Lightweight spatial attentive network for vehicular visual odometry estimation in urban environments
NEURAL COMPUTING & APPLICATIONS(2022)
摘要
Visual odometry is the process of estimating the motion between two consecutive images. Traditional visual odometry algorithms require the careful fabrication of state-of-the-art building blocks based on geometry. These algorithms are highly sensitive to noise, and performance degradation of a single subprocess compromises the performance of the entire system. On the other hand, learning-based methods automatically learn the features required through motion mapping. However, current learning-based methods are computationally expensive and require a significant amount of time to estimate the pose from a video sequence. This method proposes a lightweight deep neural networks architecture to estimate the odometry by exploiting the refined features through spatial attention. Three different training and test splits of the KITTI benchmark are used to effectively evaluate the proposed approach. The execution time of the proposed approach is ∼ 1 ms, speeded up by 47 times over [ 1 ]. Performed experiments demonstrate the promising performance of the proposed method to the methods used in the comparison.
更多查看译文
关键词
Visual odometry,Pose estimation,Spatial attention,Convolutional neural networks,Homoscedastic uncertainty
AI 理解论文
溯源树
样例
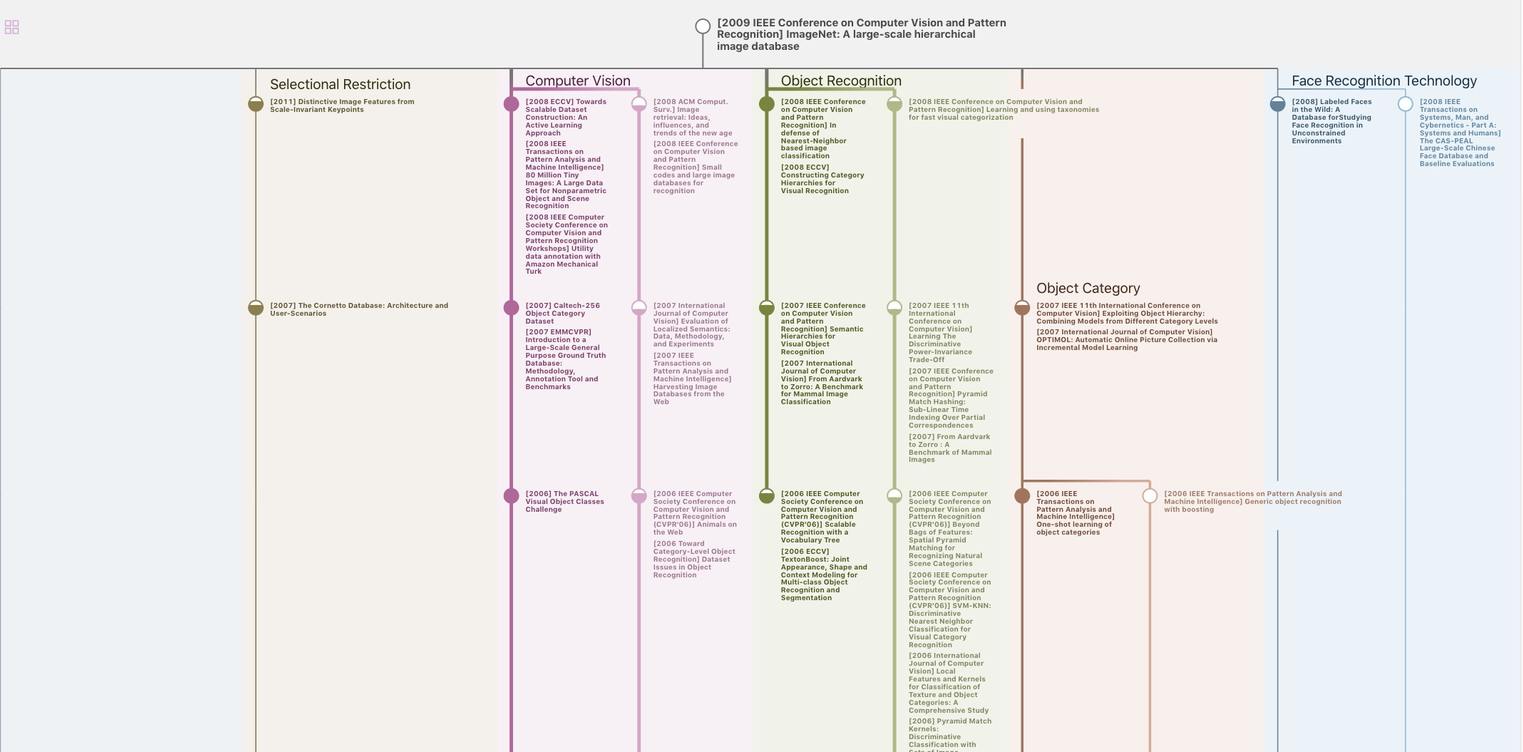
生成溯源树,研究论文发展脉络
Chat Paper
正在生成论文摘要