Cross-Modal Knowledge Distillation for Depth Privileged Monocular Visual Odometry
IEEE Robotics and Automation Letters(2022)
摘要
Most self-supervised monocular visual odometry (VO) suffer from the scale ambiguity problem. A promising way to address this problem is to introduce additional information for training. In this work, we propose a new depth privileged framework to learn a monocular VO. It assumes that sparse depth is provided during training time but not available at the test stage. To make full use of the privileged depth information, we propose a cross-modal knowledge distillation method, which utilizes a well-trained visual-lidar odometry (VLO) as a teacher to guide the training of the VO network. Knowledge distillation is conducted at both output and hint levels. Besides, a distillation condition check is also designed to leave out the noise that may be contained in the teacher’s predictions. Experiments on the KITTI odometry benchmark show that the proposed method produces accurate pose estimation results with a recovered actual scale. It also outperforms most stereo privileged monocular VOs.
更多查看译文
关键词
Deep learning methods,knowledge distillation,localization,visual odometry
AI 理解论文
溯源树
样例
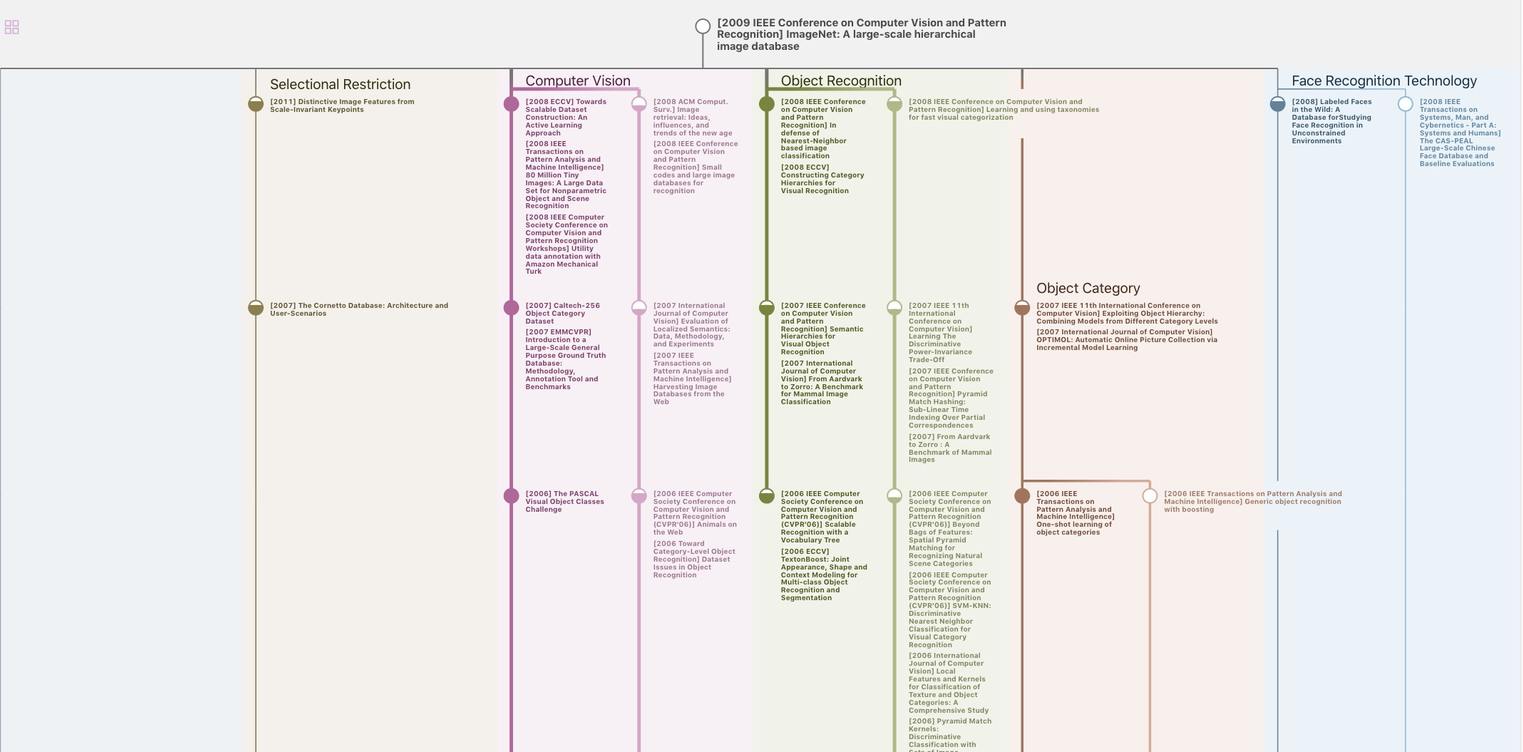
生成溯源树,研究论文发展脉络
Chat Paper
正在生成论文摘要