Improving Wheat Yield Prediction Accuracy Using LSTM-RF Framework Based on UAV Thermal Infrared and Multispectral Imagery
AGRICULTURE-BASEL(2022)
摘要
Yield prediction is of great significance in agricultural production. Remote sensing technology based on unmanned aerial vehicles (UAVs) offers the capacity of non-intrusive crop yield prediction with low cost and high throughput. In this study, a winter wheat field experiment with three levels of irrigation (T1 = 240 mm, T2 = 190 mm, T3 = 145 mm) was conducted in Henan province. Multispectral vegetation indices (VIs) and canopy water stress indices (CWSI) were obtained using an UAV equipped with multispectral and thermal infrared cameras. A framework combining a long short-term memory neural network and random forest (LSTM-RF) was proposed for predicting wheat yield using VIs and CWSI from multi-growth stages as predictors. Validation results showed that the R-2 of 0.61 and the RMSE value of 878.98 kg/ha was achieved in predicting grain yield using LSTM. LSTM-RF model obtained better prediction results compared to the LSTM with R-2 of 0.78 and RMSE of 684.1 kg/ha, which is equivalent to a 22% reduction in RMSE. The results showed that LSTM-RF considered both the time-series characteristics of the winter wheat growth process and the non-linear characteristics between remote sensing data and crop yield data, providing an alternative for accurate yield prediction in modern agricultural management.
更多查看译文
关键词
UAV, wheat yield, multispectral, thermal infrared, long short-term memory network
AI 理解论文
溯源树
样例
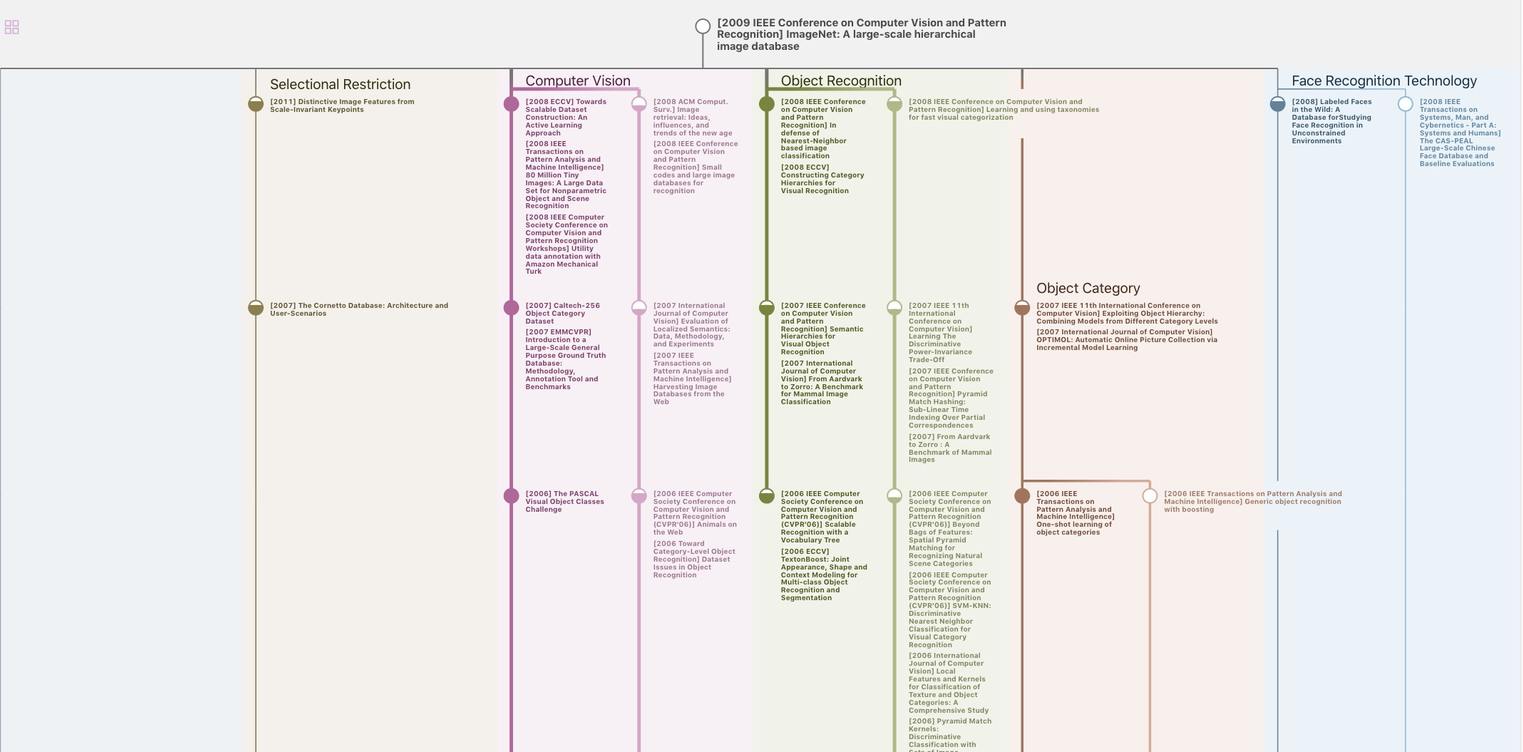
生成溯源树,研究论文发展脉络
Chat Paper
正在生成论文摘要