Statistical Control Requires Causal Justification
ADVANCES IN METHODS AND PRACTICES IN PSYCHOLOGICAL SCIENCE(2022)
Key words
statistical control,causality,confounder,mediator,collider,open materials
AI Read Science
Must-Reading Tree
Example
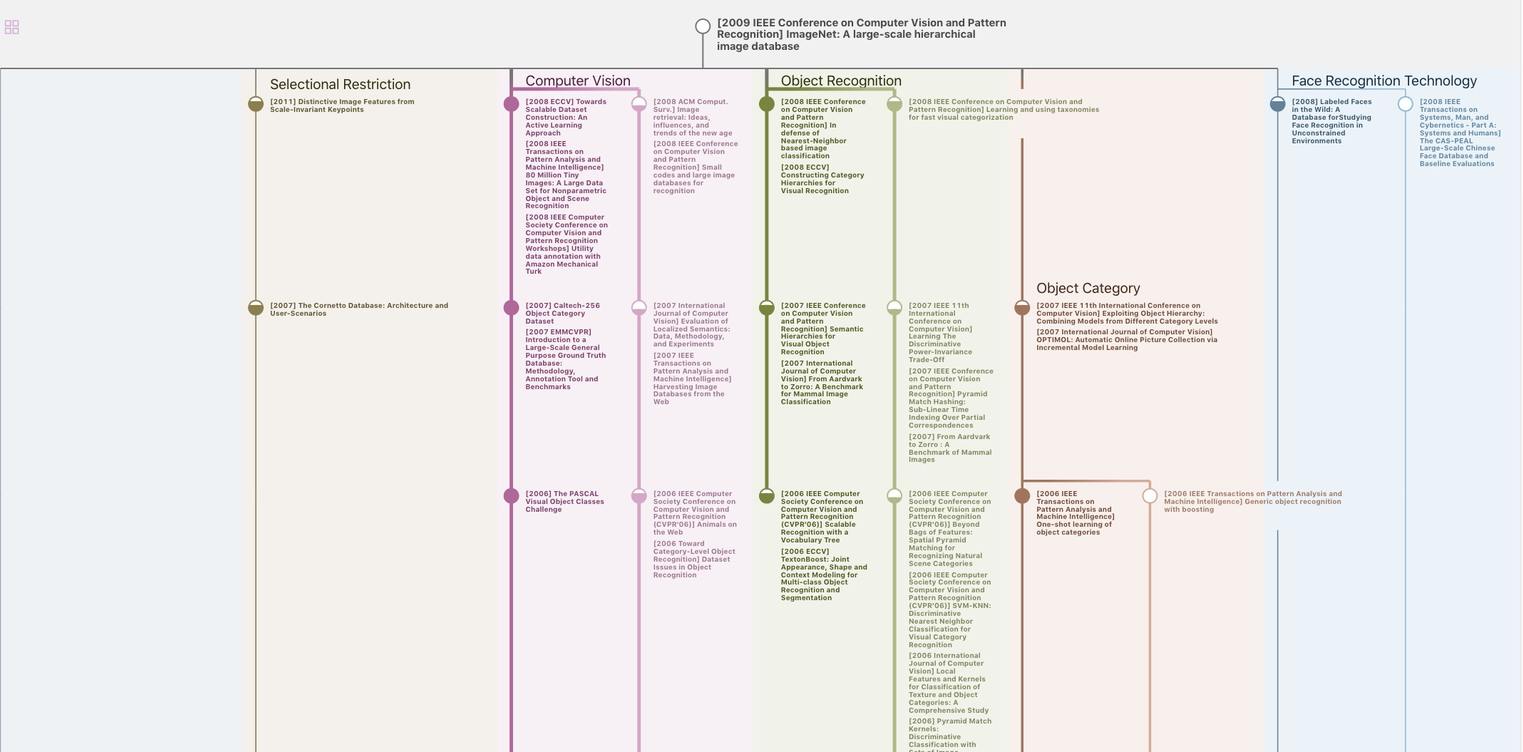
Generate MRT to find the research sequence of this paper
Chat Paper
Summary is being generated by the instructions you defined