Attentional Gated Res2Net for Multivariate Time Series Classification
NEURAL PROCESSING LETTERS(2022)
摘要
Multivariate time series classification is a critical problem in data mining with broad applications. It requires harnessing the inter-relationship of multiple variables and various ranges of temporal dependencies to assign the correct classification label of the time series. Multivariate time series may come from a wide range of sources and be used in various scenarios, bringing the classifier challenge of temporal representation learning. We propose a novel convolutional neural network architecture called Attentional Gated Res2Net for multivariate time series classification. Our model uses hierarchical residual-like connections to achieve multi-scale receptive fields and capture multi-granular temporal information. The gating mechanism enables the model to consider the relations between the feature maps extracted by receptive fields of multiple sizes for information fusion. Further, we propose two types of attention modules, channel-wise attention and block-wise attention, to better leverage the multi-granular temporal patterns. Our experimental results on 14 benchmark multivariate time-series datasets show that our model outperforms several baselines and state-of-the-art methods by a large margin. Our model outperforms the SOTA by a large margin, the classification accuracy of our model is 10.16% better than the SOTA model. Besides, we demonstrate that our model improves the performance of existing models when used as a plugin. Further, based on our experiments and analysis, we provide practical advice on applying our model to a new problem.
更多查看译文
关键词
Multivariate time series classification,Convolutional neural network,Attention module,Gating mechanism
AI 理解论文
溯源树
样例
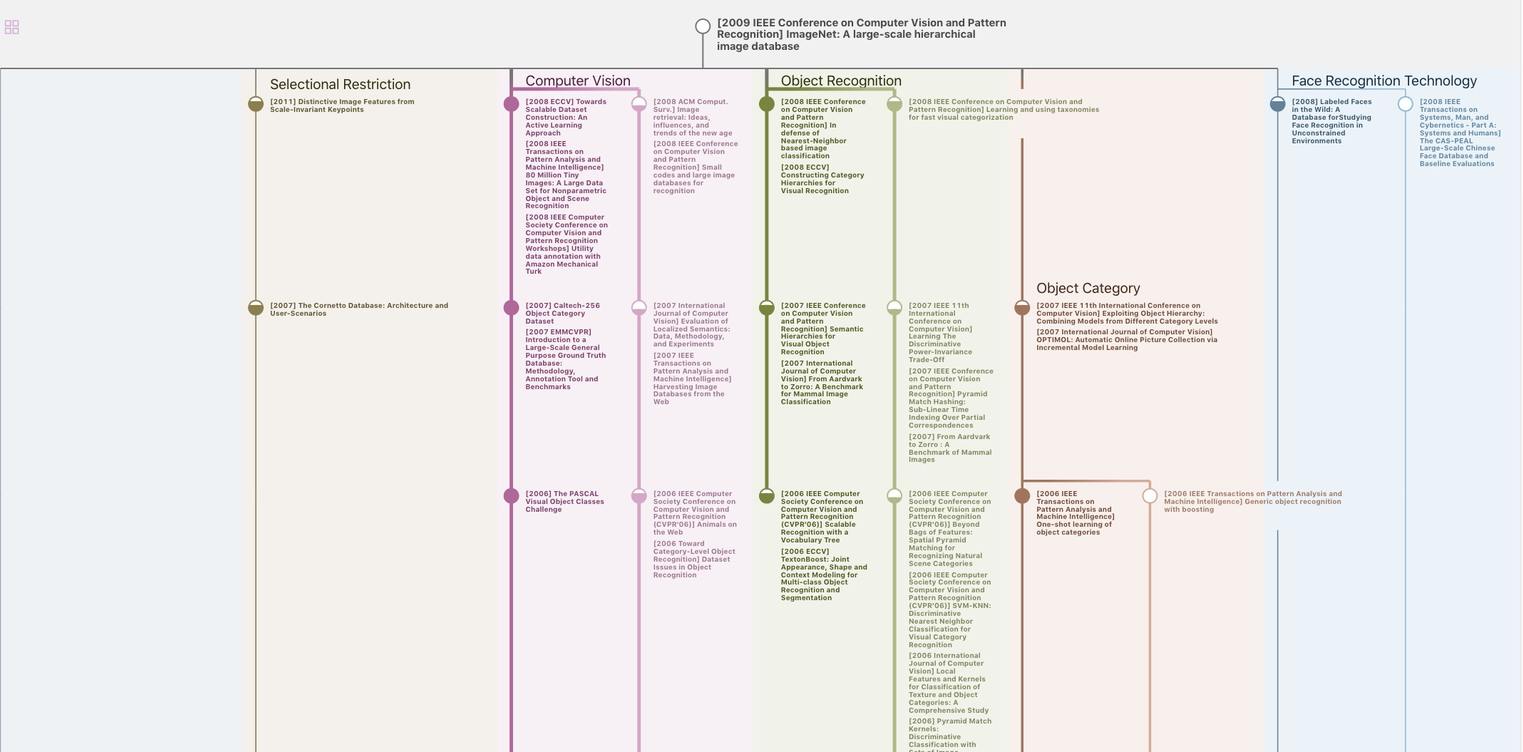
生成溯源树,研究论文发展脉络
Chat Paper
正在生成论文摘要