Mechanical health indicator construction and similarity remaining useful life prediction based on natural language processing model
MEASUREMENT SCIENCE AND TECHNOLOGY(2022)
Abstract
Remaining useful life (RUL) prediction, as an essential task for prediction and health management, can improve the reliability of degradation systems. In this paper, multi-dimensional mechanical monitoring readings are treated as natural language sequences and inputted to a natural language processing model, Transformer, to extract semantic compression vectors. The health indicators (HIs) are constructed from the changes of compressed semantic vectors during the mechanical system operation, reflecting the mechanical health status at each monitoring point. Subsequently, combined with the similarity matching method, the RUL prediction is achieved by weighted summation. Transformer mainly consists of attention layers and residual connection layers, which contain no recurrence and convolution. The attention layer can highlight the critical time step information during the HI construction. Moreover, the output of different time steps can be obtained simultaneously in a parallel computation manner. The proposed scheme is conducted on two publicly available datasets for experimental and evaluation metrics comparison. The experimental results show that the proposed scheme can achieve competitive prediction results, with some of the metrics reaching the highest. An autoencoder only containing attention mechanism can effectively extract degradation feature representations for the adaptive construction of unsupervised HIs.
MoreTranslated text
Key words
remaining useful life, transformer, multi-head attention, health indicator
AI Read Science
Must-Reading Tree
Example
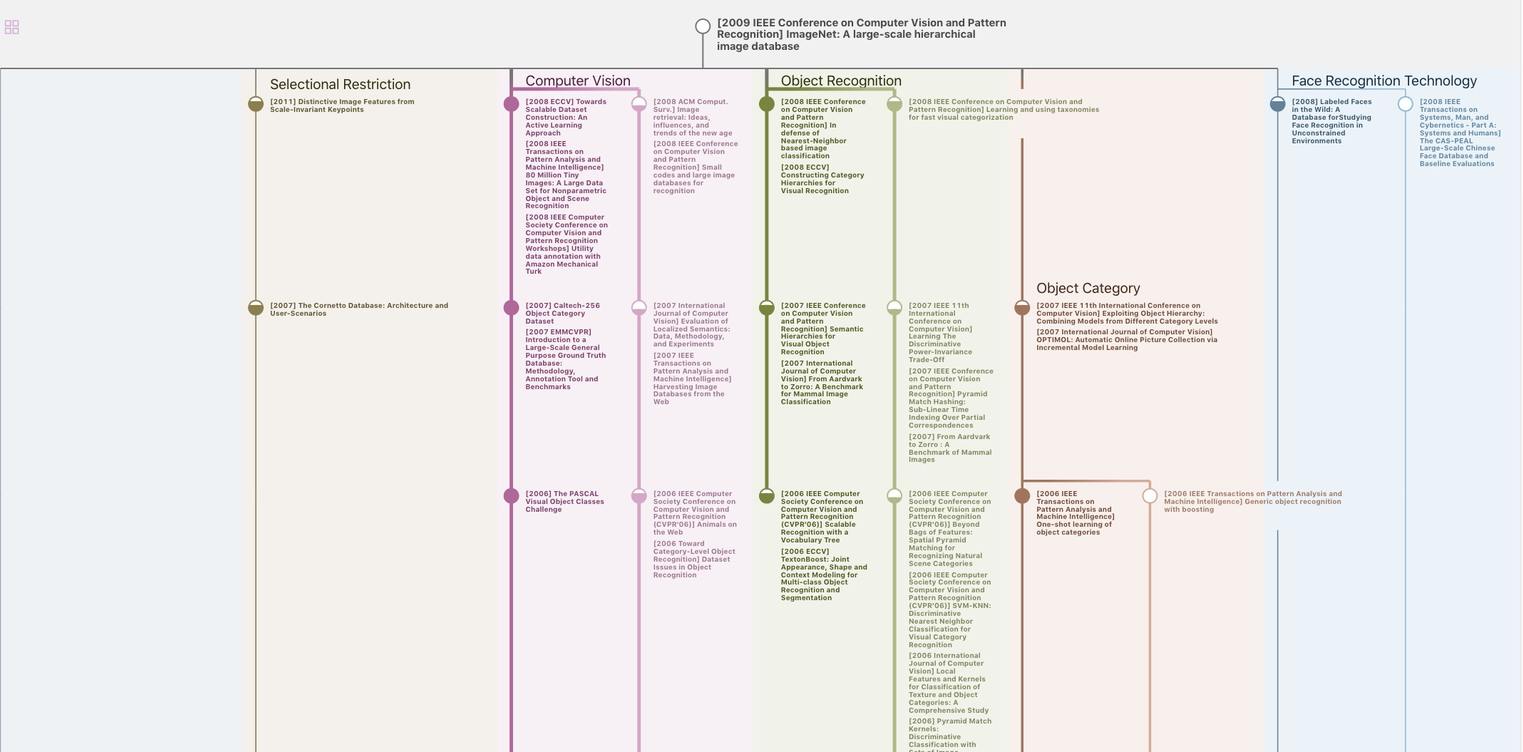
Generate MRT to find the research sequence of this paper
Chat Paper
Summary is being generated by the instructions you defined