Suitability of different multivariate analysis methods for monitoring leaf N accumulation in winter wheat using in situ hyperspectral data
COMPUTERS AND ELECTRONICS IN AGRICULTURE(2022)
摘要
Leaf nitrogen accumulation (LNA) is an important indicator of crop growth, and the real-time and nondestructive estimation of LNA by hyperspectral remote sensing is crucial for crop N management. In this study, five field experiments were conducted over four successive years at three ecological sites (Xinyang, Zhengzhou and Zhoukou) in Henan, China, incorporating different N amounts and wheat varieties. The canopy spectral data were obtained by a ground-based spectrometer, and the original spectral reflectance (R) was transformed using continuum removal (CR), first-derivative reflectance (FDR) and inverse-log reflectance (log (1/R)) methods. The effective bands of the above four transformed reflectance data were selected by the variable importance in projection (VIP) scores of the partial least square regression method (PLSR). Then, four multivariate regression methods (PLSR, stepwise multiple linear regression (SMLR), extreme learning machine (ELM) and support vector machine (SVM)) were used to develop an optimal forecasting model for LNA in winter wheat. The results show that FDR spectral data had the best comprehensive performance and were a better method for spectral data preprocessing. In the comparison of different nonparametric algorithms, the SVM model performed the best using the full wavelengths, in which R(cal/val)( )(2)and RMSEcal/val were 0.927-0.963/0.899-0.925 and 0.725-1.066/ 1.161-1.331 g m(-2), respectively. The characteristic bands of FDR were mainly distributed in the visible light and red edge regions (445, 501, 546, 683, 747 and 771 nm, respectively), and these effective bands were consistent under different field conditions. The SVM regression method using the effective wavelengths of FDR exhibited excellent performance for LNA prediction with R-val(2) = 0.875 and RMSEval = 1.294. In addition, the FDR-PLSRSVM model produced satisfactory inversion results under different field conditions. The accuracy of the filling period was better than that of the reviving-anthesis growth period, which might be due to diminished N dilution and stable canopy structure during the reproductive stage. R2val and RMSEval of the FDR-PLSR-SVM model during the filling period were 0.914 and 1.156 g m(-2) in Xinyang, 0.825 and 1.542 g m(-2) in Zhengzhou and 0.796 and 1.902 g m(-2) in Zhoukou, respectively. Considering the advantages of simple and efficient computer calculations, the FDR-PLSR-SVM algorithm is strongly recommended to predict the LNA in winter wheat. This study provides a reference basis for the selection of effective wavelengths for N nutrition diagnosis by unmanned aerial vehicles and satellite remote sensing in the future.
更多查看译文
关键词
Winter wheat, Hyperspectral reflectance, Leaf nitrogen accumulation, Spectral transformation techniques, Multivariate analysis
AI 理解论文
溯源树
样例
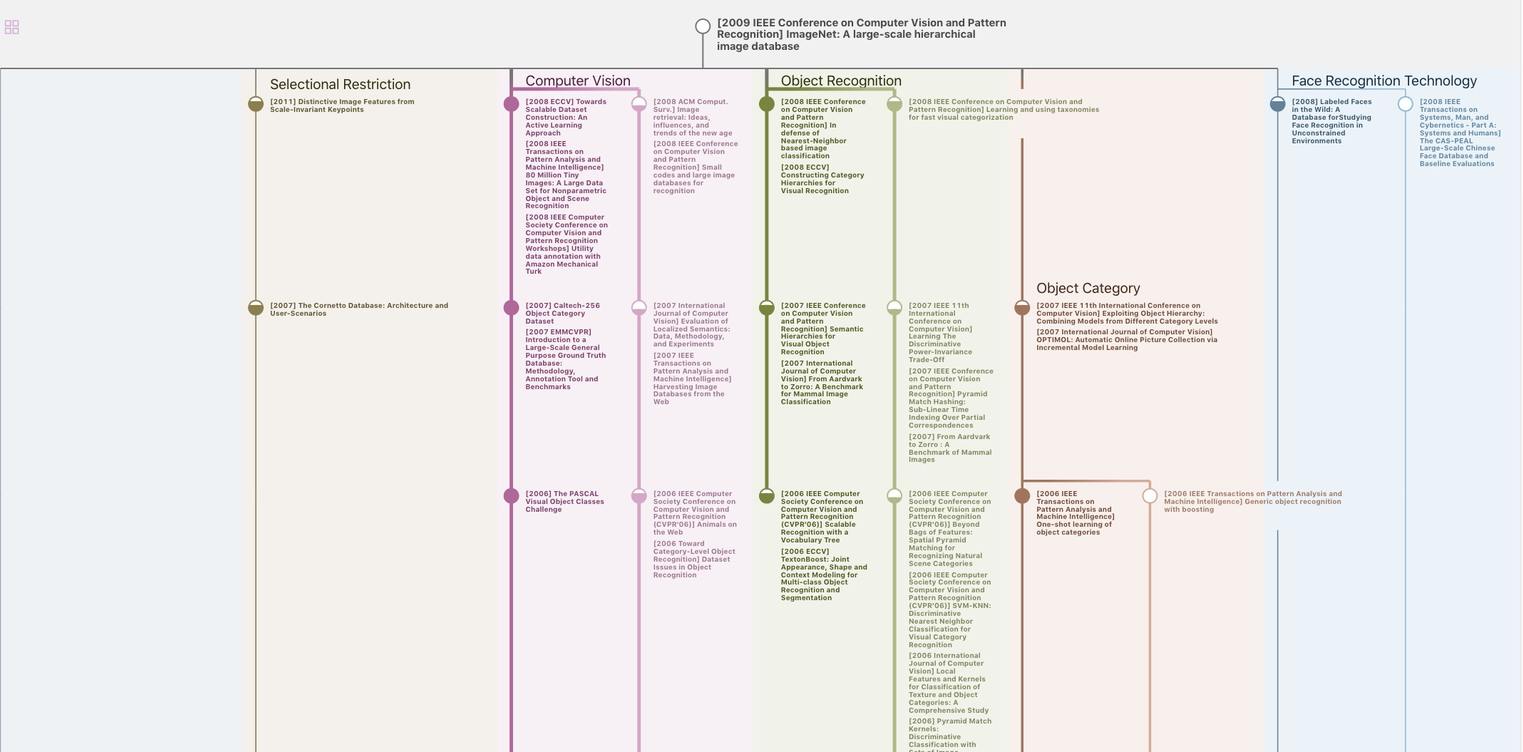
生成溯源树,研究论文发展脉络
Chat Paper
正在生成论文摘要