LiDAR SLAM Based Multivehicle Cooperative Localization Using Iterated Split CIF
IEEE Transactions on Intelligent Transportation Systems(2022)
摘要
High-precision localization in an unknown environment is the fundamental requirement of autonomous vehicle for safe advanced driving. Cooperative localization has obvious advantages in precision, fault tolerance, and flexibility compared with single-vehicle localization, which estimates the vehicle pose via fusing the multiple sources data from sensors and extra shared neighbor vehicle information available when multiple vehicles operate simultaneously. For cooperative localization, there are more than one type of error data sources that degenerate the state estimation need to be considered, such as inter-estimation correlation and innovation, observation outliers, which may exist simultaneously. Previous works usually evaluate performance under one of the error types at one time, or they do not perform well under some extreme situations where both of the above-mentioned correlation and outliers exist. This paper presents an accurate and robust iterated split covariance intersection filter (Iterated Split CIF) based cooperative localization strategy with a decentralized framework, which can ensure the performance when data sources of various error types exist at the same time. In addition, we adopt an effective point cloud registration method to obtain the cooperative relative pose estimation using mutually shared information from neighbor vehicles. A CARLA simulator based comparative study demonstrates the potential and advantage of the proposed multi-vehicle cooperative localization using Iterated Split CIF in terms of accuracy, robustness and efficiency.
更多查看译文
关键词
Iterated split CIF,cooperative localization,data fusion,inter-vehicle relative pose,autonomous vehicle
AI 理解论文
溯源树
样例
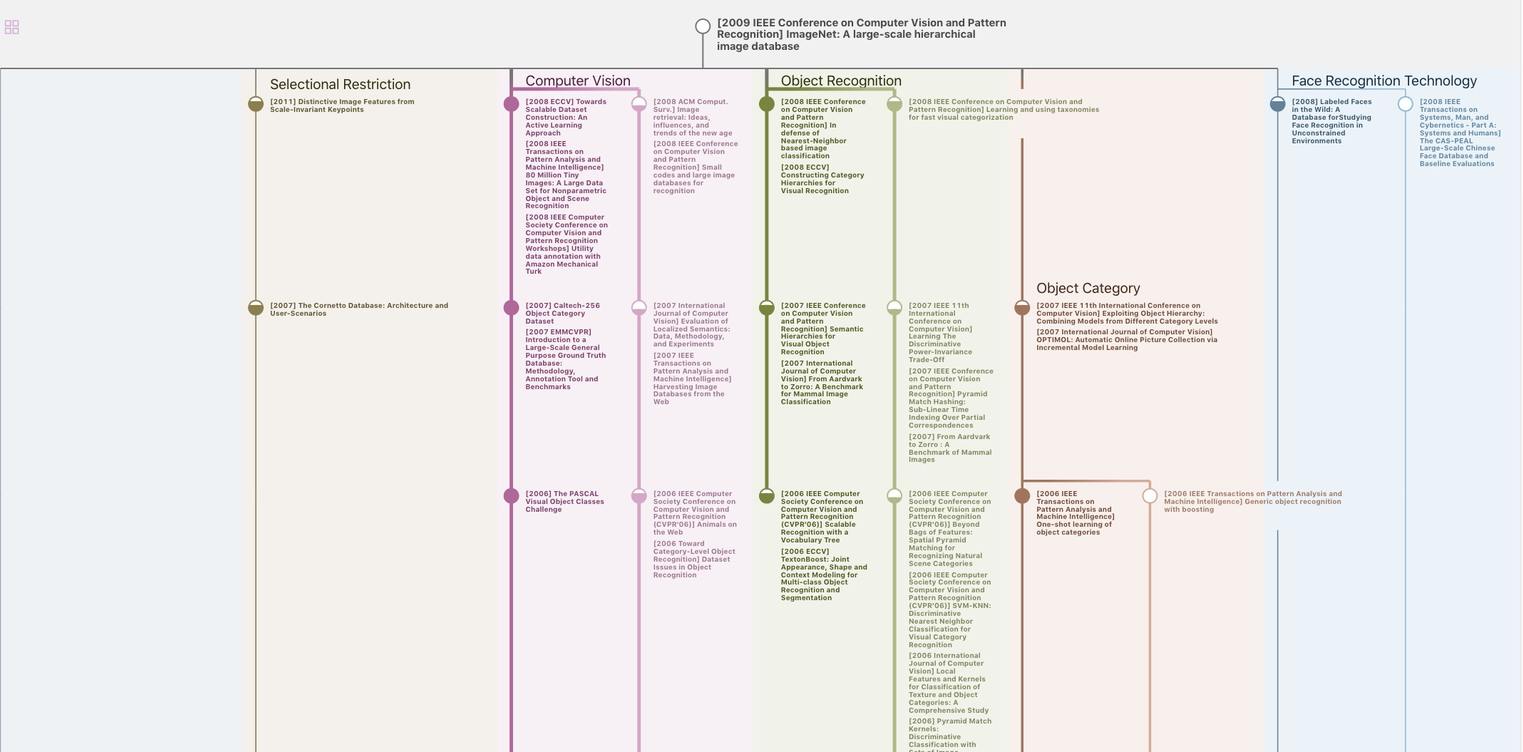
生成溯源树,研究论文发展脉络
Chat Paper
正在生成论文摘要