In situ microstructure property prediction by modeling molten pool-quality relations for wire-feed laser additive manufacturing
Journal of Manufacturing Processes(2022)
摘要
Metal additive manufacturing (AM) is sensitive to changes in process or environment variables that potentially significantly impact the part quality. The lack of in-process microstructure monitoring and control for quality assurance of AM parts is the main barrier that prevents the widespread use of AM technologies. To enable in situ quality monitoring of printed part characteristics, we have developed an in-process sensing system integrated with a wire-feed laser AM (WLAM) machine to monitor the molten pool dimensional and temperature profiles, which are a significant indicator of microstructural properties of final parts. The part microstructure characteristics such as Alpha lath thickness and Beta grain size are evaluated comprehensively. A convolutional neural network (CNN) model is developed for establishing the correlations directly from in-process molten pool information to the microstructural properties. The multi-modality CNN (m-CNN) receives both camera and pyrometer sensors as input to improve the property prediction performance. The m-CNN model is investigated, validated, and benchmarked with the traditional Gaussian process regression (GPR) model. The developed model proves the feasibility of in-process microstructural property estimation from molten pool sensing data to provide a pathway for high quality design and control of metallic AM technologies.
更多查看译文
关键词
Wire-feed laser additive manufacturing (WLAM),Convolutional neural network (CNN),Molten pool,Microstructure,Quality control
AI 理解论文
溯源树
样例
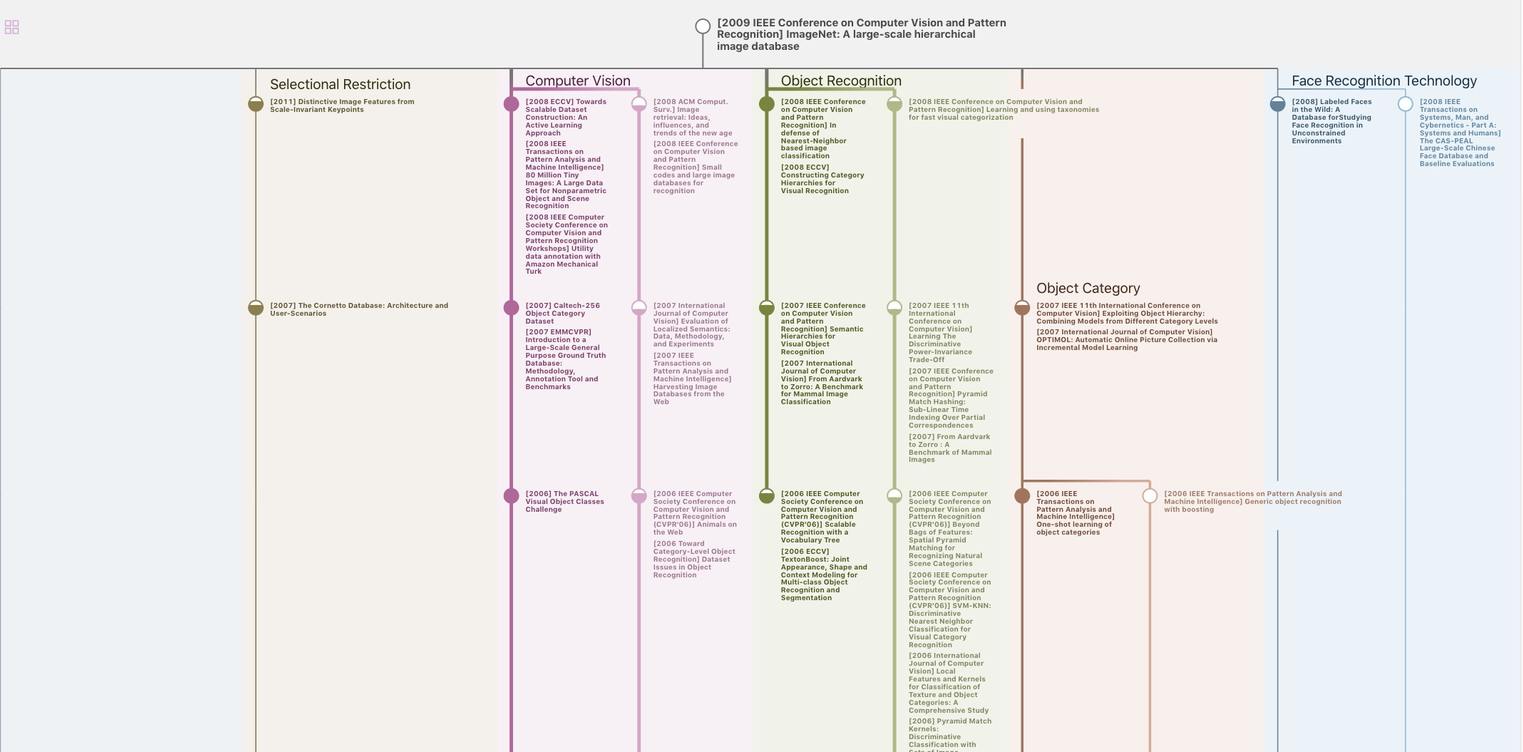
生成溯源树,研究论文发展脉络
Chat Paper
正在生成论文摘要