Modeling Average Grain Velocity for Rectangular Channel Using Soft Computing Techniques
WATER(2022)
摘要
This study was undertaken with the primary objective of modeling grain velocity based on experimental data obtained under the controlled conditions of a laboratory using a rectangular hydraulic tilting channel. Soft computing approaches, i.e., support vector machine (SVM), artificial neural network (ANN), and multiple linear regression (MLR), were applied to simulate grain velocity using four input variables; shear velocity, exposed area to base area ratio (EATBAR), relative depth, and sediment particle weight. Quantitative performance evaluation of predicted values was performed with the help of three different standard statistical indices, such as the root mean square error (RMSE), Pearson's correlation coefficient (PCC), and Wilmot index (WI). The results during the testing phase revealed that the SVM model has RMSE (m/s), PCC, and WI values obtained as 0.1195, 0.8877, and 0.7243, respectively, providing more accurate predictions than the MLR and ANN models during the testing phase.
更多查看译文
关键词
grain velocity, sediment transportation, shear velocity, ANN, SVM
AI 理解论文
溯源树
样例
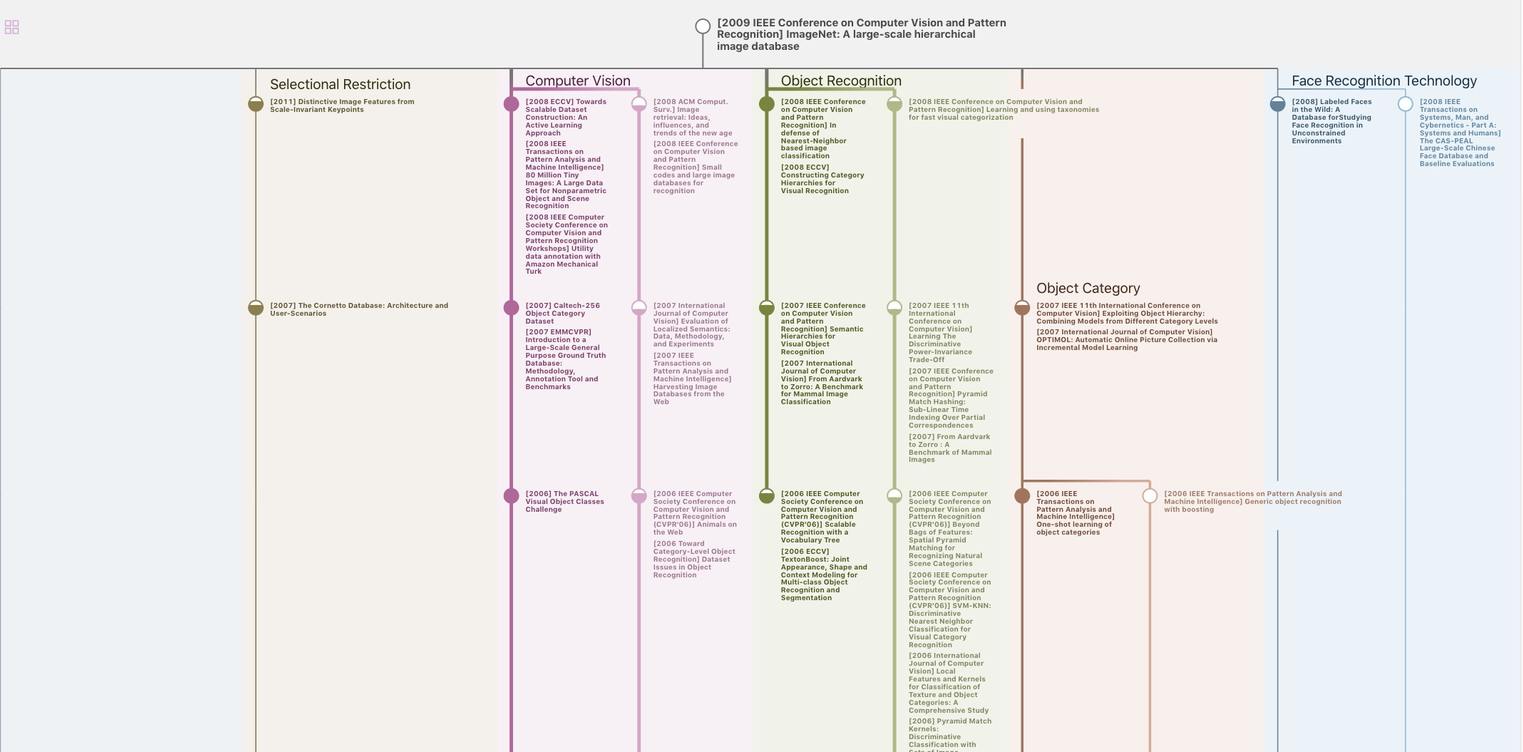
生成溯源树,研究论文发展脉络
Chat Paper
正在生成论文摘要