Silage Grass Sward Nitrogen Concentration and Dry Matter Yield Estimation Using Deep Regression and RGB Images Captured by UAV
AGRONOMY-BASEL(2022)
摘要
Agricultural grasslands are globally important for food production, biodiversity, and greenhouse gas mitigation. Effective strategies to monitor grass sward properties, such as dry matter yield (DMY) and nitrogen concentration, are crucial when aiming to improve the sustainable use of grasslands in the context of food production. UAV-borne spectral imaging and traditional machine learning methods have already shown the potential to estimate DMY and nitrogen concentration for the grass swards. In this study, convolutional neural networks (CNN) were trained using low-cost RGB images, captured from a UAV, and agricultural reference measurements collected in an experimental grass field in Finland. Four different deep regression network architectures and three different optimizers were assessed. The best average results of the cross-validation were achieved by the VGG16 architecture with optimizer Adadelta: r(2) of 0.79 for DMY and r(2) of 0.73 for nitrogen concentration. The results demonstrate that this is a promising and effective tool for practical applications since the sensor is low-cost and the computational processing is not time-consuming in comparison to more complex sensors.
更多查看译文
关键词
convolutional neural networks, grass swards, nitrogen concentration, dry matter yield, remote sensing, UAV, UAS
AI 理解论文
溯源树
样例
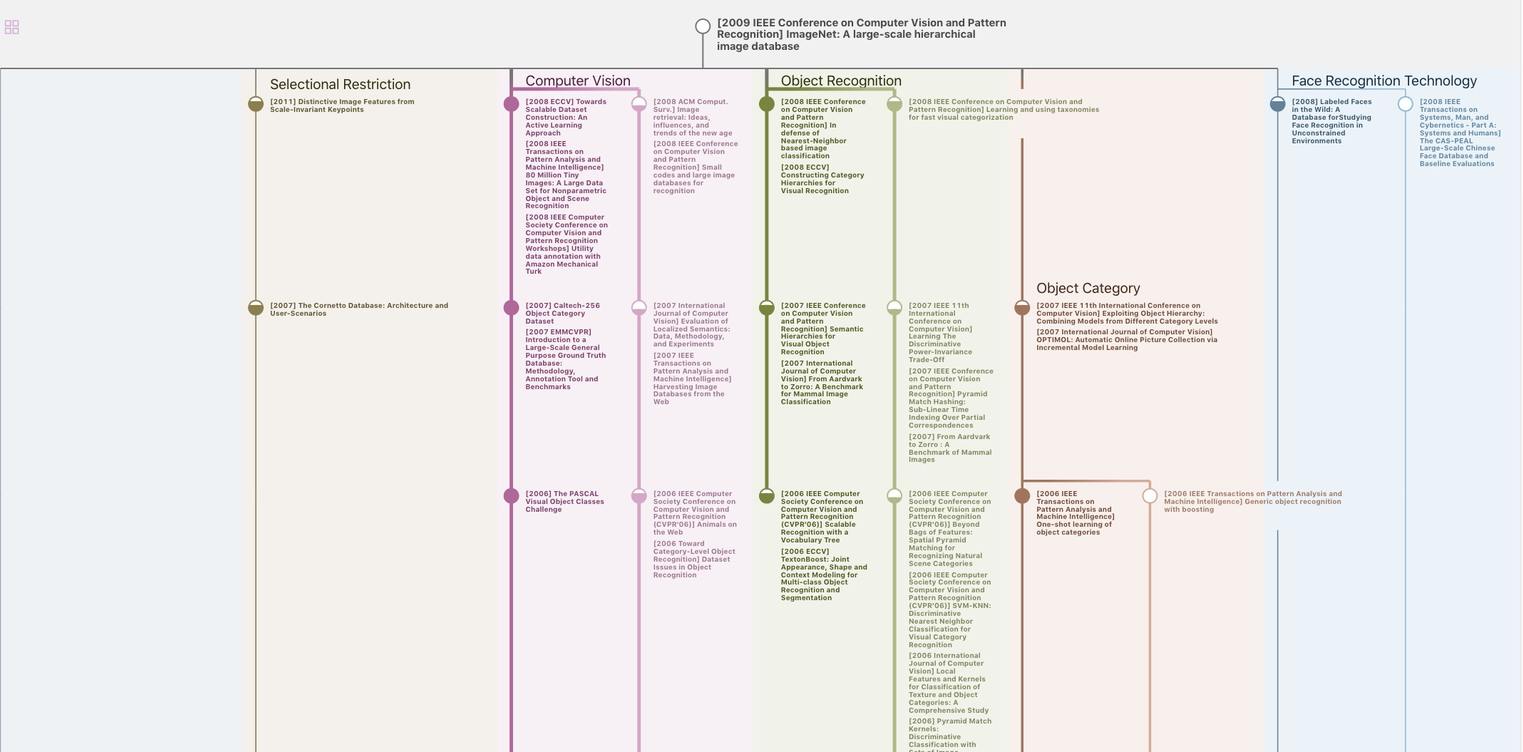
生成溯源树,研究论文发展脉络
Chat Paper
正在生成论文摘要