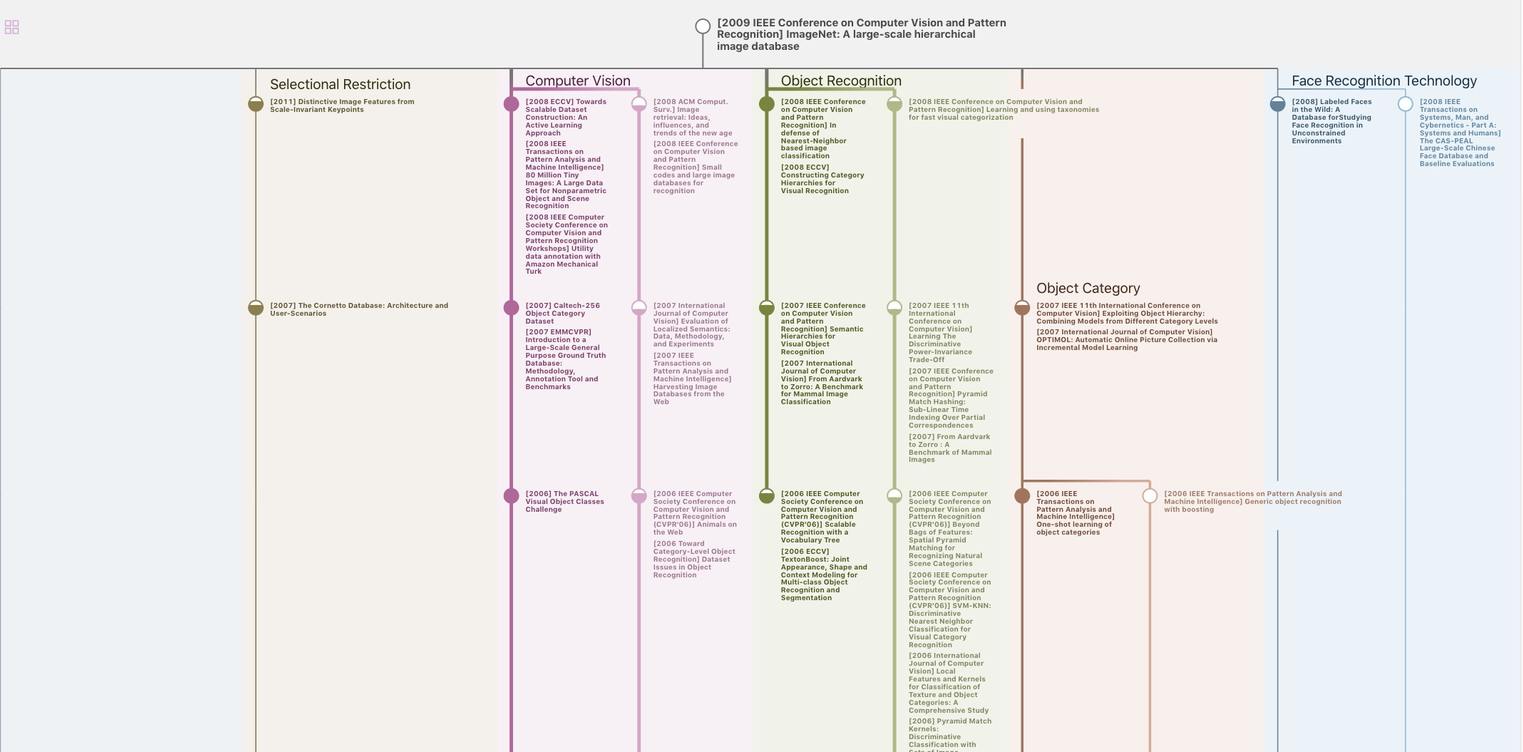
生成溯源树,研究论文发展脉络
Chat Paper
正在生成论文摘要