Targeted projection pursuit similarity based attribute selection for academic performance prediction
CONCURRENCY AND COMPUTATION-PRACTICE & EXPERIENCE(2022)
摘要
The performance of student in the academic field reveals the consideration over researchers to enhance student's weakness. With the consumption of high potential factors from the dataset, accurate student performance prediction is carried out. Targeted projection pursuit similarity based attribute selection (TPPS-AS) technique is designed to improve the student academic performance prediction. TPPS is a machine learning technique that observes the given input. The relevant attributes from the multidimensional space is determined by TPPS. Several research works were recognized recently to conclude the high potential factors for observing student academic performances. A novel technique is designed in this research work to improve the student academic performance prediction in a taken dataset by choosing more relevant attributes. To detect the student academic performance with better accuracy and lesser time, proposed TPPS-AS technique is employed. The performance of student academic performance prediction is improved by TPPS-AS technique through the attribute selection with higher accuracy. With this proposed technique, prediction accuracy of the student academic performance is increased after the relevant attributes selection process.
更多查看译文
关键词
academic prediction, attribute selection, Jaccard similarity coefficient, performance metrices, TPPS-AS
AI 理解论文
溯源树
样例
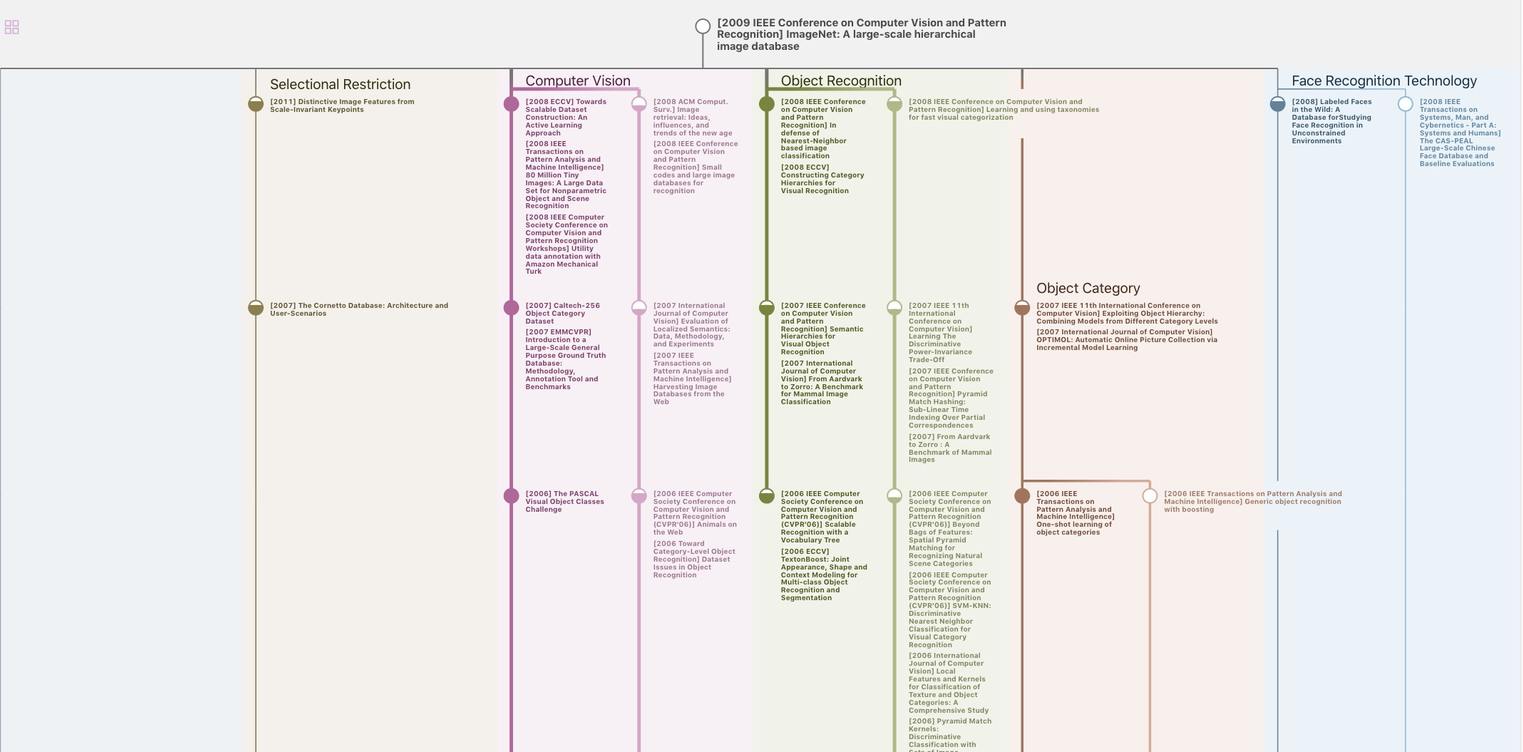
生成溯源树,研究论文发展脉络
Chat Paper
正在生成论文摘要