Short-Term Traffic Speed Forecasting Model for a Parallel Multi-Lane Arterial Road Using GPS-Monitored Data Based on Deep Learning Approach
SUSTAINABILITY(2022)
摘要
Traffic speed forecasting in the short term is one of the most critical parts of any intelligent transportation system (ITS). Accurate speed forecasting can support travelers' route choices, traffic guidance, and traffic control. This study proposes a deep learning approach using long short-term memory (LSTM) network with tuning hyper-parameters to forecast short-term traffic speed on an arterial parallel multi-lane road in a developing country such as Vietnam. The challenge of mishandling the location data of vehicles on small and adjacent multi-lane roads will be addressed in this study. To test the accuracy of the proposed forecasting model, its application is illustrated using historical voyage GPS-monitored data on the Le Hong Phong urban arterial road in Haiphong city of Vietnam. The results indicate that in comparison with other models (e.g., traditional models and convolutional neural network), the best performance in terms of root mean square error (RMSE), mean absolute error (MAE), and median absolute error (MDAE) is obtained by using the proposed model.
更多查看译文
关键词
deep learning approach, LSTM network, short-term traffic speed forecasting
AI 理解论文
溯源树
样例
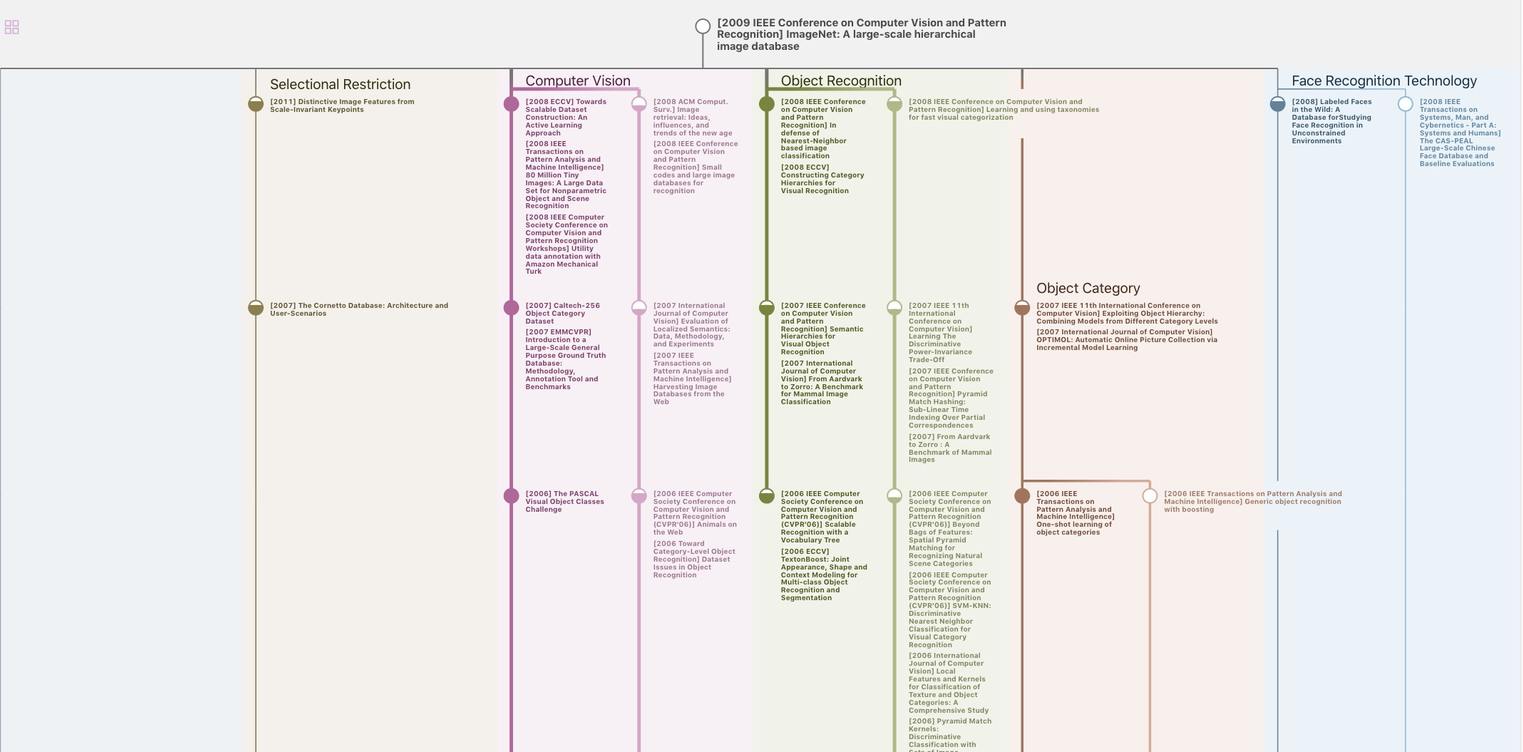
生成溯源树,研究论文发展脉络
Chat Paper
正在生成论文摘要