Condition Classification of Fibre Ropes during Cyclic Bend over Sheave testing Using Machine Learning
INTERNATIONAL JOURNAL OF PROGNOSTICS AND HEALTH MANAGEMENT(2022)
摘要
Fibre ropes have been shown to be a viable alternative to steel wire rope for offshore lifting operations. Visual inspection remains a common method of fibre rope condition monitoring and has the potential to be further automated by machine learning. This would provide a valuable aid to current inspection frameworks to make more accurate decisions on recertification or retirement of fibre ropes in operational use. Three different machine learning algorithms: decision tree, random forest and support vector machine are compared to classical statistical approaches such as logistic regression, k-nearest neighbours and Naive-Bayes for condition classification for fibre ropes under cyclic-bend-over-sheave (CBOS) testing. By measuring the rope global elongation throughout the CBOS tests, a binary classification system has been used to label recorded samples as healthy or close to rupture. Predictions are made on one rope through leave-one-out cross validation. The models are then assessed through calculating the accuracy, probability of detection, probability of false alarm and Matthew's Correlation Coefficient, and ranked based on the results. The results show that both machine learning and classical statistical methods are effective options for condition classification of fibre ropes under CBOS regimes. Typical values for Matthews Correlation Coefficient (MCC) were shown to exceed 0.8 for the best performing methods.
更多查看译文
关键词
fibre rope,condition monitoring,machine learning,decision trees,random forest,support vector machines
AI 理解论文
溯源树
样例
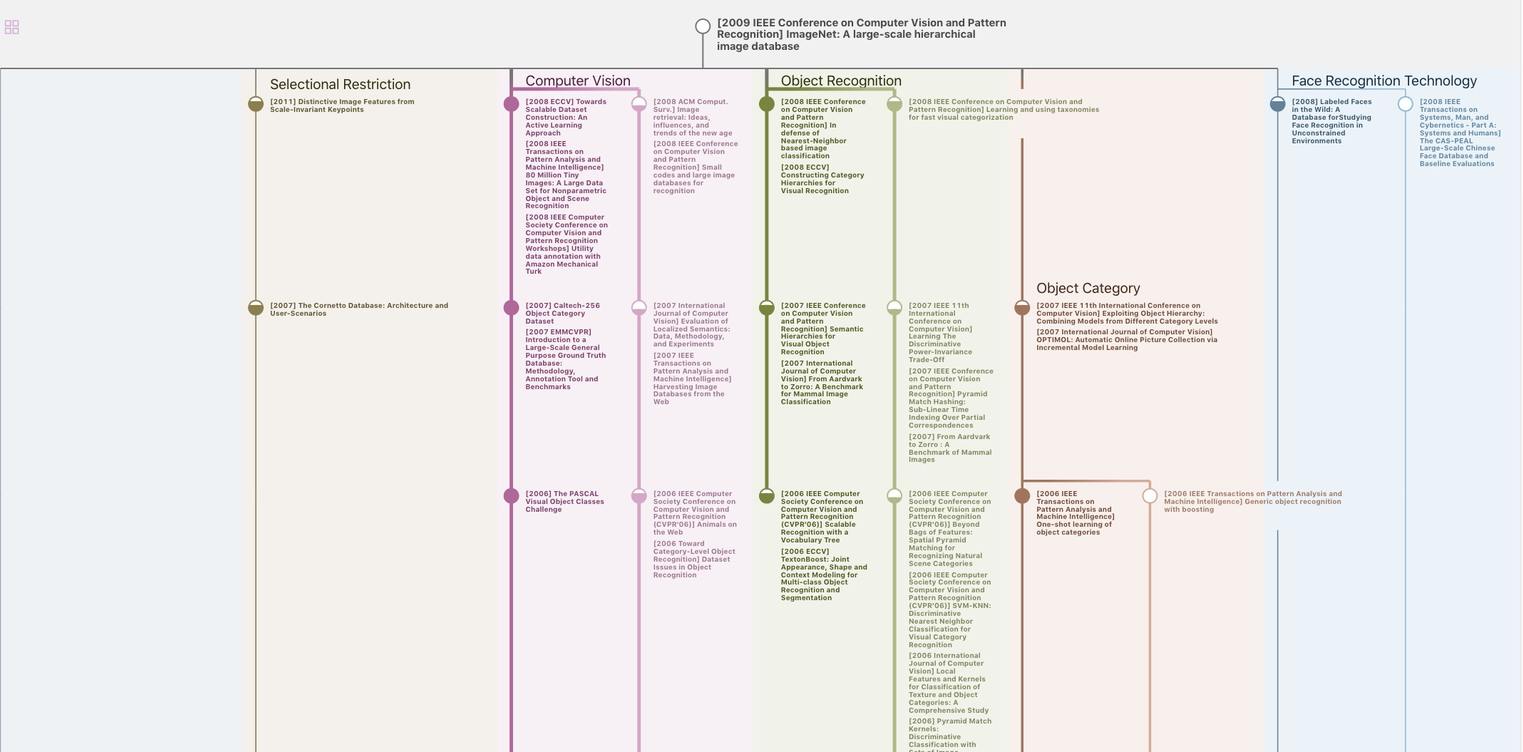
生成溯源树,研究论文发展脉络
Chat Paper
正在生成论文摘要