Self-Supervised Multi-Label Transformation Prediction for Video Representation Learning
JOURNAL OF CIRCUITS SYSTEMS AND COMPUTERS(2022)
摘要
Self-supervised learning is a promising paradigm to address the problem of manual-annotation through effectively leveraging unlabeled videos. By solving self-supervised pretext tasks, powerful video representations can be discovered automatically. However, recent pretext tasks for videos rely on utilizing the temporal properties of videos, ignoring the crucial supervisory signals from the spatial subspace of videos. Therefore, we present a new self-supervised pretext task called Multi-Label Transformation Prediction (MLTP) to sufficiently utilize the spatiotemporal information in videos. In MLTP, all videos are jointly transformed by a set of geometric and color-space transformations, such as rotation, cropping, and color-channel split. We formulate the pretext as a multi-label prediction task. The 3D-CNN is trained to predict a composition of underlying transformations as multiple outputs. Thereby, transformation invariant video features can be learned in a self-supervised manner. Experimental results verify that 3D-CNNs pre-trained using MLTP yield video representations with improved generalization performance for action recognition downstream tasks on UCF101 (+2.4%) and HMDB51 (+7.8%) datasets.
更多查看译文
关键词
Action recognition, multi-label transformation, self-supervised learning, video representation
AI 理解论文
溯源树
样例
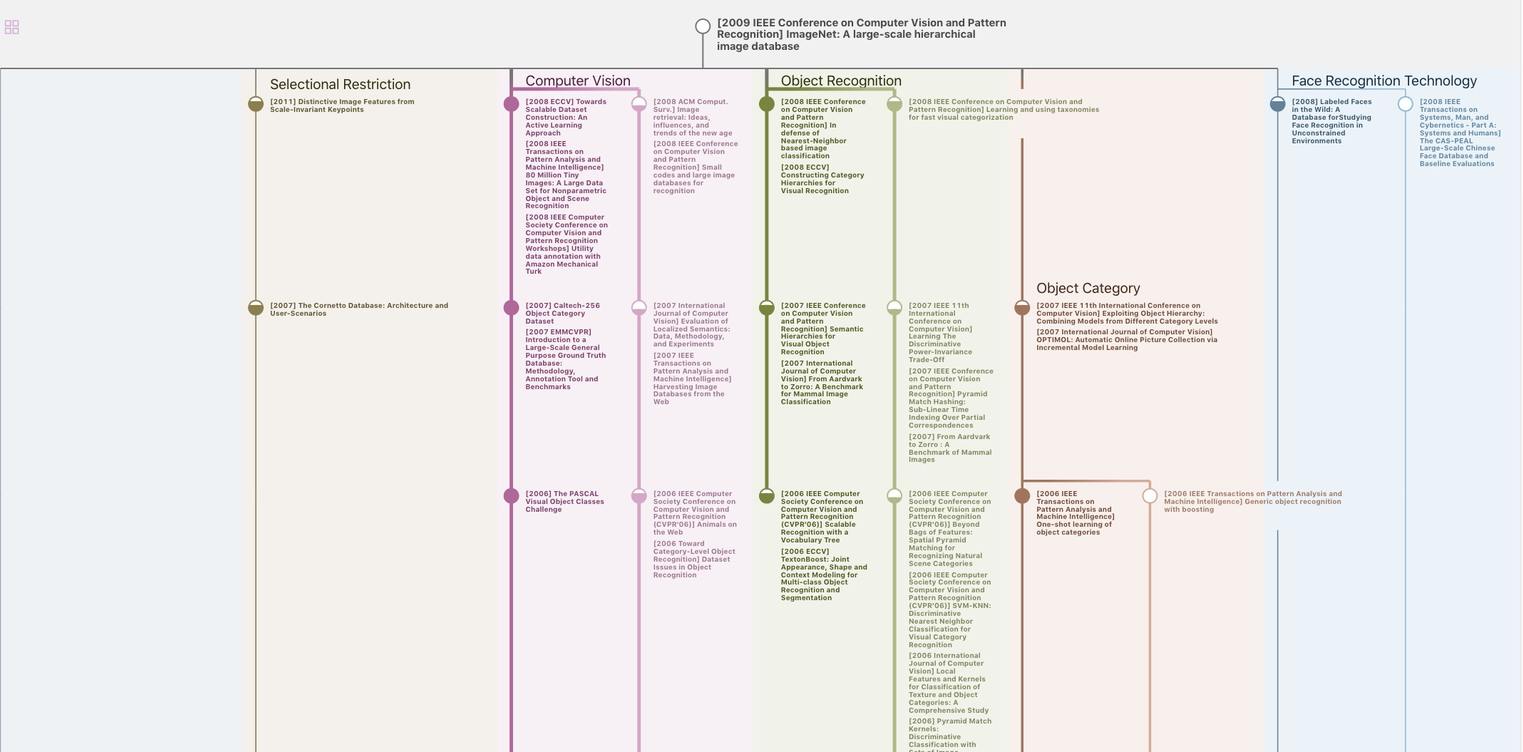
生成溯源树,研究论文发展脉络
Chat Paper
正在生成论文摘要