Portfolio Optimization Via Online Gradient Descent and Risk Control
COMPUTATIONAL ECONOMICS(2022)
摘要
Since Markowitz’s initial contribution in 1952, portfolio selection has undoubtedly been one of the most challenging topics in finance. The development of online optimization techniques indicates that dynamic learning algorithms are an effective approach to portfolio construction, although they do not evaluate the risk associated with each investment decision. In this work, the performance of the well-known Online Gradient Descent (OGD) algorithm is evaluated in comparison with a proposed approach that incorporates portfolio risk using β control of portfolio assets modeled with the CAPM strategy and considering a time-varying β that follows a random walk. Thus, the traditional OGD algorithm and the OGD with β constraints are compared with the Uniform Constant Rebalanced Portfolio (UCRP) and two specific indexes for the Brazilian market, consisting of small caps and the assets belonging to the Bovespa index. The experiments have shown that β control, combined with an appropriate definition of the β interval by the investor, is an efficient strategy, regardless of market periods with gains or losses. Moreover, time-varying β has been shown to be an efficient measure to force the desired correlation with the market and also to reduce the volatility of the portfolio, especially during hazardous bear markets.
更多查看译文
关键词
Portfolio optimization, Online gradient descent, CAPM model
AI 理解论文
溯源树
样例
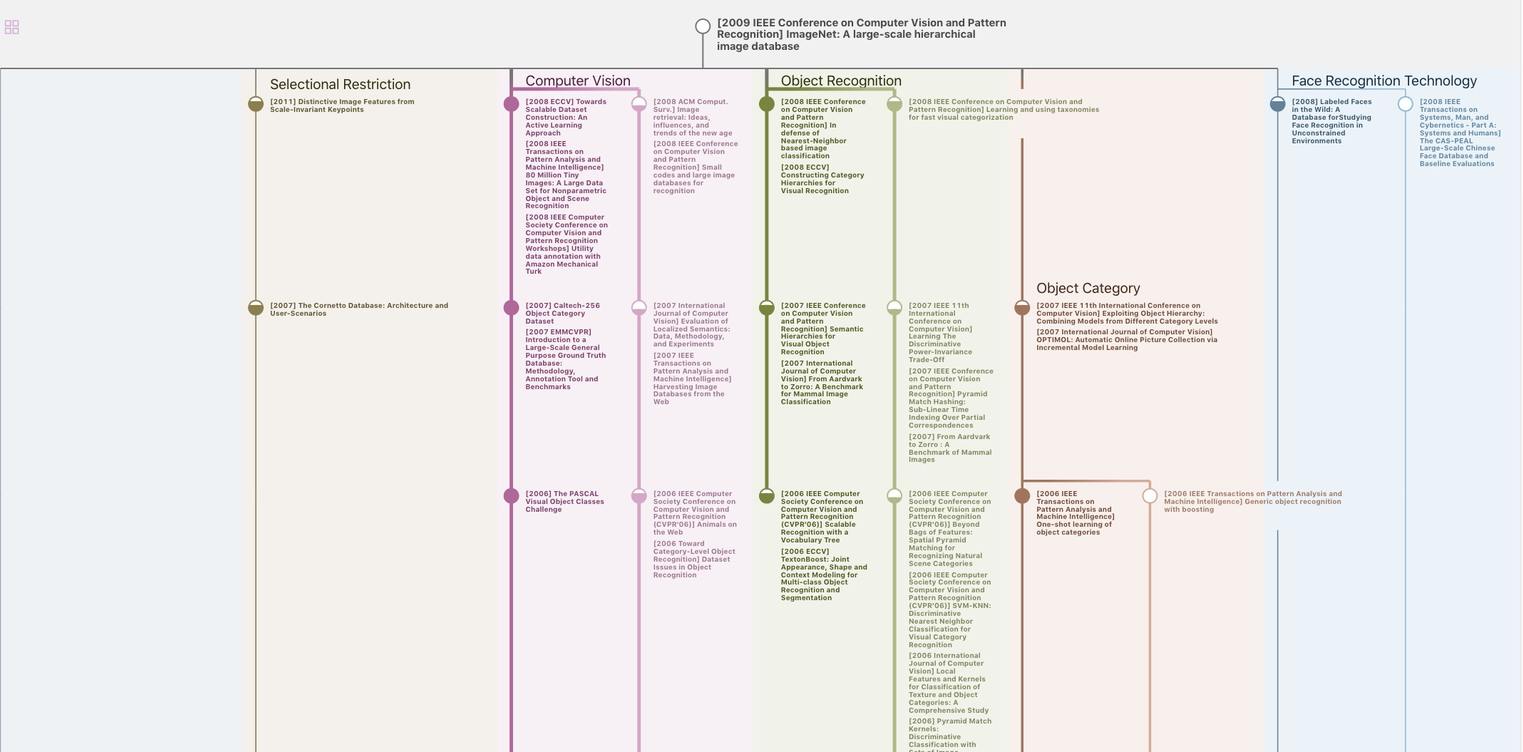
生成溯源树,研究论文发展脉络
Chat Paper
正在生成论文摘要