Accurate Estimation of Tensile Strength of 3D Printed Parts Using Machine Learning Algorithms
PROCESSES(2022)
摘要
Manufacturing processes need optimization. Three-dimensional (3D) printing is not an exception. Consequently, 3D printing process parameters must be accurately calibrated to fabricate objects with desired properties irrespective of their field of application. One of the desired properties of a 3D printed object is its tensile strength. Without predictive models, optimizing the 3D printing process for achieving the desired tensile strength can be a tedious and expensive exercise. This study compares the effectiveness of the following five predictive models (i.e., machine learning algorithms) used to estimate the tensile strength of 3D printed objects: (1) linear regression, (2) random forest regression, (3) AdaBoost regression, (4) gradient boosting regression, and (5) XGBoost regression. First, all the machine learning models are tuned for optimal hyperparameters, which control the learning process of the algorithms. Then, the results from each machine learning model are compared using several statistical metrics such as R-2, mean squared error (MSE), mean absolute error (MAE), maximum error, and median error. The XGBoost regression model is the most effective among the tested algorithms. It is observed that the five tested algorithms can be ranked as XG boost > gradient boost > AdaBoost > random forest > linear regression.
更多查看译文
关键词
machine learning, predictive models, regression, 3D printing, XGBoost
AI 理解论文
溯源树
样例
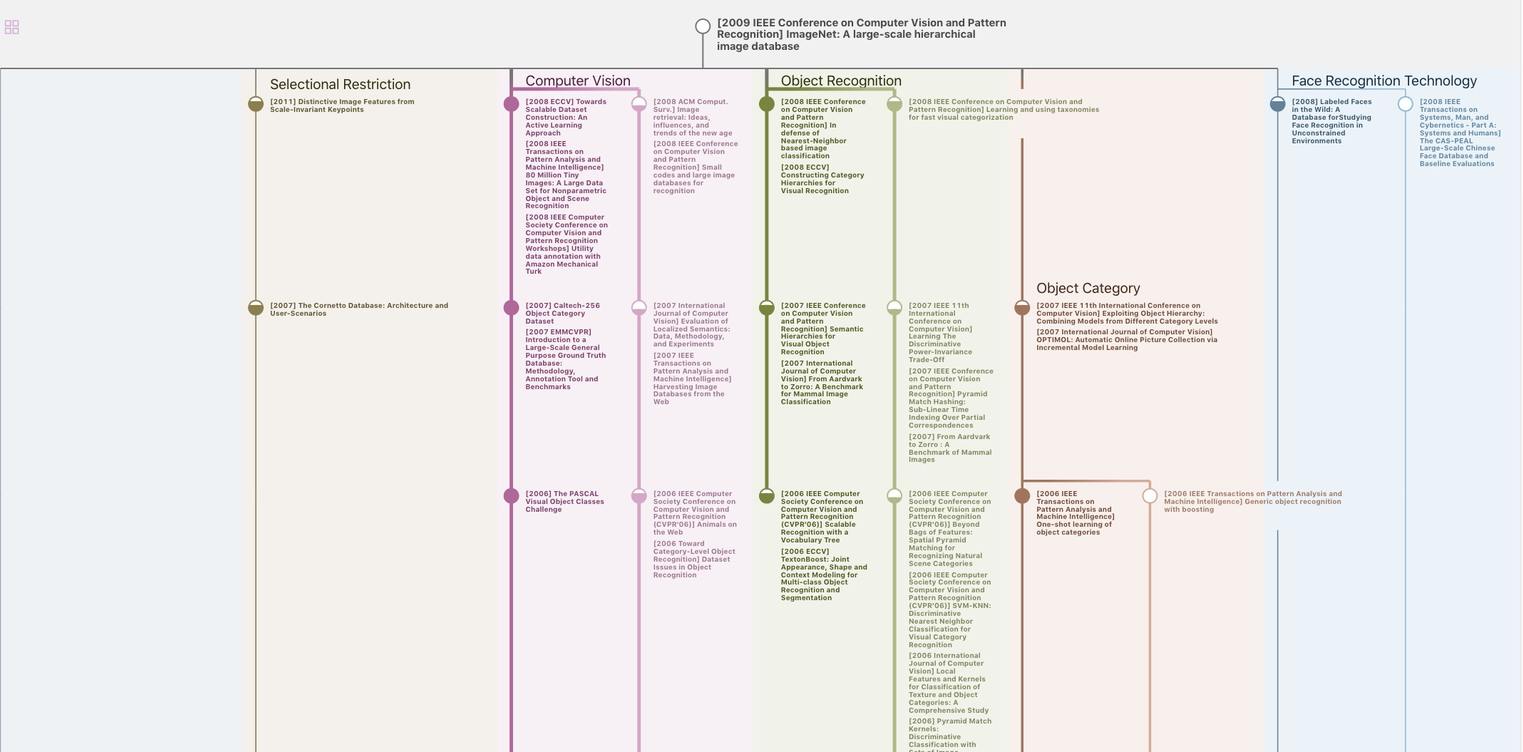
生成溯源树,研究论文发展脉络
Chat Paper
正在生成论文摘要